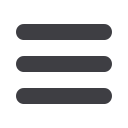
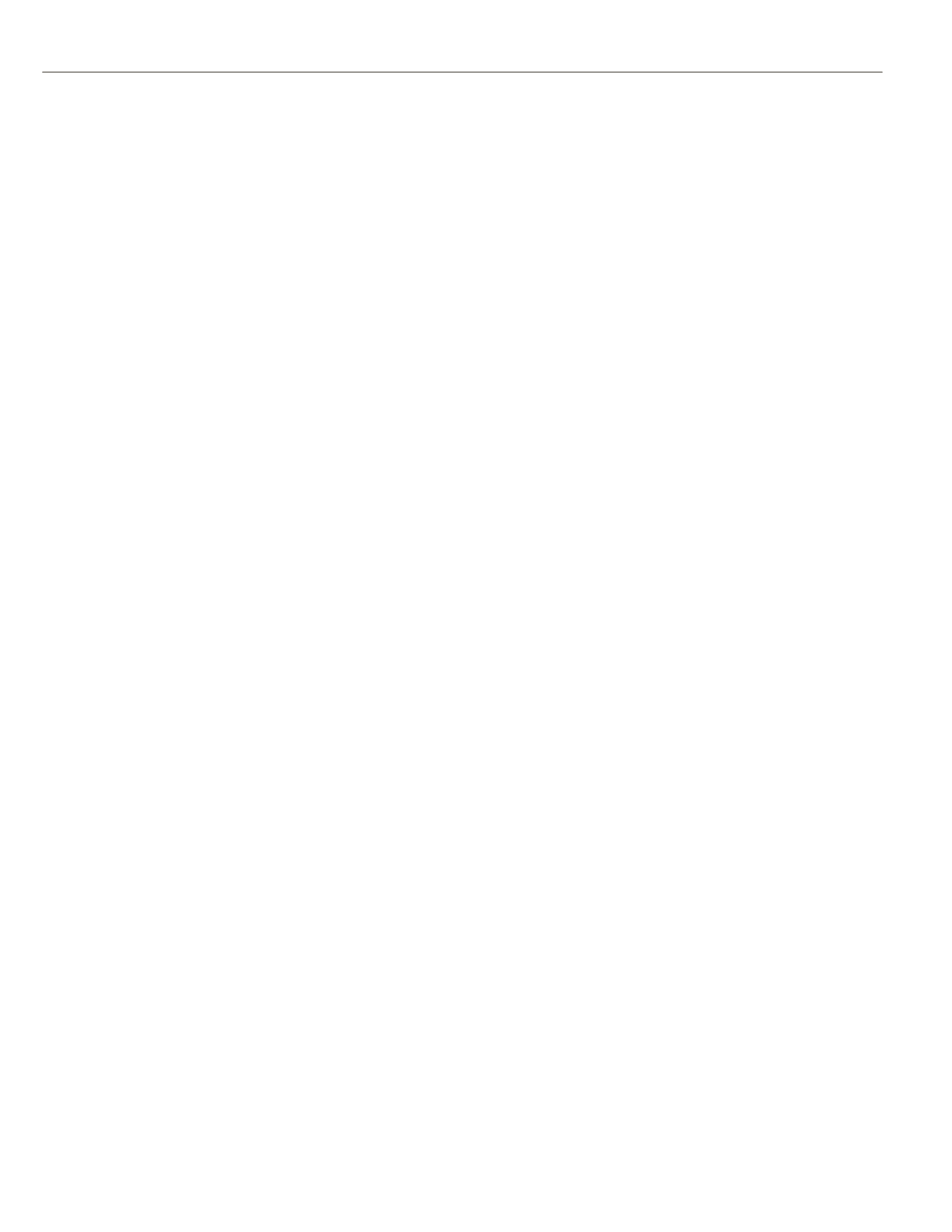
INFORMS Philadelphia – 2015
42
SA15
SA15
15-Franklin 5, Marriott
Nonlinear Optimization in Energy Systems
Sponsor: Optimization/Nonlinear Programming
Sponsored Session
Chair: Nai-Yuan Chiang, Argonne National Laboratory, 9700 South
Cass Avenue, Lemont, IL, United States of America,
nychiang@mcs.anl.govCo-chair: Yankai Cao,Ph.d. Student, Purdue University, FRNY G053B,
480 Stadium Mall Drive, West Lafayette IN 47907, United States of
America,
cao142@purdue.edu1 - Clustering-Based Interior-Point Strategies for Convex
Stochastic Programs
Yankai Cao, PhD Student, Purdue University, FRNY G053B, 480
Stadium Mall Drive, West Lafayette, IN, 47907, United States of
America,
cao142@purdue.edu, Victor M. Zavala, Carl Laird
We present a clustering-based interior-point strategy for two-stage stochastic
programs. The key idea is to perform adaptive clustering of scenarios inside the
solver. The resulting compressed KKT system is much smaller and is used as a
preconditioner. We derive spectral and error properties for the preconditioner. We
also describe our parallel implementation and demonstrate that high compression
rates of 87% and speedups of 30 are achievable for electricity market clearing
problems.
2 - Arc Search Methods for Linearly Constrained Optimization
Nick Henderson, Research Associate and Instructor, Stanford
University, Stanford, CA,
nwh@stanford.eduWe present an arc search algorithm for linearly constrained optimization. The
method constructs and searches along smooth arcs that satisfy a small set of
properties. When second derivatives are used, the method is shown to converge
to a second-order critical point. We discuss use of arc search in Quasi-Newton
methods and different strategies for handling constraints.
3 - A Progressive Method to Solve Large-scale AC Optimal Power
Flow with Discrete Variables
Maxime Fender, Optimization Consultant, Artelys Canada Inc.,
2001 Boulevard Robert-Bourassa, #1700, Montréal, QC, H3A
2A6, Canada,
maxime.fender@artelys.com, Manuel Ruiz,
Jean Maeght, Alexandre Marié, Patrick Panciatici
This study on power system networks aims to produce a dynamic simulation
based security assessment taking into account uncertainties. An extended OPF
without any guarantee on feasibility leads to the resolution of a Mixed-Integer
NonLinear Problem, very challenging, and even harder to solve when the
problem is not convex. A custom filtering method which tries to explain
infeasibilities and uses the nonlinear solver KNITRO to reformulate discrete
variables into nonlinear constraints is proposed.
4 - A Robust Approach to Chance Constrained Optimal Power Flow
with Renewable Generation
Yury Dvorkin, PhD Student/Research Assistant, University of
Washington, 185 Stevens Way NE, Paul Allen Center, Room
AE104R, Seattle, WA, 98195, United States of America,
iouridvorkin@gmail.com, Miles Lubin, Scott Backhaus
We formulate a Robust Chance Constrained (RCC) OPF that accounts for
uncertainty in the parameters of these probability distributions by allowing them
to be within an uncertainty set. The RCC OPF is solved using a scalable cutting-
plane algorithm. We evaluate the RRC OPF on a modified BPA test system, which
includes 2209 buses and 176 controllable generators. Deterministic, chance
constrained (CC), and RCC OPF formulations are compared using several cost and
reliability metrics.
SA16
16-Franklin 6, Marriott
Topics in Optimization
Sponsor: Optimization/Linear and Conic Optimization
Sponsored Session
Chair: John Mitchell, Professor, Rensselaer Polytechnic Institute,
Mathematical Sciences Dept, Troy, NY, 12180, United States of America,
mitchj@rpi.edu1 - A Rounding Procedure for a Maximally Complementary Solution
of Semidefinite Optimization Problems
Ali Mohammad Nezhad, PhD Student, Lehigh University, 200
West Packer Ave., Industrial and Systems Engineering Dept.,
Bethlehem, PA, 18015, United States of America,
ali.mohammadnezhad@gmail.com,Tamás Terlaky
In this paper, we deal with the identification of optimal partitioning in
semidefinite optimization. We derive some bounds on the condition numbers of
the problem using the first order theory of reals and estimate the magnitude of
the eigenvalues in the vicinity of the central path, which depends on the degree
of singularity of the optimality conditions. We then present a rounding procedure
for the solution of an interior point method to get a maximally complementary
solution.
2 - Convex and Structured Nonconvex Stochastic Optimization with
Stochastic Constraints
Zhiqiang Zhou, University of Florida, 2330 SW Williston RD,
Apt3034, Gainesville, FL, 32608, United States of America,
brianzhou1991@gmail.com,Guanghui Lan
We present a new stochastic approximation (SA) algorithm to minimize a class of
convex or nonconvex objective functions subject to certain expectation
constraints. We show that this algorithm exhibits the optimal rates of
convergence in expectation and with high probability under different conditions.
Some numerical results are provided for portfolio management and machine
learning.
3 - Benders Decomposition for Discrete-constrained Problems with
Complementarity Constraints
John Mitchell, Professor, Rensselaer Polytechnic Institute,
Mathematical Sciences Dept, Troy, NY, 12180, United States of
America,
mitchj@rpi.edu,Jong-shi Pang, Andreas Waechter,
Francisco Jara-Moroni
We discuss a logical Benders decomposition approach to discrete-constrained
mathematical programs with complementarity constraints. This is an extension of
our prior approach to linear and quadratic programs with complementarity
constraints. The inclusion of discrete and binary constraints broadens the
applicability of the approach.
SA17
17-Franklin 7, Marriott
Social Network Modeling and Optimization
Sponsor: Optimization/Network Optimization
Sponsored Session
Chair: Alexander Nikolaev, Assistant Professor, University at Buffalo
(SUNY), 312 Bell Hall, Buffalo, NY, 14260-2050,
United States of America,
anikolae@buffalo.edu1 - Seed Selection Scheduling for Long-term Campaign Planning on
Large Social Networks
Mohammadreza Samadi, PhD Candidate, University at Buffalo
SUNY, 327 Bell Hall, Buffalo, NY, 14260, United States of
America,
msamadi@buffalo.edu,Alexander Nikolaev,
Nagi Rakesh
The influence maximization problem lies in finding a set of seeds that can
optimally initiate a diffusion-driven cascade. We explore flexible, time-dependent
seed activation solutions for long-term intervention/campaign planning on
networks. The Seed Selection Scheduling Problem (SSSP) is that of selecting an
optimal policy for seed activation over a finite time horizon under knapsack
constraints. The ideas from the wireless sensor scheduling domain are used to
tackle SSSP on large networks.
2 - Critical Nodes in Network Cohesion
Alexander Veremyev, University of Florida, 1350 N Poquito Road,
Shalimar, FL, United States of America,
averemyev@ufl.edu,
Oleg Prokopyev, Eduardo Pasiliao
We consider a class of critical nodes detection problems that involves
minimization of a graph cohesion measure (e.g., graph efficiency or harmonic
average geodesic distance, Harary index, characteristic path length,
communication utility) that depends on the actual pairwise distances between
nodes in the remaining graph after nodes removal. We derive linear integer
programming formulations along with additional enhancements, and develop an
exact iterative algorithm to solve this problem.
3 - Detecting Cliques of Maximum and Minimum Centrality: Methods
and Applications
Chrysafis Vogiatzis, Assistant Professor, North Dakota State
University, 1410 14th Avenue North, Room 202 Civil & Industrial
Engineering, Fargo, ND, 58102, United States of America,
chvogiat@ufl.edu, Alexander Veremyev
In this talk, we consider the problem of finding the most and least “influential” or
“influenceable” cliques in graphs based on three classical centrality measures:
degree, closeness, and betweenness. In addition to standard betweenness, we also
consider its optimistic and pessimistic counterparts along with a new metric for
cluster closeness, namely residual closeness centrality. Applications discussed
include analysis of information and social networks, and results on the stock
market graph.