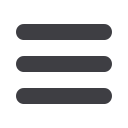

INFORMS Philadelphia – 2015
159
2 - Dynamic Assignment of Emergency Department Patients to
Primary and Secondary Inpatient Units
Derya Kilinc, Arizona State University, 699 S. Mill Ave.,
Tempe, AZ, 85281, United States of America,
dkilinc@asu.edu,
Soroush Saghafian, Stephen J. Traub
One of the main reasons for Emergency Department (ED) crowding is the long
boarding time, of patients who are waiting for admission to inpatient wards. We
study suitable mechanisms to overflow such patients to alternative wards. An
overflow policy can improve ED waiting times and Length of Stay (LOS), but may
reduce the quality of care. We study an MDP-based approach to gain insights into
the impact of overflow policies on waiting times and quality of care.
3 - Data Driven Staffing of Hospital Support Staff
Cassandra Hall, PhD Student, Northwestern University,
IEMS Department, Evanston, IL,
CassandraHall2017@u.northwestern.edu, Sanjay Mehrotra,
Seyed Iravani
We study hospital patient transport requests as a multiclass queue with server
specialization and construct an approximation for the minimum number of
servers required to achieve a probabilistic bound on the patient waiting time. We
also explore the effects of different specialization and routing policies on
performance at a given staffing level. Output is then compared with theoretical
worst case waiting time bounds derived from the literature.
4 - A Clinical Decision Support System for Treatment-resistant
Depression: A Pilot Study
Martin Cousineau, Desaultels Faculty of Management, McGill
University, 1001 Sherbrooke Street West, Montreal, QC, H3A
1G5, Canada,
martin.cousineau@mail.mcgill.ca, Gustavo Turecki,
Vedat Verter, Joelle Pineau
This research project aims to develop a clinical decision support system to assist
psychiatrists seeking to achieve remission in treatment-resistant depression
patients. This system is based on a longitudinal dataset of outpatient mental
health clinic patients, and consists of (1) a predictive model of the patient
outcome depending on the selected treatment, and (2) a decision-theoretic
module for recommending treatment strategies based on similar patient files.
5 - An Empirical Study of Patient Prioritization in Emergency
Department Triage Systems
Mahesh Nagarajan, University of British Columbia,
2053 Main Mall, Vancouver, BC, V6T1Z2, Canada,
mahesh.nagarajan@sauder.ubc.ca, Eric Park, Yichuan Ding
We analyze patient choice behavior of the ED personnel who decides which
patient waiting in the ED will be seen by the next available physician. We use a
discrete choice framework consistent with random utility theory. The choice
maker’s valuation of each patient depends on both the patient’s medical and
operational characteristics including wait time and ED congestion. We study over
270,000 patient choices in five EDs using the Canadian Triage and Acuity Scale
(CTAS).
MA42
42-Room 102B, CC
Modeling Healthcare Provider and
Processes Interaction
Sponsor: Manufacturing & Service Oper
Mgmt/Healthcare Operations
Sponsored Session
Chair: Vikram Tiwari, Vanderbilt University Medical Center, Nashville,
TN, United States of America,
vikram.tiwari@vanderbilt.edu1 - Quantifying Case Cancellations and Add-On Case Scheduling
among Patients Inpatient Preoperatively
Franklin Dexter, Professor, University of Iowa, Anesthesia,
200 Ha, Iowa City, IA, 52242, United States of America,
franklin-dexter@uiowa.edu, Richard Epstein
Among 24,735 inpatient scheduled cases, 22.6 ±0.5% (SE) of scheduled minutes
cancelled after 7 AM the day before or day of surgery (14.0% ±0.3% day of).
Most (83.1% ±0.6%) cases performed were evaluated the day before surgery,
62.3% ±1.5% before 6:00 PM. The cancelled procedures were very diverse
(Herfindahl index 0.021 ±0.001). When cancelled, often no procedure was
subsequently performed (50.6% ±0.9%), showing the surgical indication no
longer existed or patient/family decided no surgery.
2 - The Role of Wearable Devices Data in
Physician-patient Relationship
Zafar Özdemir, Professor, Miami University, Farmer School of
Business, Oxford, OH, 45056, United States of America,
ozdemir@miamioh.edu,Shailesh Kulkarni, Hakan Tarakci
We investigate a medical provider’s optimal level of investment in remote
monitoring where, unlike the current norm, the provider can initiate a face-to-
face visit or a remote treatment depending on the information streamed from the
patient’s wearable device. Our model provides valuable insights across a variety of
payment models.
3 - Evaluating Peer-to-peer Performance of Anesthesiology Fellows
using Data Envelopment Analysis
Vikram Tiwari, Vanderbilt University Medical Center, Nashville,
TN, United States of America,
vikram.tiwari@vanderbilt.edu,
Avinash Kumar
Factors that contribute to the success of individuals at a critical care fellowship
have not been well studied. We explore what aspects of the educational program
and work characteristics contribute the most to an individual fellow’s success as
determined by year end Multidisciplinary Critical Care Knowledge Assessment
Program scores and summative evaluations. We show the feasibility of using data
envelopment analysis to evaluate the academic performance of fellows compared
to their peers.
MA43
43-Room 103A, CC
Game Theoretic Models in Revenue Management I
Sponsor: Revenue Management and Pricing
Sponsored Session
Chair: Ozan Candogan, University of Chicago, Booth School of
Business, Chicago, IL, United States of America,
ozan.candogan@chicagobooth.eduCo-Chair: Santiago Balseiro, Assistant Professor, Duke University,
100 Fuqua Drive, Durham, NC, 27708, United States of America,
srb43@duke.edu1 - Customer Referral Incentives and Social Media
Ilan Lobel, NYU, 44 W 4th St, New York, NY, 10012,
United States of America,
ilobel@stern.nyu.edu, Evan Sadler,
Lav Varshney
We study how to optimally attract new customers using a referral program.
Whenever a consumer makes a purchase, the firm gives her a link to share with
friends, and every purchase coming through that link generates a referral
payment. The firm chooses the referral payment function and consumers play an
equilibrium in response. We show that the optimal payment function is nonlinear
and complex, and prove revenue properties of simple approximate solutions such
as linear and threshold policies.
2 - Dynamic Reserve Prices for Repeated Auctions:
Learning from Bids
Yash Kanoria, Assistant Professor, Columbia University,
New York, NY, United States of America,
ykanoria@columbia.edu,
Hamid Nazerzadeh
A large fraction of online advertisements are sold via repeated second price
auctions. In these auctions, the reserve price is the main tool for the auctioneer to
boost revenues. We present a simple approximately incentive-compatible and
optimal dynamic reserve mechanism that can significantly improve the revenue
over the best static reserve when there is uncertainty in the distribution of
valuations.
3 - A Dynamic Model of Crowdfunding
Mohamed Mostagir, Assistant Professor, University of Michigan
Ross School of Business, 701 Tappan Ave, R5316, Ann Arbor, MI,
48109, United States of America,
mosta@umich.edu,Saeed Alaei,
Azarakhsh Malekian
Crowdfunding has emerged as an alternative to traditional methods of funding
new products. Backers arrive over time and decide whether to pledge money to a
crowdfunding campaign. If the total contribution reaches a certain threshold, the
campaign is successful and production takes place. We identify a fundamental
tension in these environments that leads to a sharp characterization of empirical
outcomes, and we show how to determine the optimal duration and price to
maximize campaign success.
4 - Effect of Network Perturbation on Aggregate Performance
Azarakhsh Malekian, Rotman School of Management,
University of Toronto, Toronto, Canada,
azarakhsh.malekian@rotman.utoronto.ca,Opher Baron, Ming Hu
In this work, we characterize the role of perturbing network interactions in
macroeconomic aggregate performance. We then provide a fairly tight
characterization of the aggregate performance under worst-case network
perturbation as well as average-case random network perturbation. Finally, we
identify robust networks under perturbation.
MA43