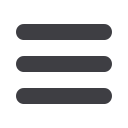

INFORMS Philadelphia – 2015
160
MA44
44-Room 103B, CC
Data Driven Pricing
Sponsor: Revenue Management and Pricing
Sponsored Session
Chair: Wedad Elmaghraby, Associate Professor, University of Maryland,
University of Maryland, 4311 Van Munching Hall, College Park, MD,
20742, United States of America,
welmaghr@rhsmith.umd.eduCo-Chair: Shawn Mankad, Assistant Prof Of Business Analytics,
University of Maryland, 4316 Van Munching Hall, College Park, MD,
21201, United States of America,
smankad@cornell.edu1 - More than Just Words: on Discovering Themes in Online Reviews
to Explain Restaurant Closures
Shawn Mankad, Assistant Prof Of Business Analytics, University
of Maryland, 4316 Van Munching Hall, College Park, MD, 21201,
United States of America,
smankad@cornell.edu,Anand Gopal,
Jorge Mejia
We complement the existing research on online reviews by proposing a novel use
of modern text analysis methods to uncover the semantic structure of online
reviews and assess their impact on the survival of merchants in the marketplace.
We analyze online reviews from 2005 to 2013 for restaurants in a major
metropolitan area in the United States and find that variables capturing semantic
structure within review text are important predictors of the survival of
restaurants.
2 - Cost-per-impression Pricing for Display Advertising
Sami Najafi-Asadolahi, Santa Clara University, 500 El Camino
Real, Santa Clara, CA, United States of America,
snajafi@scu.eduWe consider a web publisher posting display ads on its website and charging based
on the cost-per-impression pricing scheme. The publisher is faced with uncertain
demand for advertising slots and uncertain supply of visits from viewers.
Advertisers choose ad campaigns that specify their targeted viewers. We
determine the publisher’s optimal price to charge and show that it can increase in
the number of impressions, which is in contrast to the quantity-discount
commonly offered in practice.
3 - Pricing Personalized Bundles: A New Approach and
Industrial Application
Zhengliang Xue, IBM, Yorktown Heights, NY, United States of
America, IBM,
zxue@us.ibm.com,Zizhuo Wang, Markus Ettl
We optimize the pricing strategies for personalized bundles where a seller
provides a variety of products using which customers can configure a bundle and
send a request-for-quote. The seller, after reviewing the RFQ, has to determine a
price based on customers’ willingness to buy. Such problems are difficult because
of the potential unlimited possible configurations of the bundle and the
correlations among individual products. We propose a new approach and show
the business value by real data.
4 - Measuring the Effects of Advertising: The Digital Frontier
Justin Rao, Researcher, Microsoft Research, 641 Avenue of
Americas, New York, NY, 10014, United States of America,
Justin.Rao@microsoft.com, Randall Lewis, David Reiley
Online advertising offers unprecedented opportunities for measurement. A host
of new metrics have become widespread in advertising science. New
experimentation platforms open the door for firms and researchers to measure
the true causal impact of advertising. We dissect the new metrics, methods and
computational advertising techniques currently used by industry researchers,
highlighting their strengths and weaknesses, and discuss the novel analyses could
impact the advertising market.
MA45
45-Room 103C, CC
RM in Practice
Sponsor: Revenue Management and Pricing
Sponsored Session
Chair: Wei Wang, Scientist, PROS, Inc., 3100 Main Street, Suite #900,
Houston, TX, 77002, United States of America,
weiwang@pros.com1 - Modeling Issues and Best Practices in Price and
Revenue Optimization
Yanqi Xu, Director Of Applied Technology, Princess Cruises,
24305 Town Center Rd, Valencia, CA, 91355,
United States of America,
yxu@princesscruises.comPrice and revenue optimization is proven to be critical to improving the top lines
in various industries where relatively fixed capacity has to be used to satisfy
fluctuating demand. However, there exist ways to set up the models to achieve
better profits than others. In this talk, we will discuss some practical issues
frequently encountered in the modeling of price and revenue optimization, and
we will go through several real world examples to illustrate some of the best
practices.
2 - Revenue Functions: Demand Aggregation for Fleet Allocation in
Car Rental Industry
Manu Chaudhary, Scientist, PROS, 3100 Main Street,
Suite #900, Houston, TX, 77002, United States of America,
mchaudhary@pros.comFleet allocation for car rental industry is a multi-dimensional, large scale network
optimization which is computationally expensive. We address this complexity by
introducing an intermediate optimization that generates Revenue Functions
which aggregate the demand forecast to a higher level. This reduces the
dimensional complexity of the modified fleet allocation problem significantly and
makes it feasible to run in a live production system.
3 - Identifying a More Accurate Historical Data Subset from a Noisy
Historical Dataset: A Forecasting Example
Gregory Vogel, Manager, Revenue Science, Holland America
Line, 300 Elliott Ave W, Seattle, WA, 98119,
United States of America,
gvogel@hollandamerica.comWhen looking at similar products that have overlapping booking periods, noise is
common. It is common practice to compile a dataset for processes such as demand
forecasting by compiling the complete set of relevant history. We ask the
question, can we identify a subset of history that will produce a more accurate
forecast? We utilize a cruise example to demonstrate and improved forecast and
present the method developed.
4 - Joint Optimization of Pricing and Marketing for Globally
Maximum Profit
Sharon Xu, UCLA Statistics Department, 8125 Math Sciences,
Los Angeles, CA, 90095, United States of America,
sharon.xu@ucla.eduWith the recent influx of high-granularity data, businesses are able to achieve a
more comprehensive understanding of their customers. To better leverage this
data, we present a new way to maximize profit by jointly optimizing pricing and
advertising spend. We first create a predictive model to quantify how pricing and
advertising influence consumer purchase decisions, then use this to inform a
model that simultaneously optimizes pricing and advertising decisions to obtain
the maximum profit.
5 - An Airline RM Model with Capacity Sharing
Ang Li, Scientist, PROS, Inc., 3100 Main St. #900, Houston, TX,
77002, United States of America,
ali@pros.com, Darius Walczak
We consider a single-leg airline RM problem with shared seating capacities
between business and economy compartments. In particular, a curtain that
separates the two compartments can be installed on the day of flight departure.
We solve the control problem as a dynamic program that jointly considers the
booking levels on both sides of the curtain. We then compare the optimal revenue
achieved to an alternative scenario with an optimal curtain position but without
capacity sharing.
MA46
46-Room 104A, CC
Healthcare Operations
Sponsor: Manufacturing & Service Oper Mgmt/Service Operations
Sponsored Session
Chair: Carri Chan, Columbia Business School, 3022 Broadway,
Uris Hall, Room 410, New York, NY, 10027, United States of America,
cwchan@columbia.edu1 - Service Decisions with Two-dimensional Customer Heterogeneity
Tolga Tezcan, Associate Professor, London Business School,
Regent’s Park, London, UK, NW14SA, United Kingdom,
ttezcan@london.edu, Balaraman Rajan, Avi Seidmann
In this work we analyze the operational decisions of a server dealing with
customers who are heterogeneous on two dimensions. We apply the results in the
context of a specialist seeing patients suffering from chronic conditions and
patients who differ in their preferences to a newly introduced telemedicine
technology. Our results help analyze telemedicine adoption and optimal decisions
for a service provider.
MA44