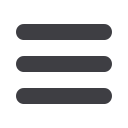

INFORMS Philadelphia – 2015
342
TC69
69-Room 201C, CC
Multimodal Traffic Signal Control in a Connected
Vehicle Environment
Sponsor: TSL/Intelligent Transportation Systems (ITS)
Sponsored Session
Chair: K. Larry Head, University of Arizona, Tucson, AZ,
United States of America,
larry@sie.arizona.edu1 - The Multi Modal Intelligent Traffic Signal Control
System (MMITSS)
K. Larry Head, University of Arizona, Tucson, AZ, United States of
America,
larry@sie.arizona.edu, Yiheng Feng, Mehdi Zamanipour,
Shayan Khoshmagham, Byunho Beak, Sara Khosravi
The Multi Modal Intelligent Traffic Signal Control System (MMITSS) is a Dynamic
Mobility Application for connected vehicles in signalized networks. MMITSS
provides intelligent signal control, priority control for emergency vehicles, transit,
trucks, and pedestrians, and performance observation. MMITSS has been
implemented in the Arizona Connected Vehicle Testbed in Anthem, AZ.
2 - Personalized Signaling for Connected Travelers in a Multi Modal
Traffic Signal System
Sara Khosravi, University of Arizona, Tucson, AZ, United States of
America,
sarakhosravi@email.arizona.edu, Sriharsha Mucheli,
K. Larry Head
Smartphones have become standard equipment for almost all travelers. The
smartphone can be used to provide personalized signaling information for multi
modal travelers including pedestrians and bicycles at signalized intersections,
transit riders, and automobile drivers using navigation applications. This talk will
explore how smartphone applications can impact the transportation system.
3 - Multi-Modal Intelligent Traffic Signal System, Optimal
Priority Control
Mehdi Zamanipour, University of Arizona, Yiheng Feng,
K. Larry Head, Shayan Khoshmagham
A priority control algorithm is presented that simultaneously considers the needs
of different modal users in a Connected Vehicle environment. A mathematical
programming framework that allows multiple priority requests to be considered
simultaneously based on a hierarchical control policy at the intersection level will
be presented.
4 - Real-Time Performance Observation under Connected
Vehicle Technology
Shayan Khoshmagham, University of Arizona, K. Larry Head,
Yiheng Feng, Mehdi Zamanipour
This paper introduces an approach to observe the performance measures of a
multi-modal transportation system in a connected vehicle environment. Different
types of metrics including traffic-based, CV-based and signal-based measures are
observed and estimated by mode by movement. Challenges regarding low market
penetration rate and privacy of the road users are addressed respectively
TC70
70-Room 202A, CC
Predictive Analytics in Railway – Practice
Sponsor: Railway Applications
Sponsored Session
Chair: Dharma Acharya, President, KOSU Services LLC, 241
Auburndale Dr., Ponte Vedra, FL, 32081, United States of America,
acharya.dharma@gmail.com1 - State of Railway Analytics
Dharma Acharya, President, KOSU Services LLC, 241 Auburndale
Dr., Ponte Vedra, FL, 32081, United States of America,
acharya.dharma@gmail.comA brief overview of how the new emerging technology “Analytics” has been
leveraged by railroads will be presented. Potential new areas where railways
might be able to further utilize this new techniques to bring bottom line value to
the company will also be discussed.
2 - Big Data Analytics for Optimized Track Maintenance and
Renewal Management
Luca Ebreo, MERMEC Inc., 110 Queen Parkway, Columbia, NY,
United States of America,
Luca.ebreo@mermecgroup.com,
Pietro Pace
Nowadays, track inspection technology allows railways to collect more and more
data on track’s condition. These data are comparable to “big data” and require
proper analysis in order to extract information for properly managing Track
Maintenance and Renewals. Since railways need to make use as much as possible
of the available data for optimizing their maintenance programs, the required
analytics to support key decisions in an efficient and effective manner will be
illustrated and discussed.
3 - Using Data Visualization to Assess Performance Risk
Eric Pachman, Director, Network Modeling & Analytics, CSX, 500
Water Street, Jacksonville, FL, 32202, United States of America,
Eric_Pachman@csx.comAt CSX, the way we think about “capacity” is changing. By adding data
visualization to traditional industry modeling tools, discussions on capacity are
shifting to discussions on risk and reliability. Our evolution in capacity analysis is
helping CSX better prioritize infrastructure projects to improve network fluidity.
In addition, through data visualization, we can start to “see” how various
operating and commercial requirements and initiatives impact line of road
capacity and risk.
4 - Deploying Predictive Analytics Solutions in the Rail Industry and
Seeing a Return on the Investment
Robert Morris, Chief Science Officer, Predikto, Inc., 1320
Ellsworth Industrial Blvd, Suite A1600, Atlanta, GA, 30318,
United States of America,
Robert@predikto.com,Mario Montag
In this panel, Predikto will provide an overview of automated dynamic predictive
analytics solutions specific to the rail industry. Use cases currently in deployment
across the globe specific to predicting and reducing downtime in freight and
commuter locomotives are discussed alongside the challenges that organizations
face during the process of deploying such technology. Also considered are
strategies to assist in expediting monetary return on investments in predictive
maintenance.
TC71
71-Room 202B, CC
Transportation Planning I
Contributed Session
Chair: Antonio Antunes, Professor, University of Coimbra, Dept. of
Civil Engineering, Coimbra, Portugal,
antunes@dec.uc.pt1 - Plug-in Electric Vehicle Charging Infrastructure Planning using
Cellular Network Data
Jing Dong, Assistant Professor, Iowa State University, 350 Town
Engineering Building, Ames, IA, 50011, United States of America,
jingdong@iastate.edu, Luning Zhang
This paper presents a method to identify activity-travel patterns, in terms of
timing and duration at home, work, and other major destinations, using multiday
cell phone records. A Hidden Markov Model (HMM) is built to link traveler’s
activity transitions to the observed cell tower locations. The probabilistic
parameters of HMM are estimated using the Baum–Welch algorithm. The derived
travel distances and dwell times are key inputs for plug-in electric vehicle
charging infrastructure planning.
2 - The Barriers of Electric Vehicles Spread Adoption in China
Faping Wang, Ph.d Candidate, Tsinghua University, Shenzhen
Graduate School of Tsinghua, Shenzhen, GD, 518100, China,
wfp13@mails.tsinghua.edu.cnThis paper present a survey research about barriers of electric vehicle spread
adoption in China, 1000 questionnaire was designed and send to participants
which come from Beijing, Shanghai, Guangzhou and Shenzhen, all of which are
large city in China. Majority of participants have EVs driving experience or owner
of EV or PHEV. The demographic data was analyzed by statistic methods which
reveal that more different choice behavior exit between western consumer and
Chinese in EVs consumption.
3 - Strategic Infrastructure Development for Alternative Fuel Vehicles
with Routing Considerations
Seong Wook Hwang, PhD Student, The Pennsylvania State
University, 232 Leonhard Building, The Pennsylvania State
University, University Park, PA, 16802, United States of America,
soh5223@psu.edu, Sang Jin Kweon, Jose A. Ventura
This research considers decisions on the siting of alternative fuel (AF) refueling
stations and on the routing of AF vehicles when drivers take a detour to refuel.
Supposing that a driver takes any path whose distance is less than or equals to a
detour distance, we provide an algorithm that finds feasible paths between origins
and destinations. Then, a mathematical model is proposed to determine the
locations of AF refueling stations with the objective of maximizing the covered
flows.
TC69