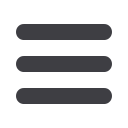

S854
ESTRO 36 2017
_______________________________________________________________________________________________
parameters. We approach the problem on a mechanistic
level, linking nanoscale energy deposition to cellular
repair.
Material and Methods
We present a stochastic model to predict ion induced DNA
damage and subsequent repair. DNA damage patterns are
predicted using nanodosimetric principles applied to track
structure simulations within the Monte Carlo based
Geant4-DNA toolkit. A section of detailed DNA geometry is
irradiated to study specific DNA double strand break
structures; building up a library of break models for a
given radiation quality. These patterns are then fed into a
modified Geant4-DNA simulation where the DNA double
strand break ends are explicitly modelled within a
simplified cell nucleus. Double strand break ends then
progress along the predefined Non-Homologous End
Joining repair pathway according to stochastic, time
constant based state changes. This allows the prediction
of differences in DNA repair for a range of radiation
qualities.
Results
We show that break complexity and repair kinetics are
dependent on the particle LET and particle type, with
more complex breaks becoming more probable for higher
LET (fig 1.). Our simulations predict a greater number of
residual DSBs after 24h when higher LET particles are used
(fig 2.), which is in good agreement with the literature.
We also observe a difference in break complexity for
protons and alpha particles at the same LET due to
differences in radiation track structure.
Conclusion
Monte Carlo track structure simulation coupled to a
mechanistic DNA damage repair simulation is a useful tool
for modelling biologically relevant endpoints to cellular
radiation injury. We have modelled DSB damage and repair
with respect to several beam delivery parameters. The
complexity of the biological response caused by different
ions of the same LET was found to differ due to the
radiation track structure. We suggest that this is as a
direct consequence of the complexity of the breaks
caused, as similar trends are observed for both repair and
break induction. This is of relevance for potential
application to LET based treatment plans.
EP-1605 Deep learning of radiomics features for
survival prediction in NSCLC and Head and Neck
carcinoma
A. Jochems
1
, F. Hoebers
1
, D. De Ruysscher
1
, R.
Leijenaar
1
, S. Walsh
1
, B. O'Sullivan
2
, J. Bussink
3
, R.
Monshouwer
3
, R. Leemans
4
, P. Lambin
1
1
MAASTRO Clinic, Radiotherapy, Maastricht, The
Netherlands
2
Princess Margaret Cancer Centre, Cancer Clinical
Research Unit, Toronto, Canada
3
Radboud University Medical Center Nijmegen, Radiation
Oncology, Nijmegen, The Netherlands
4
VU University Medical Center, Department of
Otolaryngology/Head and Neck Surgery, Amsterdam, The
Netherlands
Purpose or Objective
In order to facilitate personalized medicine in cancer
care, predictive models are of vital importance.
Radiomics, the high-throughput extraction of large
amounts of image features from radiographic images,
facilitates predictive model development by providing
non-invasive biomarkers. Previous work indicates that
radiomics features have high predictive quality
1
. However,
these studies used conventional models and the added
value of deep learning combined with radiomics features
is unexplored. Furthermore, conventional modelling
strategies require a selection of features to establish a
signature whereas deep learning algorithms do not. In this
work we learn a deep learning model on radiomics
features and compare it to a previously published cox
regression model
1
.
Material and Methods
4 independent Lung and Head & Neck (H&N) cancer
cohorts (1418 total patients) were used in this study.
Radiomic features were extracted from the pre-treatment
computed tomography images. The model was learned on
the Institute 1 lung cohort (N=422) and validated on the
other datasets. The outcome is two-year survival following
treatment. A 3 layer deep learning network was used to
make predictions.
Results
Validation on Institute 2 dataset (N=154) yields an AUC of
0.71 (95% CI: 0.63-0.8) for the deep learning network and
0.66 on the conventional model (95% CI: 0.56-0.75). The
difference is not significant (P=0.11). Validation on
Institute 3 dataset (N=95) yields an AUC of 0.64 (95% CI:
0.53-0.79) for the deep learning network and 0.75 on the
conventional model (95% CI: 0.64-0.86). The difference is
not significant (P=0.19). Validation on Institute 4 dataset
(N=136) yields an AUC of 0.71 (95% CI: 0.59-0.8) for the
deep learning network and 0.74 on the conventional model
(95% CI: 0.64-0.83). The difference is not significant (P =
0.24). Validation on Institute 5 dataset (N=540) yields an
AUC of 0.58 (95% CI: 0.52-0.63) for the deep learning
network and 0.65 on the conventional model (95% CI: 0.59-
0.70). The difference is not significant (P = 0.10).