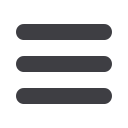

INFORMS Philadelphia – 2015
150
MA16
16-Franklin 6, Marriott
Conic Convex Optimization:
New Algorithms and Results
Sponsor: Optimization/Linear and Conic Optimization
Sponsored Session
Chair: Robert Freund, Professor, MIT Sloan School of Management,
Building E62-567, 77 Massachusetts Ave., Cambridge, MA, 02139,
United States of America,
rfreund@mit.edu1 - Approximation Schemes for Linear Programming in
Inner Product Spaces
Sergei Chubanov, University of Siegen, Kohlbettstr. 15, Siegen,
Germany,
sergei.chubanov@uni-siegen.deThe size of an LP is the sum of binary sizes of the coefficients describing this LP.
Such LPs are known to be polynomially solvable. The situation changes if each
element of the data can be accessed only via an oracle and the size is defined as
the size of the data used by the oracle. This class includes dynamic flows and DP
formulations of some other NP-hard problems. A further generalization leads to
LPs in inner product spaces. In this talk, we discuss a new algorithm for such
problems.
2 - Solving General Convex Conic Problems with First-order Methods
James Renegar, Professor, Cornell University, 224 Rhodes Hall,
Ithaca, NY, 14853, United States of America,
renegar@cornell.eduWe present recent results in ongoing research pertaining to a framework that is
novel in allowing any convex, conic optimization problem to be recast as an
equivalent convex optimization problem whose only constraints are linear
equations and whose objective function has Lipschitz constant no greater than
one, to which a broad class of first-order methods can be applied.
3 - New Computational Guarantees for First-order Methods for
Convex Optimization, via a Function Growth Constant
Robert Freund, Professor, MIT Sloan School of Management,
Building E62-567, 77 Massachusetts Ave., Cambridge, MA,
02139, United States of America,
rfreund@mit.edu,Haihao Lu
We present new algorithms and complexity bounds for solving convex
optimization problems using first-order methods. We presume we are given a
strict lower bound on f^*. We introduce a new functional measure called the
growth constant G for f(x) that measures how quickly the function level sets grow
and that plays a fundamental role in the complexity analysis. We present new
computational guarantees for non-smooth and smooth optimization that
improves on existing complexity bounds in many ways.
MA17
17-Franklin 7, Marriott
Network Optimization under Uncertainties
Sponsor: Optimization/Network Optimization
Sponsored Session
Chair: Neng Fan, University of Arizona, 1127 E. James E. Rogers Way
Room 111, Tucson, AZ, 85721, United States of America,
nfan@email.arizona.edu1 - Identifying Critical Nodes of Interdependent Networks by
Integer Programming
Shanshan Hou, University of Arizona, Tucson, AZ, 85721, United
States of America,
shanshanh@email.arizona.edu, Neng Fan,
Andres Garrido
In this talk, we analyze the vulnerability of interdependent networks by identify a
set of nodes in power grid, whose removal results high impacts by the cascading
failures in the interdependent communication network and itself. We propose an
approach by integer programming to identify such set of nodes. Knowing the
behavior of these networks can help to be more prepared before attacks and
failures that may affect the power network supply and functionality.
2 - Improving the Global Pre-positioning Network for Natural
Disaster Recovery
Adam Prokop, Graduate Msc Student In Supply Chain
Management, Wilfrid Laurier University, 75 University Avenue
West, Waterloo, ON, N2L3C5, Canada,
prok3910@mylaurier.caPre-positioning critical supplies in strategic locations can increase the effectiveness
of humanitarian relief aid for natural disasters. An optimization model, utilizing
recent global disaster risk indexes, was developed to evaluate the current United
Nations Humanitarian Response Depot network. Alterations of the current
network were shown to significantly minimize the average distance between pre-
positioning facilities and demand regions.
3 - Network Optimization under Non-linear Utility
Chin Hon Tan, National University of Singapore, 1 Engineering
Drive, Singapore, 117576, Singapore,
isetch@nus.edu.sgDecision makers are rarely risk neutral in practice. Hence, solutions that
maximize expected rewards or minimize expected costs, which assumes that the
decision maker is risk neutral, may not be appropriate. In this presentation, we
study the sensitivity of solutions with respect to the risk neutral assumption and
discuss how solutions that are robust to the decision maker’s utility can be
improved upon.
4 - An Integer Programming Approach for Mixed Fault Diameters
Elham Sadeghi, Graduate Research Assistant,
Univesity of Arizona, Tucson, AZ, United States of America,
sadeghi@email.arizona.edu, Neng Fan
We consider the minimum (k,l)-connected d-dominating set problem which is a
fault-tolerance dominating set. This problem is a generalization of minimum
connected dominating set problem. The integer programming formulations based
on vertex-cut and edge-cut is introduced and a cutting plane algorithm is
proposed to solve it.
MA18
18-Franklin 8, Marriott
The Reborn of Traditional OR Methods in the Era of
Big Data
Cluster: Modeling and Methodologies in Big Data
Invited Session
Chair: Shouyi Wang, Assistant Professor, University of Texas at
Arlington, 3105 Birch Ave, Grapevine, TX, 76051,
United States of America,
shouyiw@uta.eduChair: Danica Xiao, PhD Candidate, University of Washington, Seattle,
3900 Northeast Stevens Way, Seattle, WA, 98195,
United States of America,
xiaoc@uw.edu1 - A Dynamic Active-Set Method for Linear Programming
Alireza Noroziroshan, University of Texas at Arlington, 600 Grand
Ave, Apt#103, Arlington, TX, 76010, United States of America,
alireza.norozi.en@gmail.com,Bill Corley, Jay Rosenberger
An active-set method obtains solution for linear programming problems by
adding one or more constraints at a time to solve smaller problems iteratively. We
present an e?cient constraint selection rule for adding varying numbers of
constraints at each iteration. This approach is significantly faster than the standard
linear programming algorithms.
2 - Big Data Analytics for RFID-Enabled Logistics Data from
Ubiquitous Manufacturing Shopfloors
Ray Y. Zhong, Post-doctoral Fellow, The University of Hong Kong,
8-16 Haking Wong Building, IMSE, Pokfulam Road, HKU, Hong
Kong, Hong Kong - PRC,
zhongzry@gmail.com, George Q. Huang
RFID has been widely used in logistics and supply chain management. This paper
discusses the manufacturing shopfloor where typical logistics resources are
converted into smart manufacturing objects (SMOs) by using RFID and wireless
technologies to create a RFID-enabled intelligent shopfloor environment. In such
environment, enormous RFID data has been captured and collected. This paper
introduces a Big Data Analytics for the RFID logistics data by defining different
behaviors of the SMOs.
3 - On the Mixed Set Covering, Packing and Partitioning Polytope
Yong-Hong Kuo, Research Assistant Professor, The Chinese
University of Hong Kong, Shatin, N.T., Hong Kong - PRC,
yhkuo@cuhk.edu.hk, Janny Leung
We study the polyhedral structure of the mixed set covering, packing and
partitioning problem, derive the mixed odd hole inequality, and identify sufficient
condition for it to be facet-defining. In the special case when the induced graph is
a (mixed) odd hole, the inclusion of this new facet-defining inequality provides a
complete polyhedral characterization. Computational experiments show that
these new valid inequalities achieve a significant time reduction in solving the
mixed problems.
4 - Using Big-Data Analytics for Identifying Hot Spots of
Border Security
Haibo Wang, Killam Distinguished Asso Prof, Texas A&M
International University, 5201 University Blvd, Laredo, TX,
United States of America,
hwang@tamiu.edu, Yaquan Xu,
Jun Huang, Wei Wang
This project develops a comprehensive data aggregation and analysis system to
provide the decision support for identifying hot spots of border security using a
complex network model for transportation infrastructure in the border region. All
these research related data will be aggregated on both space and time dimensions
and analyzed by using “big data” models and tools developed in this study
MA16