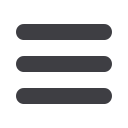

INFORMS Philadelphia – 2015
233
MD04
04-Room 304, Marriott
Panel: International Opportunities
Sponsor: Junior Faculty Interest Group
Sponsored Session
Chair: Raha Akhavan-Tabatabaei, Associate Professor, Universidad de
los Andes, Carrera 1 Este # 19 A - 40, Bogota, Colombia,
r.akhavan@uniandes.edu.coCo-Chair: Shengfan Zhang, Assistant Professor, University of Arkansas,
4207 Bell Engineering Center, Fayetteville, United States of America,
shengfan@uark.edu1 - A Panel Discussion on International Opportunities
Moderator:Shengfan Zhang, Assistant Professor, University of
Arkansas, 4207 Bell Engineering Center, Fayetteville, AR,
United States of America,
shengfan@uark.edu, Panelists:
Dionne Aleman, Andres Medaglia, Fugee Tsung
This panel consists of department heads, junior and senior faculty members of
universities in Canada, Colombia, Hong Kong and Turkey. They will discuss
opportunities in academic jobs, tenure and promotion processes, research
resources (funding and visiting opportunities), professional development,
recruiting, etc.
MD05
05-Room 305, Marriott
Predicting Customer Behavior using Facebook Data
Cluster: Social Media Analytics
Invited Session
Chair: Michel Ballings, Assistant Professor Of Business Analytics, The
University of Tennessee, 249 Stokely Management Center, Knoxville,
TN, 37996, United States of America,
michel.ballings@utk.edu1 - Using Customers’ Facebook Pages to Improve Lead Qualification
in a B2B Acquisition Process
Matthijs Meire, PhD Student, Ghent University,
Tweekerkenstraat, 2, Ghent, 9000, Belgium,
Matthijs.Meire@ugent.be,Michel Ballings, Dirk Van Den Poel
The purpose of this study is to investigate the added value of Facebook data in
B2B customer acquisition. We use a Random Forest prediction model. The results
indicate that adding customers’ Facebook page data can indeed improve B2B lead
qualification. Our contribution is twofold. First, to the best of our knowledge it is
the first to use Facebook data in B2B lead qualification. Second, we quantify the
monetary gains of using Facebook data by conducting a real-life lead targeting
experiment.
2 - Investigating the Drivers of Likes and Comments on Facebook
Steven Hoornaert, PhD Student, Ghent University, Ghent, 9000,
Belgium,
Steven.Hoornaert@ugent.be,Michel Ballings,
Dirk Van Den Poel
The objective of this study is to investigate the added value of user context data in
Facebook post popularity prediction models. For this purpose, two Random Forest
models were built: one including only post variables (e.g., post type) and another
containing both post and user variables (e.g., age). Predictability is improved for
likes (x3.7) and comments (x3.6). This study is the first to augment post
popularity prediction models with user context data and analyze a large quantity
of posts.
3 - Predicting Buyer Behavior using Social Media Data
Matthias Bogaert, PhD Student, Ghent University,
Tweekerkenstraat 2, Ghent, 9000, Belgium,
matthias.bogaert@ugent.be, Michel Ballings, Dirk Van Den Poel
The purpose of this study is to explain customer behavior (offline event
attendance) based on SM data. In order to substantiate our findings, we used
propensity score matching and built a Random Forest model. This study reveals
that social media data can predict offline event attendance with high predictive
accuracy. Moreover, the results suggest that the number of friends that are
attending the focal event and event attendance on Facebook were highly
significant.
4 - The Power of Facebook to Predict Customer Acquisition
and Defection
Michel Ballings, Assistant Professor Of Business Analytics,
The University of Tennessee, 249 Stokely Management Center,
Knoxville, TN, 37996, United States of America,
michel.ballings@utk.edu, Matthijs Meire, Dirk Van Den Poel
The main purpose of this study is to investigate the value of Facebook data in
predicting individual customer behavior. In addition we study the importance of
different online engagement variables such as likes, answers to event rsvp’s, and
group memberships in predicting acquisition and defection. The results indicate
that customer acquisition can be predicted very accurately using Facebook data.
In addition Facebook data significantly improve defection prediction over and
above customer data.
MD06
06-Room 306, Marriott
Finance and Risk Management
Sponsor: Financial Services
Sponsored Session
Chair: Samim Ghamami, Board of Governors of the Federal Reserve
System, 20th Street and Constitution Avenue N.W., Washington, DC,
United States of America,
samim.ghamami@frb.gov1 - Derivatives Pricing under Bilateral Counterparty Risk
Samim Ghamami, Board of Governors of the Federal Reserve
System, 20th Street and Constitution Avenue N.W., Washington,
DC, United States of America,
samim.ghamami@frb.govWe consider risk-neutral valuation of a contingent claim under bilateral
counterparty risk in a setting similar to that of Duffie and Singleton (1999). We
develop probabilistic valuation formulas that have closed-form solution or can
lead to computationally efficient pricing schemes. Drawing upon the work of
Ghamami and Goldberg (2014), we show that derivatives values under wrong
way risk (WWR) need not be less than the derivatives values in the absence of
WWR.
2 - Stochastic Intensity Margin Modeling of Credit Default
Swap Portfolios
Dong Hwan Oh, Economist, Federal Reserve Board, 20th Street
and Constitution Avenue N.W., Washington, DC, 20551, United
States of America,
donghwan.oh@frb.gov,Samim Ghamami,
Baeho Kim
We consider the problem of initial margin (IM) modeling for portfolios of credit
default swaps (CDS) from the perspective of a derivatives CCP. Inspired by Cont
and Kan (2011), the CCPs’ IM models in practice are based on theoretically-
unfounded direct statistical modeling of CDS spreads. Using the well-known
reduced-form approach, our IM model prices the portfolio constituents in a
theoretically meaningful way and shows that statistical IM models can
underestimate CCPs collateral requirements.
3 - Evaluating Central Counterparty Risk
Anton Badev, Economist, Federal Reserve Board, 1801 K St. NW,
Washington, DC, 20006, United States of America,
anton.i.badev@frb.gov,Samim Ghamami
A conceptually sound and logically consistent definition of the CCP risk capital is
challenging, and incoherent CCP risk capital requirements may create an obscure
environment. Based on novel applications of well-known mathematical models in
finance, this paper introduces a risk measurement framework that coherently
specifies all layers of the default waterfall resources of typical derivatives CCPs.
We apply the proposed framework on DTCC data and evaluate various risk
management practices.
4 - Risk Screening in Microfinance: Modeling and an Extragradient-
based Online Learning Algorithm
Yuqian Xu, NYU Stern School of Business, 44 West 4th Street,
New York City, NY, 10002, United States of America,
yxu@stern.nyu.edu, Michael Pinedo, Binqing Xiao
In this paper, we get the business loan application and default data from one of
the leading banks in China. We then propose a statistical model with three
different types of indexes to quantify the potential performance of a firm: its
financial level index, operational level index, and business owner level index and
provide an efficient extragradient-based online learning algorithm to solve it.
MD06