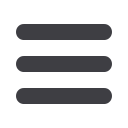

S851
ESTRO 36 2017
_______________________________________________________________________________________________
presence of immunosuppressive cells in the tumor
microenvironment and tumor infiltrating lymphocytes, the
regulation of immunogenic cell surface receptors, and
immunogenic cell death. However, the balance between
pro-tumor and anti-tumor effects is delicate, and the
application of immunotherapy in combination with
radiotherapy has to be designed very carefully in order to
tip the immunomodulatory effect of radiation in the right
direction. There are many parameters that can be varied
in this equation, including time, dose and fractionation.
Therefore, in order to better understand the
immunomodulatory effect of radiation, and to be able to
optimize the combined treatment, there is a great need
for mathematical models.
Material and Methods
In this work, a mathematical model based on the work by
Serre et al.
1
was developed to describe the synergistic
effect of immunotherapy and radiotherapy observed in a
previous pre-clinical study in glioma carrying rats.
2
Animals with intracranial tumors were given indoleamine
2,3-dioxygenase (IDO) inhibitory treatments with
intraperitoneal injections of 1-methyl tryptophan (1-MT),
in combination with radiotherapy given as single fractions
of 8 Gy.
1
Serre R, et al. Mathematical model of cancer
immunotherapy and its synergy with radiotherapy. Cancer
Res
76(17):4931–40, 2016.
2
Ahlstedt J, et al. Effect of Blockade of Indoleamine 2, 3-
dioxygenase in Conjunction with Single Fraction
Irradiation in Rat Glioma. J J Rad Oncol 2(3):022, 2015.
Results
Using the mathematical model tumor growth and survival
curves were simulated, and the parameters of the model
were fit to the experimental data. Good agreement for
median survival time was achieved both for the two
modalities given separately as monotherapies, as well as
for
the
combined
treatment,
see
Figure.
Conclusion
Conclusion: The simplified mathematical model presented
in this work captures the general features of the
synergistic combination of IDO-inhibitory immunotherapy
and single fraction radiotherapy. The model can be used
to explore possible alternative time, dose and
fractionation, in order to gain improved insight into the
effects of these parameters, and to generate plausible
hypotheses for further pre-clinical studies.
EP-1600 Delta radiomics of NSCLC using weekly cone-
beam CT imaging: a feasibility study
J. Van Timmeren
1
, R. Leijenaar
1
, W. Van Elmpt
1
, S.
Walsh
1
, A. Jochems
1
, P. Lambin
1
1
Department of Radiation Oncology - MAASTRO, GROW
School for Oncology and Developmental Biology -
Maastricht University Medical Centre MUMC, Maastricht,
The Netherlands
Purpose or Objective
Currently, prognostic information is commonly derived
using radiomics features from medical images acquired
prior to treatment. However, the potential of delta
radiomics, i.e. the change of radiomic features over time,
has not yet been extensively explored. Cone-beam CT
(CBCT) imaging can be performed daily for lung cancer
patients and is therefore a potential candidate for delta
radiomics, which may allow further treatment
individualization. In this study we explored delta
radiomics using CBCT imaging by investigating the number
of features changing at a specific time point during
treatment. Moreover, we investigated the differences
between patients having an overall survival of less or more
than 2 years.
Material and Methods
A total of 40 stage II-IV NSCLC patients, receiving
curatively intended radiotherapy for a period of at least
six weeks, were included in the study. The CBCT images
used in this study were 1) CBCT prior to the first fraction
of treatment (CBCTfx1), 2) CBCT prior the second fraction
of treatment (CBCTfx2), 3) CBCT one week after the start
of treatment (CBCTweek2), 4) CBCT three weeks after the
start of treatment (CBCTweek4) and 5) CBCT five weeks
after the start of treatment (CBCTweek6). For 38 patients
CBCTfx1 and CBCTfx2 were available, whereas for 33
patients all weekly CBCTs were available. All patients had
a minimal follow-up of 2 years. Per time point, a total of
1046 radiomic features were derived from the primary
tumor volume. The images prior to the first and second
fraction were used to calculate the variability in imaging
features using the coefficient of repeatability (COR),
defined as 1.96*SD. The weekly images were used to
investigate the number of features changing more than
the COR with respect to baseline (CBCTfx1).
Results
Figure 1 represents the total number of features that
changed more than the COR, ranging from 0 to 999
features. The median number of features that changed for
the group with overall survival <2 years was 279, whereas
this was 500 for the group with overall survival >2 years
(Mann-Whitney U test, p = 0.06). For 8 out of 10 patients
that survived >2 years, more features (31.7%) changed one
week after CBCTfx1 than for 13 out of 23 patients that did
not survive two years.
Conclusion
This study shows that a large proportion of the radiomic
features derived from cone-beam CT images change
significantly during the course of treatment, meaning that
an interval of about two weeks is feasible for a radiomics
study using CBCT imaging. The larger number of features
that changed in the group with overall survival >2 years
could reflect an early response of the tumor to the
treatment. In future research, the prognostic value of
changing radiomic features (delta radiomics) should be
explored in a larger cohort.
EP-1601 Do higher CT pixel values outside the GTV
predict for poorer lung cancer survival?
M. Van Herk
1
, J. Kennedy
2
, E. Vasquez Osorio
1
, C. Faivre-
Finn
1
, A. McWilliam
1
1
University of Manchester, Division of Molecular and
Clinical Cancer Sciences- Faculty of Biology- Medicine
and Health, Manchester, United Kingdom
2
The Christie NHS Foundation Trust, Department of
Infomatics, Mancehster, United Kingdom