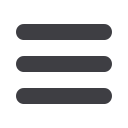

INFORMS Philadelphia – 2015
237
MD16
16-Franklin 6, Marriott
Application of Linear and Conic Programs with
Complementarity Constraints
Sponsor: Optimization/Linear and Conic Optimization
Sponsored Session
Chair: Xin Shen, RPI, 110 8th Street, Troy, NY, 12180,
United States of America,
shenx5@rpi.edu1 - Robust Optimization for Network Design Problems with
Equilibrium Flows
Liu Su, Iowa State University, 0076 Black Engineering, Ames, IA,
50011, United States of America,
suliu@iastate.edu,Lizhi Wang,
Guiping Hu
To identify optimal network capacity expansion, we build up a bi-level model for
network design problems with equilibrium flows under the robust optimization
paradigm. We transformed the lower level problem into a mixed-integer linear
program and use a branch and cut algorithm to solve the bi-level optimization
problem.
2 - Application of Complementarity Problems in Multibody
Dynamics and Robotics
Ying Lu, Rensselaer Polytechnic Institute, 2408 21st St. Apt. 6,
Troy, NY, 12180-1811, United States of America,
rosebudflyaway@gmail.com,Jeff Trinkle
Frictional contacts in multibody dynamics and robotic simulation are generally
written as differential Complementarity Problems (dCPs), which are solved as a
series of CPs. We compare several models and solution algorithms, as well as a
GPU based CUDA parallel solver to to solve the Complementarity Problems
arising in physical simulation.
3 - Property of a Relaxation Scheme for Rank Constrained
Optimization Problems
Xin Shen, RPI, 110 8th Street, Troy, NY, 12180,
United States of America,
shenx5@rpi.edu, John Mitchell
Recently rank constrained optimization problems have received increasing
interest because of their wide application. This class of problems has been
considered computationally challenging because of its nonconvex nature. In this
talk we focus on a mathematical program with semidefinite cone
complementarity constraints formulation of the class. We’ll consider a relaxation
scheme for the formulation and discuss its properties including stationary
conditions and local optimality.
4 - Heuristics for QPLCCS using Nlp Solvers Aided by
Semidefinite Relaxations
Patricia Gillett, PhD Candidate, Département de Mathématiques
et de Génie Industriel, École Polytechnique de Montréal,
Montréal, QC, Canada,
patricia-lynn.gillett@polymtl.ca,Miguel Anjos, Joaquim Júdice
We present a semidefinite programming relaxation technique with iterative
cutting planes for quadratic programs with linear complementarity constraints
(QPLCC). We discuss how an optimal solution to the SDP relaxation can be used
to warmstart the solution of the QPLCC using common local and global NLP
solvers. We report some numerical results demonstrating the quality of the SDP
bound and the effectiveness of the warmstarting procedures.
MD17
17-Franklin 7, Marriott
Modeling Social Influence in Networks
Sponsor: Optimization/Network Optimization
Sponsored Session
Chair: Vladimir Boginski, University of Florida, 303 Weil Hall,
Gainesville, FL, United States of America,
boginski@reef.ufl.edu1 - Fashion Supply Chain Network Competition with Ecolabelling
Min Yu, Assistant Professor, University of Portland, 5000 N.
Willamette Blvd., Portland, OR, 97203, United States of America,
yu@up.edu, Jonas Floden, Anna Nagurney
We develop a competitive supply chain network model for fashion that
incorporates ecolabelling. We capture the individual profit-maximizing behavior
of the fashion firms which incur ecolabelling costs with information associated
with the carbon footprints of their supply chains revealed to the consumers.
Consumers, in turn, reflect their preferences for the branded products of the
fashion firms through their demand price functions, which include the carbon
emission information.
2 - Impact of Sub-networks on the Diffusion of Innovation
Xu Dong, Research Assistant, University of Miami, 1251
Memorial Drive, Coral Gables, FL, 33146, United States of
America,
x.dong3@umiami.edu,Nazrul Shaikh
Extant research shows that the structural properties of social networks influence
the diffusion of innovation; however, these studies assume that the network is
one giant cluster. Networks can have disconnected clusters (sub-networks) that
introduce discontinuities in the diffusion pathways. Our research provides an
understanding of the impact of discontinuities on diffusion.
3 - Identifying High Value Customers in a Network: Individual
Characteristics Versus Social Influence
Sang-Uk Jung, Assistant Professor, Hankuk University of Foreign
Studies, Imunro 102, Dongdaemun-gu, Seoul, 130-791, Korea,
Republic of,
sanguk.jung@hufs.ac.kr,Qin Zhang, Gary Russell
Firms are interested in identifying customers who generate the highest revenues.
In a social network setting, customer interactions can play an important role in
purchase behavior. This study proposes a spatial autoregressive model that
explicitly shows how network effects and individual characteristics interact in
generating firm revenue.Using model output, we develop a method of identifying
individuals whose purchase behavior most impacts the total revenues in the
network.
MD18
18-Franklin 8, Marriott
Methodologies in Text Mining for Big Data
Cluster: Modeling and Methodologies in Big Data
Invited Session
Chair: Onur Seref, Virginia Tech, 2060 Pamplin Hall (0235),
Blacksburg, VA, 24061, United States of America,
seref@vt.edu1 - A Tangled Web: Evaluating the Impact of Displaying Fraudulent
Reviews on Review Portals
Uttara Ananthakrishnan, Carnegie Mellon University, 5000
Forbes Ave, Pittsburgh PA 15213, United States of America,
umadurai@andrew.cmu.edu,Michael D Smith, Beibei Li
This paper studies how users respond to fraudulent reviews and how platforms
can leverage such knowledge to design better fraud management. We combine
randomized experiments, behavioral economics with machine learning using
large-scale data from Yelp. We find to improve user trust platforms should display
the fraudulent information. Finally, our statistical analysis using MLE allows us to
design a novel fraud-awareness reputation system.
2 - Strength in Numbers: Can Big Data Eliminate the Need for
Complex Opinion-mining Algorithms?
Theodoros Lappas, Assistant Professor, Stevens Institute of
Technology, 335 Washington, Apt. 2, Hoboken, NJ, 07030,
Greece,
tedlappas@gmail.comOpinion mining, defined as the task of identifying the polarity of text segments, is
a building block for many popular applications. Relevant methods have evolved
from simple lexicon-based approaches to expensive NLP algorithms that try to
emulate human thought. What if there was a simpler way to capture complex
linguistic patterns, by utilizing the unprecedented availability of opinion-rich
datasets? Our work addresses this question and motivates a new line of
applications for Big Data.
3 - Restaurant Hygiene Grades and Online Reviews
Jorge Mejia, University of Maryland, Robert H. Smith School of
Business, College Park, MD, United States of America,
jmejia@rhsmith.umd.edu,Shawn Mankad, Anand Gopal
We focus on understanding the relationship between online reviews and a
significant public health problem: restaurant-related foodborne illness. Recent
initiatives to publicize the results of restaurant health inspections have been
shown to reduce the occurrence of foodborne illness. We use the semantic
information in online reviews to forecast health inspection results for restaurants
in NYC. This approach can be used to improve the effectiveness of health
inspection programs.
4 - Automatic Sequence Extraction for Sequence Alignment in
Text Mining
Michelle Seref, Virginia Tech, Pamplin 1007, 0235, Blacksburg,
VA, 24061, United States of America,
mmhseref@vt.edu,
Onur Seref
We illustrate novel methods to automatically extract sequences from pre-labeled
text in order to apply sequence alignment for classifying text. Sequences are
initially generated using n-gram approaches and then aggregated into
semantically unique sequences. Sequence alignment uses these sequences to
detect semantically equivalent text with either exact word or synonym matches.
We demonstrate our method on several text domains.
MD18