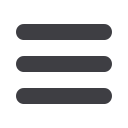

INFORMS Philadelphia – 2015
41
SA14
3 - Approximation Tools for Structured Nonconvex
Optimization Problems
Fatma Kilinc Karzan, Assistant Professor Of Operations Research,
Carnegie Mellon University, 5000 Forbes Avenue, Pittsburgh, PA,
15213, United States of America,
fkilinc@andrew.cmu.edu,
Levent Tuncel
We study the structured nonconvex optimization problem of maximizing a
convex function over a convex domain. Such problems generalize computing
matrix norms and often arise in applications in robust optimization and machine
learning. In many cases, these problems are NP-Hard; and thus we establish a
framework for building tractable convex relaxations. We study various properties,
approximation quality, and exactness of these relaxations, and establish
connections to existing results.
SA12
12-Franklin 2, Marriott
Surrogate-Based and Derivative-Free Optimization I
Sponsor: Optimization/Mixed Integer Nonlinear Optimization and
Global Optimization
Sponsored Session
Chair: Rommel Regis, Saint Joseph’s University, Mathematics
Department, 5600 City Avenue, Philadelphia, 19131,
United States of America,
rregis@sju.edu1 - A Swarm Intelligence Based Data-driven Optimizer using
Adaptive Meta-modeling
Mengqi Hu, University of Illinois at Chicago, Chicao, IL,
United States of America,
mhu@uic.eduAlthough many different meta-models are developed to reduce computational
cost for time-intensive model, it is not conclusive that any meta-model performs
better than others across diverse set of problems. To this end, we develop an
adaptive model selection algorithm to adaptively select the most suited meta-
model for different problems. This approach is integrated with a novel swarm
intelligence algorithm for data-driven optimization which can achieve good result
with less computational cost.
2 - Response Correction Techniques for Multifidelity
Design Optimization
Leifur Leifsson, Iowa State University, Department of Aerospace
Engineering, 2271 Howe Hall, Ames, IA, 50011,
United States of America,
leifur@iastate.eduSimulations are widely used in engineering for analysis and design. A key
challenge is the computational cost. Conventional design techniques typically
require a large number of model evaluations. Thus, the use of simulations in the
design process can be prohibitive. Physics-based surrogates, so-called multifidelity
models, can be used to accelerate the optimization process. This talk describes
several response correction techniques developed specifically for multifidelity
design optimization.
3 - FALCON: A Function Approximation Algorithm for Large-scale
Constrained Black-box Optimization
Rommel Regis, Saint Joseph’s University, Mathematics
Department, 5600 City Avenue, Philadelphia, PA, 19131,
United States of America,
rregis@sju.eduThis talk presents the FALCON algorithm for constrained expensive black-box
optimization that uses surrogates to approximate the objective and constraint
functions. Unlike previous methods, FALCON can handle infeasible start points
and equality constraints. FALCON is implemented using an interpolating radial
basis function surrogate and compared with alternatives on benchmark problems,
including a large-scale automotive application with 124 decision variables and 68
black-box constraints.
SA13
13-Franklin 3, Marriott
Nonconvex Statistical Optimization
Sponsor: Optimization/Optimization Under Uncertainty
Sponsored Session
Chair: Han Liu, Princeton University, Sherrerd Hall, Charlton Street,
Princeton, NJ, United States of America,
hanliu@princeton.edu1 - Blessing of Massive Scale: Spatial Graphical Model Inference
with a Total Cardinality Constraint
Ethan X. Fang, Princeton University, Sherrerd Hall ORFE,
Charlton Street, Princeton, NJ, 08544, United States of America,
ethanfangxy@gmail.com,Han Liu, Mengdi Wang
We propose a novel inferential framework for estimating large-scale spatial
graphical models with a total cardinality constraint. This work has two major
contributions. (i) From a computational perspective, we show that the
computational accuracy increases when the problem scale increases. (ii) From a
statistical perspective, we justify the obtained graph estimator achieves the
minimax optimal rate of convergence under weak assumptions.
2 - A General Theory of Pathwise Coordinate Optimization
Tuo Zhao, Johns Hopkins University, 12124 East Run Drive,
Lawrenceville, NJ, 08648, United States of America,
tourzhao@gmail.com,Tong Zhang, Han Liu
The pathwise coordinate optimization achieves superior empirical performance
for solving high dimensional nonconvex sparse learning problems, but at the
same time poses significant challenge to theoretical analysis. To tackle this long
lasting problem, we develop a new theory showing that the unique algorithmic
structure of the pathwise coordinate optimization plays pivotal roles in
guaranteeing its optimal statistical and computational performance.
SA14
14-Franklin 4, Marriott
Stochastic Optimization with Discrete Moments
Sponsor: Optimization/Optimization Under Uncertainty
Sponsored Session
Chair: Anh Ninh, College of William and Mary, 200 Stadium Dr,
Williamsburg, VA, 23186, United States of America,
ninhtuananh@gmail.com1 - Binomial Moments and Boolean Bounding for Functions in
Random Variables
Jinwook Lee, Drexel University, 3220 Market Street,
Philadelphia, PA, United States of America,
jw78kr@gmail.com,
Jongpil Kim, Andras Prekopa
For a desirable statistical precision level, it is often required to run a simulation
more than million times. A new mathematical model is introduced for sharp
bounds of a function of random variables. For the model construction we use the
binomial moment scheme for systematic mathematical representation, and it’s
further developed by the use of Boolean logic over set algebra. Numerical
examples of various probability distributions are presented.
2 - Improved Bounds on the Probability of the Union of Events Some
of Whose Intersections are Empty
Kunikazu Yoda, Rutgers University,
100 Rockafeller Rd, Piscataway NJ 08854, United States of
America
,kunikazu.yoda@rutgers.edu, Andras Prekopa
We formulate a linear program whose optimal objective function value can be
used in other formulations to yield improved upper and lower bounds on the
probability of the union of events if we know some empty intersections of small
numbers of events. The LP relaxation of an extension of the maximum
independent set problem provides an upper bound on the largest number of
events that have a nonempty intersection. We present numerical experiments
demonstrating the effectiveness of our formulation.
3 - New Methods for Probabilistically Constrained Optimization
Problem with Degenerate Distribution
Olga Myndyuk, Rutgers University, 100 Rockafeller Rd,
Piscataway, NJ, 08854, United States of America,
olik.myn@gmail.comThe problem under consideration is probabilistically constrained optimization
problem with degenerate continuous probability distribution of the random
variables in the constraints.Upper and lower bounds were obtained using the
results of A.Prekopa and degeneracy of the distributions. Existing and new
methods for solving are discussed:supporting hyperplane method, Prekopa-
Vizvari-Badics hybrid algorithm and two derivative-free methods.
4 - Some Aspects of Discrete Moment Problem from the Linear
Programming Perspective: Numerical Approach
Mariya Naumova, Rutgers University, Dept. of Mathematics,
110 Frelinghuysen Rd., Piscataway, NJ, 08854, United States of
America,
mariya.v.naumova@gmail.comWe present a brief survey of some of the basic results related to the discrete
moment problems. We illustrate how piecewise polynomial lower and upper
bounds on the function, created in connection with suitable dual feasible bases in
the univariate discrete moment problem can be used to approximate definite
integrals. Numerical illustrations of valuations of financial instruments are
presented.