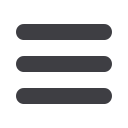

INFORMS Philadelphia – 2015
230
3 - Quantitative and Qualitative Evaluation of Printed Electronics
Based on Microscopic Images
Hongyue Sun, Virginia Tech., Grado Department of Industrial
and, Systems Engineering, Blacksburg, VA, 24061, United States
of America,
hongyue@vt.edu, Yifu Li, Chuck Zhang, Ran Jin,
Kan Wang
Aerosol jet printing is an additive manufacturing technology to fabricate printed
electronics. Although various types of machine vision sensors are used to take
images for qualitative evaluation, no methods have been reported to use image
features to quantitatively characterize the quality of electronics. This work use a
quantitative method to model the correlation of image features and quality
variables. A case study to fabricate silver conducting wires is used to evaluate the
performance.
4 - On the Asymptotics of Pairwise Modeling for Multivariate
Gaussian Process
Yongxiang Li, Research Assistant, City University of Hong Kong,
Department of SEEM, 83 Tat Chee Avenue, Kowloon,
Hong Kong - PRC,
novern.li@gmail.com, Qiang Zhou
Multivariate Gaussian process is a popular method for emulating computer
models with multiple outputs. But its complexity poses significant challenges to
parameter estimation due to high dimensionality and huge computational
burden. A pairwise modeling approach is proposed to solve the issue. The
asymptotic normality for parameter estimation is studied. Simulation studies are
conducted and the pairwise method is applied to model the low-E glass data for
such purposes as quality control.
MC74
74-Room 204A, CC
Modern Monitoring Applications
Sponsor: Quality, Statistics and Reliability
Sponsored Session
Chair: Irad Ben-Gal, Professor, Tel Aviv University, Tel Aviv, Israel,
bengal@tauex.tau.ac.il1 - An Application of Sensor Selection Based on Information
Theoretic Measurements for Change Detection
Marcelo Bacher, PhD Candidate, Tel Aviv University, Ramat Aviv,
Tel Aviv, Israel,
mgbacher@post.tau.ac.il, Irad Ben-Gal
Feature selection based on Information Theoretic measurements has been used
with great success in Machine Learning applications in special for classification
tasks. Nevertheless, less effort has been applied to process monitoring. In this
work we propose a framework that aims at finding the most significant subset of
features for change detection and bounded false alarm rate when monitoring a
process.
2 - Correlated Gamma-based Hidden Markov Model for Asthma
Control Status Diagnosis
Junbo Son, PhD Candidate, University of Wisconsin-Madison,
1513 University Avenue, Madison, WI, 53706, United States of
America,
json5@wisc.edu, Patricia Brennan, Shiyu Zhou
To effectively manage the asthma as a chronic disease, a statistical model based on
the everyday patient monitoring is crucial. Taking advantages from the remote
patient monitoring system, we propose a data-driven diagnostic tool for assessing
underlying asthma condition of a patient based on hidden Markov model (HMM).
The proposed correlated gamma-based HMM can visualize the asthma progression
to aid therapeutic decision making. Its promising features are shown in both
simulation and case study
3 - Project Management Monitoring
Irad Ben-Gal, Professor, Tel Aviv University, Tel Aviv, Israel,
bengal@tauex.tau.ac.ilWe consider the monitoring of large projects (software/hardware) and propose an
analytical approach for identifying the optimal project monitoring points by using
concepts from the Information Theory. The methodology used is based on
simulation-optimization scheme - selecting the monitoring points that provide the
highest potential information gain on the project duration. (joint work with Shiva
Kashi-Cohen and Shay Rozanes)
4 - Leveraging Analytics to Support Health-monitoring and
Management of Infrastructure Facilities
Pablo Durango-Cohen, Associate Professor, Northwestern
University, 2145 Sheridan Road, A332, Evanston, IL, 60208,
United States of America,
pdc@northwestern.edu, Yikai Chen
Motivated by recent technological advances, we describe the development and
validation of a statistical framework to support health-monitoring and
management of transportation infrastructure. The framework consists of
formulation of structural time-series models to explain, predict, and control for
common-cause variation, and use of multivariate control charts to detect special-
cause variation. We present several examples from an in-service bridge to validate
the framework.
MC75
75-Room 204B, CC
Innovations in Healthcare Products and Services
Cluster: New Product Development
Invited Session
Chair: Nitin Joglekar, Boston University, Questrom School of Business,
Boston, MA, United States of America,
joglekar@bu.edu1 - Healthtech Platforms: Barriers to Innovation
Edward Anderson, Professor, McCombs School of Business, The
University of Texas at Austin, 1 University Station B6500, Austin,
TX, 78712-1277, United States of America,
Edward.Anderson@mccombs.utexas.edu, Shi Ying Lim
The state of mobile and digital health is far behind that of other platform
industries, such as travel, retail, and even banking. Using qualitative analysis, we
present some of the more important barriers to healthtech startup success (and,
but extension, health tech in general) and outline some initial suggestions to
create an ecosystem to counter them.
2 - Platform Innovations in Healthcare Delivery
Geoffrey Parker, Professor, Tulane University, 7 McAlister Drive,
New Orleans, LA, 70118, United States of America,
ggparker@tulane.eduNetwork platform systems have reshaped the computer and telecommunications
industries and are now transforming other industries such as transportation,
lodging, and contract labor. The shift to platforms is slower in highly regulated
industries, but changes are coming quickly. We survey likely mechanisms and
entry points for a platform shift in healthcare.
3 - Patient, Heal Thyself! A Learning Algorithm to Predict How
Telemedicine Affects Patient Activation
Kellas Cameron, PhD Student, Boston University, Questrom
School of Business, Boston, MA, 02215, United States of America,
kellas@bu.edu, Carrie Queenan, Nitin Joglekar
The Patient Activation Measure (PAM) assesses an individual’s knowledge and
confidence for managing one’s health. This paper proposes a learning algorithm to
predict a patient’s PAM with data from a controlled telemedicine study,
accounting for social and technology effects. The algorithm allows for the analysis
of Type I and II errors and learning versus testing tradeoffs. Implications of this
study create opportunities for operational improvements to reduce patient
readmission rates.
MC76
76-Room 204C, CC
Accounting for Input Uncertainty in Stochastic
Simulations
Sponsor: Simulation
Sponsored Session
Chair: Canan Gunes Corlu, Assistant Professor, Boston University, 808
Commonwealth Avenue, Boston, MA, 02215, United States of America,
canan@bu.edu1 - A Sequential Experiment Design for Input Uncertainty
Quantification in Stochastic Simulation
Xie Wei, Assistant Professor, Rensselaer Polytechnic Institute,
400 McChesney Ave. Ext. 5-9, Troy, NY, United States of America,
xiew3@rpi.eduWhen we use simulations to estimate the performance of a stochastic system,
simulations are often driven by input distributions that are estimated from real-
world data. Non-parametric bootstrap could be used to quantify both input model
and parameter uncertainty. A sequential experiment design is proposed to
efficiently propagate the input uncertainty to output mean and deliver a
percentile confidence interval to quantify the impact of input uncertainty on the
system performance estimate.
2 - Input Uncertainty in Stochastic Simulations: Dependent Input
Variables of Mixed Types
Alp Akcay, Eindhoven University of Technology, Department of
Industrial Engineering, Netherlands,
A.E.Akcay@tue.nl,
Bahar Biller
We consider stochastic simulations with correlated input random variables having
NORmal-To-Anything (NORTA) distributions. We assume that the marginal
distribution functions and the NORTA base correlation matrix are unknown.
Given that the dependent input variables can take discrete and continuous values,
we develop a Bayesian procedure that decouples the input model estimation into
two stages. We investigate the role of the corresponding input uncertainty in
simulation output data analysis.
MC74