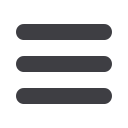

INFORMS Philadelphia – 2015
343
4 - Optimization Model for Floating Carsharing System Planning
Antonio Antunes, Professor, University of Coimbra, Dept. of Civil
Engineering, Coimbra, Portugal,
antunes@dec.uc.ptThe focus of this presentation is an optimization model aimed to assist a floating
carsharing company in the making of its key planning decisions – the area to be
operated by the company (called home area), the price rate or rates to be charged
to customers, the relocation operations to perform across zones of the home area,
and, indirectly, the size of the fleet to be used by the company. The results that
can be obtained through the model are exemplified for a real-world setting.
TC72
72-Room 203A, CC
DDDAS for Industrial and System Engineering
Applications III
Sponsor: Quality, Statistics and Reliability
Sponsored Session
Chair: Shiyu Zhou, Professor, University of Wisconsin-Madison,
Department of Industrial and Systems Eng, 1513 University Avenue,
Madison, WI, 53706, United States of America,
shiyuzhou@wisc.eduCo-Chair: Yu Ding, Professor, Texas A&M University, ETB 4016,
MS 3131, College Station, TX, United States of America,
yuding@iemail.tamu.edu1 - The Predict Project: Enhancing DDDAS/Infosymbiotics with
Privacy and Security
Vaidy Sunderam, Emory University, 400 Dowman Dr #W-401,
Math & CS, Atlanta, GA, 30322, United States of America,
vss@emory.edu, Li Xiong
The ubiquitousness of mobile devices will greatly expand the applicability of
DDDAS, provided privacy and security issues are addressed. The PREDICT project
is developing: (1) approaches to assign data-targets to participants with privacy
protection; (2) methods for aggregating and fusing data that quantify veracity of
the data sources and maintain high fidelity; and (3) secure distributed
computation for field- and region-level deployment of the DDDAS paradigm with
adaptation and feedback.
2 - Securing Industrial Control Systems with
Software-defined Networking
Dong Jin, Assistant Professor, Illinois Institute of Technology,
10 W 31st Street, Stuart Building 226E, Chicago, IL, 60614,
United States of America,
dong.jin@iit.eduModern industrial control systems (ICSes) are increasingly adopting Internet
technology to boost control efficiency, which unfortunately opens up a new
frontier for cyber-security. With the goal of safely incorporating existing
networking technologies in ICSes, we design a novel software-defined
networking (SDN) architecture for ICSes, with innovative security applications
(e.g., network verification and intrusion detection) and rigorous evaluation using
IIT’s campus microgrid.
3 - A DDDAS Approach to Distributed Control in Computationally
Constrained Environments (UAV Swarms)
Vijay Gupta, Univ. of Notre Dame, 275 Fitzpatrick Hl Engrng,
Notre Dame, IN, 46556, United States of America,
vgupta2@nd.edu, Greg Madey, Daniel Quevedo, Wann-jiun Ma
In modern applications of distributed control, the traditional assumption of ample
processing power at every time step at each agent can be challenged by use of
processor intensive sensors such as cameras. Inspired by the Dynamic Data Driven
Application System approach, we present an algorithm that shifts computational
loads among the agents to guarantee performance in spite of reduced average
processor availability. Analytical results and numerical simulations illustrate the
approach.
TC73
73-Room 203B, CC
Quality Monitoring and Analysis in Complex
Manufacturing Processes
Sponsor: Quality, Statistics and Reliability
Sponsored Session
Chair: Li Zeng, Assistant Professor, Texas A&M University, Industrial
and Systems Engineering, College Station, TX, 77843,
United States of America,
lizeng@tamu.eduCo-Chair: Qiang Zhou, Assistant Professor, City Univ of Hong Kong,
Kowloon, Hong Kong, China,
q.zhou@cityu.edu.hk1 - Monitoring Uniformity of Particle Distributions in Manufacturing
Processes using the K Function
Xiaohu Huang, Graduate Student, City University of Hong Kong,
106B, Hall 8, Student Residence, CityU, Hong Kong, China,
xhhuang6-c@my.cityu.edu.hk,Qiang Zhou
Data in the form of spatial point patterns are frequently encountered in
manufacturing processes. The distributional characteristics of a spatial point
pattern can be summarized by functional profiles like K function. In this study, a
Gaussian process is designed to characterize its behaviour under complete spatial
randomness. A T2 control chart is proposed to monitor the uniformity of point
patterns.
2 - Bayesian Hierarchical Linear Modeling of Profile Data with Apps
to Quallity Control of Nanomanufacturing
Jianguo Wu, Assistant Professor, University of Texas-El Paso, El
Paso, TX, United States of America,
jwu2@utep.edu, Yuhang Liu,
Shiyu Zhou
To achieve a highly automatic quality control, simultaneous profile monitoring
and diagnosis is often required. This paper presents a general framework by using
a hierarchical linear model to connect profiles with both explanatory variables
and intrinsic processing or product parameters for simultaneous monitoring and
diagnosis. The effectiveness is illustrated through numerical studies and
applications to NDE profiles for quality control of nanocomposites manufacturing.
3 - Modeling of Optical Profiles in Low-E Glass Manufacturing
Qian Wu, Graduate Student, Texas A&M University, Industrial
and Systems Engineering, College Station, TX, 77843,
United States of America,
hi.wuqian@gmail.com, Li Zeng
Quality of low-E glass is measured by optical profiles. This study considers
modeling of the optical profile data in Phase I analysis.
4 - Wafer Yield Prediction Based on Virtual
Metrology-generated Parameters
Wan Sik Nam, Seoung Bum Kim/Korea University, Korea
University, 145 Anam-ro, Seongbuk-, Seoul, Korea, Republic of,
wansiknam@korea.ac.krYield prediction is one of the most important issues in semiconductor
manufacturing. Especially, for a fast-changing environment of the semiconductor
industry, accurate and reliable prediction techniques are required. In this study,
we propose a procedure to predict wafer yield using process parameters generated
from the virtual metrology of the semiconductor fabrication, which is based on a
variety of regression and classification algorithms.
TC74
74-Room 204A, CC
Innovative Methods for System Informatics
Sponsor: Quality, Statistics and Reliability
Sponsored Session
Chair: Peihua Qiu, Professor, University of Florida, 2004 Mowry Road,
Gainesville, FL, 32610, United States of America,
pqiu@phhp.ufl.edu1 - When Importance Sampling Meets Stochastic Simulation Models
Eunshin Byon, Assistant Professor, University of Michigan, 1205
Beal Avenue, Ann Arbor, MI, 48109, United States of America,
ebyon@umich.edu, Youngjun Choe, Nan Chen
Importance sampling has been used to improve the efficiency of simulations
where the simulation output is uniquely determined, given a fixed input. We
extend the theory of importance sampling to estimate a system’s reliability with
stochastic simulations where a simulator generates stochastic outputs. Given a
budget constraint on total simulation replications, we derive the optimal
importance sampling density that minimize the variance of an estimator.
2 - QQ Models: Joint Modeling for Quantitative and Qualitative
Quality Responses in Manufacturing System
Ran Jin, Virginia Tech., Grado Department of Industrial and,
Systems Engineering, Blacksburg, VA, 24061, United States of
America,
jran5@vt.edu, Xinwei Deng
A manufacturing system with both quantitative and qualitative (QQ) responses is
widely encountered. The QQ responses are closely associated with each other, but
current methodologies often model them separately. This paper presents a novel
modeling approach, called “QQ models”, to jointly model the QQ responses
through a constrained likelihood estimation. Both simulation studies and a case
study are used to evaluate the performance of the proposed method.
TC74