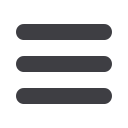

INFORMS Philadelphia – 2015
149
2 - Sparse but Efficient Operation: A Conic Programming Approach
Gao Yini, National University of Singapore, 1 Business Link,
Singapore, Singapore,
yini.gao@u.nus.edu,Chung Piaw Teo,
Zhenzhen Yan
Standardization and flexibility are two competing paradigms in designing efficient
operations. We ask whether there is a sparse but flexible operation mode to reap
the benefits of both. Using copositive conic programming, we develop a new
mechanism which gives sparse but efficient network structures. It recovers k
chain in the context of process flexibility. We further apply it to Singapore Changi
Airport “roving team” deployment problem and obtain a sparse yet efficient
deployment network.
3 - SDP Reformulation of CP Programs: Best-worst Choice and
Range Estimation Applications
Karthik Natarajan, Singapore University of Technology and
Design, Singapore, 487372, Singapore,
karthik_natarajan@sutd.edu.sg,Chung Piaw Teo
We show that the worst case moment bound on the expected optimal value of a
mixed integer linear program with a random objective c is obtained from a SDP
reformulation of a completely positive program. We illustrate the usefulness of
the distributionally robust bounds in estimating the expected range of random
variables with two applications arising in random walks and best-worst choice
models.
4 - Robust Inventory Models with Demand Partitioning Information
Joline Uichanco, Asst. Professor, University of Michigan, Ross
School of Business, 701 Tappan Ave, Ann Arbor, MI, 48109,
United States of America,
jolineu@umich.edu, Karthik Natarajan,
Melvyn Sim
We present the distributionally robust newsvendor with demand asymmetry
information through partition statistics. We derive a closed-form for the robust
order quantity under the special case of semivariance, implying a simple rule-of-
thumb for setting order quantities under limited information. The distribution can
be calibrated from primitive demand data. We demonstrate the performance of
the method in computational experiments on data from an automotive spare
parts company.
MA14
14-Franklin 4, Marriott
Stochastic Optimization Applications to Renewable
Energy Integration
Sponsor: Optimization/Optimization Under Uncertainty
Sponsored Session
Chair: Lindsay Anderson, Assistant Professor, Cornell University, 316
Riley Robb Hall, Ithaca, NY, 14853, United States of America,
landerson@cornell.edu1 - Multi-Objective Optimal Sensor Deployment under Uncertainty for
Advanced Power Systems
Urmila Diwekar, President, Vishwamitra Research Institute, 2714
Crystal Way, Crystal Lake, IL, 60012, United States of America,
urmila@vri-custom.org, Pallabi Sen, Kinnar Sen
Advanced power plants using an integrated gasification combined cycle (IGCC)
offer a competitive and economical means to produce electricity with reduced
emission levels. An efficient, safe, and reliable operation of an IGCC plant
requires effective strategies for monitoring and control. The results of this multi-
objective framework for optimizing observability, efficiency, and cost for an IGCC
system are presented in this work.
2 - Optimal Microgrid Design under Load and Photovoltaic
Power Uncertainty
Alex Zolan, University of Texas at Austin, 204 E. Dean Keeton
Street, Stop C2200, Austin, TX, 78712, United States of America,
alex.zolan@utexas.edu, Alexandra Newman, David Morton
We present a model for establishing the design and energy dispatch for a
microgrid that minimizes cost and fuel requirements given the set of technologies
(diesel generators, solar arrays and batteries), photovoltaic power (PV) availability
on location, and probability model that governs the load and PV availability of a
forward operating base. We introduce a policy-based restriction of the problem
that allows for the solution of a multiple scenario problem while preserving
solution quality.
3 - Tracking a Stochastic Generate-pump Schedule for a
Pumped-storage Hydroelectric Unit
Bismark Singh, The University of Texas at Austin, 204 E. Dean
Keeton Street, Stop C2200 ET, Austin, TX, 78705, United States
of America,
bismark.singh@utexas.edu, Surya Santoso
Using a stochastic dynamic program, we first optimize the generate-pump
schedule for a pumped-storage hydroelectric unit to maximize profit. Since
energy prices are stochastic, we find an adaptive policy for the schedule. And,
since we must submit bids to an ISO, we seek a bidding strategy that will allow us
to track the desired generate-pump schedule. Thus, we solve a model that yields
an optimal block-bidding policy in the sense of tracking the desired stochastic
generate-pump policy.
4 - A Stochastic Model to Determine Probabilistic Reserves
Requirements for Unit Commitment Problems
Gabriela Martínez, Cornell University, Ithaca, NY, United States of
America,
gabriela.martinez@cornell.edu, Lindsay Anderson
In this work, we propose a stochastic unit commitment model to decide
appropriate spinning and non-spinning reserve requirements for a power system
with high penetration of renewable energy. The day-ahead scheduling of the
systems is formulated as a chance-constrained model in which the network power
balance of the systems is ensured with a high-probability level and the system
reserves are allocated in a risk-averse fashion by selection of quantiles of the
uncertain generation.
MA15
15-Franklin 5, Marriott
Radiation Therapy Optimization
Sponsor: Optimization in Healthcare
Sponsored Session
Chair: Arka Roy, Bowling Green State University, 440 W. Barry Ave.,
Chicago, IL, United States of America,
arkaroy1@gmail.com1 - A Robust Optimization Method for Homogeneous Magnet Design
in MR-guided Radiation Therapy
Iman Dayarian, University of Toronto, 5 King’s College Road,
Toronto, ON, M4Y 2P9, Canada,
iman@mie.utoronto.ca,
Timothy Chan, Teodor Stanescu
Magnetic resonance imaging uses a magnetic field generated by a configuration of
coils to image patients. An optimization-generated coil configuration can be
sensitive to small perturbations that affect the homogeneity of the magnetic field.
This sensitivity is especially important when the coils are mounted on a treatment
device that rotates during treatment, which is the case in MR-guided radiation
therapy (MRgRT). This talk presents a robust optimization approach to magnet
design for MRgRT.
2 - Incorporating Lung Ventilation Function in Intensity-modulated
Radiation Treatment Planning
Fujun Lan, Postdoctoral Fellow, University of Maryland,
Baltimore, 22 S. Greene St., GGJ02, Baltimore, MD, 21201,
United States of America,
flan@email.arizona.edu,
Warren D’Souza, Hao Zhang
4DCT-derived ventilation images were used for pencil-beam intensity modulation
to achieve functional sparing of lung on a voxel-by-voxel basis. This functional
approach was compared to the conventional anatomical planning on 10 patients
retrospectively. Significant reductions (p-values < 0.001) of V20 (lung volume
receiving >=20Gy) (11%), functional V20 (18%), mean lung dose (MLD) (7%)
and functional MLD (11%) were achieved without significantly increasing doses
to the other organs-at-risk.
3 - Optimizing Global Liver Function in Stereotactic Body
Radiotherapy Treatment Planning
Victor Wu, PhD Student, University of Michigan, 1205 Beal
Avenue, Ann Arbor, MI, 48109, United States of America,
vwwu@umich.edu,H. Edwin Romeijn, Marina Epelman,
Martha Matuszak, Yue Cao, Mary Feng, Hesheng Wang,
Randall Ten Haken
We propose a radiotherapy treatment planning optimization model for liver
cancer cases. In this work, we plan treatment using voxel-based liver dose-
response model: post-treatment liver function depends on its pre-treatment
function and the dose received. We maximize predicted post-treatment global
liver function. We approximately solve the resulting non-linear non-convex
problem with a customized mixed-integer linear programming-based algorithm.
2D synthetic and 3D clinical cases were studied.
4 - Robust Adaptive Optimization in Radiation Therapy
Arka Roy, Bowling Green State University, 440 W. Barry Ave.,
Chicago, IL, United States of America,
arkaroy1@gmail.com,
Omid Nohadani
Radiotherapy treatments degrade over time in the presence of uncertainties.
Robust models leap beyond such limitations. However, traditional robust models
solve for the worst-case realization of the uncertainty prior to the start of the
treatment, which may be too conservative at later fractions. We propose a robust
two-stage approach that adapts to the first-stage decisions during treatment. The
results are demonstrated through a clinical prostate case.
MA15