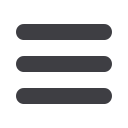

INFORMS Philadelphia – 2015
103
2 - A Statistical Learning Approach to Personalization in
Revenue Management
Zachary Owen, Massachusetts Institute of Technology, 77
Massachusetts Ave, E40-149, Cambridge, MA, 02139, United
States of America,
zowen@mit.edu,David Simchi-levi, Xi Chen,
Clark Pixton
We develop a framework for modeling personalized decision problems which
gives a data driven algorithm for revenue maximization using contextual
information. We apply our method to pricing and assortment optimization. We
prove a high-probability bound on the gap between the revenue of the estimated
policy and the revenue generated under full knowledge of the demand
distribution. We demonstrate the performance of our method on both airline
seating data and simulated data.
3 - Uncovering Hidden Decision Processes through Integration of
Independent Databases
Nooshin Valibeig, Northeastern University,
334 Snell Engineering, Boston, MA, 02115, United States of
America,
nooshin.valibeig@gmail.com, Jacqueline Griffin
Data is a key driver in analyzing and evaluating the effectiveness of decision
processes. We develop an algorithm to uncover hidden information about
decision processes in resource allocation systems. Specifically, the algorithm joins
event-oriented and snapshot-in-time databases to extract new knowledge about
decision processes. The precision and robustness of the algorithm is quantified
with simulated data. A case study with hospital patient flow data is presented.
4 - Reinforcement Learning Algorithms for Regret Minimization in
Structured Markov Decision Processes
Theja Tulabandhula, Xerox Research Centre India, Bangalore,
Bangalore, India,
theja2t@gmail.com, Prabuchandran K. J.,
Tejas Bodas
For several RL problems the optimal policy of the underlying Markov Decision
Process (MDP) is characterized by a known structure. We develop new RL
algorithms that exploit the structure of the optimal policy to minimize regret.
Numerical experiments on MDPs with structured optimal policies show that our
algorithms have better performance than current state of the art, are easy to
implement, have a smaller run-time, can be parallelized and require less number
of random number generations.
SC32
32-Room 409, Marriott
Special Topics in Supervised Learning: Variable
Selections and Dimension Reductions
Sponsor: Data Mining
Sponsored Session
Chair: Chaojiang Wu, Drexel University, 727 Gerri LeBow Hall, 3220
Market Street, Philadelphia, PA, 19104, United States of America,
cw578@drexel.edu1 - Sparse Nonlinear Feature Selection by Locally
Discriminative Constraints
Chuanren Liu, Assistant Professor, Drexel University, 3220
Market St, Philadelphia, PA, 19104, United States of America,
liuchuanren@gmail.com,Kai Zhang
We present an approach to sparse nonlinear feature selection for K-nearest
neighbor (KNN) classification. First, the factors for selecting feature are optimized
with locally discriminative constraints, which encourage smaller distances
between neighbors from the same class and larger distances between neighbors
from different classes. Then, we use lasso to achieve the sparse feature selection.
We also show an interesting connection between our formulation and the support
vector machines (SVMs).
2 - Maximum Tangent Likelihood Estimation and Robust
Variable Selection
Yichen Qin, Assistant Professor, University of Cincinnati, 2925
Campus Green Dr., Cincinnati, OH, 45221, United States of
America,
yichenqin@gmail.com, Yan Yu, Yang Li, Shaobo Li
In this article, we propose a new class of likelihood function, called Tangent
Likelihood function, that can be used to obtain robust estimates, termed as
Maximum Tangent Likelihood Estimator (MtLE). We show that the MtLE is root-
n consistent and asymptotically normally distributed. Furthermore, we consider
robust variable selection based on our proposed tangent likelihood function. The
proposed MtLE-Lasso can perform robust estimation and variable selection
simultaneously and consistently.
3 - Pseudo Sufficient Dimension Reduction
Wenbo Wu, University of Oregon, Lundquist College of Business,
Eugene, OR, 97403, United States of America,
wuwenbouga@gmail.comWe propose a new concept of pseudo sufficient dimension reduction based on an
underlying relationship between ridge regression and measurement error
regression. With such a connection, we propose a general sufficient dimension
reduction estimation procedure to obtain an estimate from a different subspace
instead of the targeted population parameter space. Variable selection based on
pseudo estimate works effectively for both highly correlated predictors and for the
small n large p problem.
4 - Structured Multitask Feature Selection
Fei Wang, Associate Professor, University of Connecticut,
371 Fairfield Way, Unit 4155, Storrs, CT, 06269,
United States of America,
fei_wang@uconn.eduIdentification of important features for specific tasks is an important problem in
modern data analytics. In this talk, I will focus on the multitask feature selection
problem, where multiple related tasks are considered simultaneously and the
important features for each task is selected. I propose a structured optimization
approach, where similar tasks share similar important feature set. I applied the
proposed approach for risk factor identification in Comprehensive Geriatric
Assessment.
SC33
33-Room 410, Marriott
Statistics and Optimization Methods for Pain
Management
Sponsor: Health Applications
Sponsored Session
Chair: Jay Rosenberger, Associate Professor, University of Texas at
Arlington, Box 19017 UT, Arlington, TX, 76019,
United States of America,
jrosenbe@uta.edu1 - Iterative Data Imputation for Adaptive Pain Management
Yeqing Li, University of Texas Arlington, P.O. Box 19017,
Arlington, TX, 76019, United States of America,
yeqing.li@mavs.uta.edu,Junzhou Huang
Pain management is a major global health problem. Many efforts have been
devoted to developing data-driven decision models. However, the raw data is
usually subject to various levels of missing. The missing values in data limit the
quality and quantity of data and additionally limit the performance of the
decision models. To address this problem, we proposed an iterative data
imputation algorithm, which can accurately recover various kinds of missing
values.
2 - Inverse Probability of Treatment Weighting for Adaptive
Interdisciplinary Pain Management
Victoria Chen, The University of Texas at Arlington, Dept. of Ind.,
Manuf., & Sys. Engr., Campus Box 19017, Arlington, TX, 76019,
United States of America,
vchen@uta.edu, Li Zeng,
Aera Leboulluec, Robert Gatchel
We present a process based on the inverse probability of treatment weighting
method to address the endogeneity while estimating state transition and outcome
models for a two-stage adaptive interdisciplinary pain management program.
First, a method is developed for independent treatments then a general method is
developed for correlated treatments.
3 - Two-stage Feature Selection for Efficient Modeling of Pain
Management Data
Rohit Rawat, University of Texas Arlington, P.O. Box 19017,
Arlington, TX, 76019, United States of America,
rohit.rawat@mavs.uta.edu, Michael Manry
We use data from a pain management study in which treatment variables and
two pain intensity metrics were recorded in two treatment stages. Data sets for
the 235 subjects had 899 features for stage one and 1889 for stage two. A two-
stage feature selection algorithm was developed that fits a piecewise linear
network to the data, and removes useless inputs. We prevent overfitting through
the use of random probes and regularization. The method results in smaller
datasets and reduced testing error.
SC33