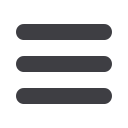

INFORMS Philadelphia – 2015
100
3 - Optimal Online Selection of a Monotone Subsequence:
A Central Limit Theorem
Vinh V. Nguyen, PhD Student, The Fuqua School of Business,
Duke University, 100 Fuqua Drive, Durham, NC, 27708,
United States of America,
vn26@duke.edu, Alessandro Arlotto,
J. Michael Steele
Consider a sequence of n independent random variables with common
continuous distribution and consider the task of choosing an increasing
subsequence where the observations are revealed sequentially and must be
accepted or rejected when they are first revealed. There is a unique selection
policy that maximizes the expected number of selected observations. We prove a
central limit theorem for this number of optimally selected observations and
characterize its mean and variance for large n.
4 - A Bayesian Decision-theoretic Model of Sequential Clinical Trials
with Delayed Responses
Stephen Chick, Professor, INSEAD, Technology Management
Area, Boulevard de Constance, Fontainebleau, 77300, France,
stephen.chick@insead.edu, Martin Forster, Paolo Pertile
Clinical trials are necessary for evaluating the benefit of health technologies but
are quite costly, but most analysis do not account for the effect of delayed
observations on optimal trial design. We take a Bayesian sequential learning
approach to the economics of clinical trial design with dynamic programming. We
provide structural results and apply optimal stopping time solutions to data from
recent clinical trials.
SC23
23-Franklin 13, Marriott
Solutions for Large Markov Chains
Sponsor: Applied Probability
Sponsored Session
Chair: Mor Harchol-Balter, Professor, Carnegie Mellon University,
Computer Science Dept., 5000 Forbes Ave., Pittsburgh, PA,
United States of America,
harchol@cs.cmu.edu1 - Clearing Analysis on Phases: Distribution of Skip-free,
Unidirectional, Quasi-birth-death Processes
Sherwin Doroudi, PhD Candidate, Carnegie Mellon University,
sdoroudi@andrew.cmu.edu, Mor Harchol-Balter, Brian Fralix
A wide variety of problems in manufacturing, service, and computer systems can
be modeled by infinite repeating quasi-birth-death process (QBD) Markov chains
with infinitely many levels and finitely many phases. Many such chains have
transitions that are skip-free in level and unidirectional in phase. We present the
Clearing Analysis on Phases (CAP) method, which yields the exact limiting
probabilities of the states in such chains as a linear combinations of scalar bases
raised to powers.
2 - M/M/C Queue with Two Priority Classes
Opher Baron, University of Toronto, 105 St. George St, Toronto,
ON, Canada,
opher.baron@rotman.utoronto.ca, Jianfu Wang,
Alan Scheller-wolf
We provide the first exact analysis of a preemptive M/M/C queue with two
priority classes with different service rates. We introduce a technique to reduce
the 2-dimensionally Infinite Markov Chain (IMC), representing this problem, into
a 1-dimensionally IMC. We derive the law for the number of low-priority jobs.
Numerical examples demonstrate the accuracy of our algorithm and generate
new insights. We demonstrate how our methodology solves other problems.
3 - Product-Form Solutions for a Class of Structured Multi-
Dimensional Markov Processes
Jori Selen, PhD Candidate, Eindhoven University of Technology,
De Zaale, Eindhoven, Netherlands,
j.selen@tue.nl,Johan Van Leeuwaarden, Ivo Adan
Motivated by queueing systems with heterogeneous parallel servers, we consider
a class of structured multi-dimensional Markov processes whose state space can
be partitioned into two parts: a finite set of boundary states and a structured
multi-dimensional set of states, exactly one dimension of which is infinite. Using
separation of variables, we show that the equilibrium distribution, typically of the
queue length, can be represented as a linear combination of product forms.
SC24
24-Room 401, Marriott
Fashion, Innovation and Technology
Sponsor: Artificial Intelligence
Sponsored Session
Chair: Heng Xu, Associate Professor, Pennsylvania State University,
316H IST Building, University Park, PA, 16802,
United States of America,
hxu@ist.psu.edu1 - Fashion-Telling: Predicting the Future of Digital Innovations
Ping Wang, Associate Professor, University of Maryland, College
Park, 4105 Hornbake Bldg., College of Information Studies,
College Park, MD, 20742, United States of America,
pwang@umd.eduThe fusion of digital technologies and fashion makes innovation trajectories
notoriously fickle and hard to predict. This study combines organizational ecology
and network theories to conceptualize digital innovation ecosystems and proposes
to use the ecology and structure of these ecosystems to predict the trajectories of
digital technologies. The utility of this approach is illustrated in a case study, with
implications to Information Systems strategy and management articulated.
2 - Making Sense of Materiality: The Case of 3d Printed Fashion
Ning Su, Assistant Professor, Ivey Business School, Western
University, 1255 Western Road, London, ON, N6G 0N1, Canada,
nsu@ivey.uwo.ca3D printing is increasingly embraced by fashion designers. Due to the nascent and
evolving nature of 3D printing, however, there is significant uncertainty around
its affordances and constraints. Drawing on qualitative data, this exploratory
study shows that fashion designers seek to make sense of this emerging
technology by pursuing a collective design process with multiple stakeholders.
The ongoing interaction among diverse actors gives rise to innovative perceptions,
practices, and products.
3 - Fashion and Text Analytics
Heng Xu, Associate Professor, Pennsylvania State University,
316H IST Building, University Park, PA, 16802,
United States of America,
hxu@ist.psu.edu,Yilu Zhou
The Fashion Industry is extremely competitive in terms of adapting to fast
evolving fashion trends and consumer demand. Building upon various fashion
theories, we empirically examine fashion designer’s evolution by studying a
variety of data including fashion articles, magazines, blogs, and social media. By
using text analytics, we envision the color, print, and style for upcoming trends
and help retailers to stay on top of the latest fashion.
4 - Mining Social Media and Press Release for Competitive
Intelligence: A Case Study on IBM
Yuan Xue, The George Washington University, 2201 G Street,
NW, Suite 515, Washington, DC, United States of America,
xueyuan@gwmail.gwu.edu, Subhasish Dasgupta, Yilu Zhou
Competitive intelligence (CI) refers to the study of competitors and competitive
environment in support of decision-making. CI is usually generated by CI
professionals. The recent CI trend is to collect competitor information online. We
believe that social media analysis can help us to identify both direct and indirect
competitors, rank competitors based on their market commonality and learn the
strength and weakness of focal company.
SC25
25-Room 402, Marriott
E-Commerce and Digital Marketing
Sponsor: Information Systems
Sponsored Session
Chair: Dokyun Lee, Carnegie Mellon University,
United States of America,
leedokyun@gmail.com1 - Measuring Display Advertising Effects on Online Search Behavior
Vilma Todri, PhD Candidate In Information Systems, NYU, 44 W
4th St, KMC Room 8-181, New York, Ne, 10012, United States of
America,
vtodri@stern.nyu.edu, Anindya Ghose
The increasing availability of individual-level data has raised the standards for
measurability and accountability in online advertising. Using a novel data set with
granular measurements of advertising exposures, we examine a wide range of
consumer behaviors and capture the effectiveness of display advertising across
channels as well as the dynamics of these effects across the purchasing funnel
path of consumers. We discover rich findings with important managerial
implications.
SC23