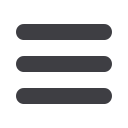

INFORMS Philadelphia – 2015
48
SA32
3 - Balanced Tree Partitioning with Compact Mathematical
Programming Formulations
Onur Seref, Virginia Tech, 2060 Pamplin Hall (0235), Blacksburg,
VA, 24061, United States of America,
seref@vt.edu, J. Paul Brooks
In this paper, we study mathematical programming formulations for the balanced
subtree partitioning problem, some variations of which are known to be NP-
complete. We introduce compact exact mixed integer programming formulations
for different variations of the problem. We explore the effect of different sets of
constraints on our formulations and provide comparative computational results
among our formulations as well as other exact formulations and approximation
algorithms.
SA32
32-Room 409, Marriott
Data Mining in Medical and Brain Informatics
Sponsor: Data Mining
Sponsored Session
Chair: Sina Khanmohammadi, SUNY Binghamton, 4400 Vestal
Parkway East, Binghamton, NY, 13902, United States of America,
skhanmo1@binghamton.eduCo-Chair: Chou-An Chou, Binghamton University, 4400 Vestal
Parkway, Vestal, NY, United States of America,
cachou@binghamton.edu1 - Nonlinear Dimensionality Reduction for Analysis of
Electroencephalography Records
Anton Kocheturov, Research Assistant, Department of Industrial
and Systems Engineering, University of Florida, 303 Weil Hall,
Gainesville, FL, 32611-6595, United States of America,
antrubler@gmail.comWe suggest using nonlinear dimensionality reduction technique called the Local
Linear Embedding for analysis of EEG records. This approach enabled us to
distinguish between different states of the brain in a more efficient way
comparing to the existing machine learning techniques since it is faster and
doesn’t require training of the algorithm. We also detected evidence for local
linearity of the brain in the resting state and introduced a new model of the brain
based on it.
2 - The Gap Statistics for Determining the Number of Linear
Autoregressive Modes in a Multimode Model
Vahid Tarokh, Professor, Harvard University, 33 Oxford Street,
MD347, Cambridge, MA, 02138, United States of America,
vahid@seas.harvard.edu, Jie Ding, Mohammad Noshad
We consider modeling of non-stationary stochastic-processes using a multi-mode
linear autoregressive (AR) model. Each process is modeled as a mixture of
unknown AR components (modes) with an unknown number of modes. We are
interested in online determination of the number of modes, and also in
identification of each mode. A new model selection approach based on GAP
statistics for this purpose is introduced with applications in modeling and
prediction of financial and biological data, etc.
3 - Online Machine Learning Framework for Detecting
EEG Abnormalities
Sina Khanmohammadi, SUNY Binghamton, 4400 Vestal Parkway
East, Binghamton, NY, 13902, United States of America,
skhanmo1@binghamton.edu, Chou-An Chou
Electroencephalogram (EEG) signals provide insight about human brain function.
EEG signal abnormalities can be a sign of mental disorders such as depression,
epilepsy, etc. Hence, detecting EEG abnormalities can be beneficial for diagnosis,
prognosis, and overall improvement of patient’s quality of life. In this work, we
present a new online classification framework to detect EEG signal abnormalities
in real time. The proposed framework is validated using public EEG datasets.
SA33
33-Room 410, Marriott
Health Policy/ Public Health
Sponsor: Health Applications
Sponsored Session
Chair: Ozge Karanfil, PhD Candidate, MIT Sloan School of
Management, 100 Main Street, E62-379, Cambridge, MA, 02142,
United States of America,
karanfil@mit.edu1 - Multi-Criteria, Multi-Place, Multi-Time: Evaluating System
Strategies with the Rethink Health Model
Jack Homer, Owner, Homer Consulting, 72 Station Hill Rd.,
Barrytown, NY, 12507, United States of America,
jhomer609@gmail.com,Gary Hirsch, Bobby Milstein
The ReThink Health Dynamics Model simulates many possible interventions to
improve a local health system by lowering costs and deaths and boosting equity
and productivity through 2040. It has been carefully calibrated to represent
several localities across the U.S. A multi-criteria weighting scheme reflecting local
values helps to select a single “best” intervention strategy. The best strategy may
also depend upon a locality’s room for improvement with regard to health care
and health risks.
2 - Systems Models of Post-traumatic Stress Disorder
Navid Ghaffarzadegan, Professor, Virginia Tech, 231 Durham Hall,
1185 Perry Street, Blacksburg, VA, 24060,
United States of America,
navidg@vt.edu, Richard Larson
Little agreement exists about effective methods of screening for PTSD, optimal
cutoff values, and even the number of PTSD patients. In a series of modeling
efforts, we uncover five vicious cycles that inhibit PTSD treatment, and
investigate characteristics of an optimal cutoff value for screening. We then show
a population level model of PTSD patients in a military/post-military system. The
models represent different levels of complexities of PTSD at individual and
societal levels.
3 - Patient Flows in Mental Health Clinics
Anne Claire Collin, Graduate Student, MIT, 235 Albany Street,
Ashdown House #5052, Cambridge, MA, 02139,
United States of America,
acollin@mit.eduThere are different factors that can hinder access and quality of care for veterans
in a mental health clinic, many of which are not related to the treatment itself.
The purpose of this research is to analyse the flows of patients in Veterans Affairs’
Mental Health clinics in order to improve access. After a thorough work of
understanding patients’ conditions and paths through the different services,
simulation is used to find a configuration which improves wait times.
4 - Cost Escalation in Health Systems Dominated by
Private Health Insurance
Nisa Onsel, Research Assistant, Bogazici University, Bogazici
University, Industrial Engineering Dept, Bebek, Istanbul, 34342,
Turkey,
nisa.guler@boun.edu.tr, Yaman Barlas, Günenç Yöcel
Adverse selection and moral hazard are effective in individuals’ health insurance
plan choice and healthcare service utilization. The inefficient behaviors have a
potential impact on increasing insurance premiums. In the existence of increasing
healthcare costs due to advanced health technologies, cost escalation in health
systems is a complex problem. A system dynamics model is constructed and
analyzed for understanding financial sustainability of health systems dominated
by private insurance.
SA34
34-Room 411, Marriott
Health-care Decision Making
Sponsor: Health Applications
Sponsored Session
Chair: Ebru Bish, Associate Professor, Virginia Tech, Dept of Industrial
and Systems Eng, 250 Durham Hall, Blacksburg, VA, 24061-0118,
United States of America,
ebru@vt.edu1 - The Management of Mass Casualty Incident Response
Behrooz Kamali, PhD Candidate, Virginia Tech, 250 Durham Hall
(MC 0118), Blacksburg, VA, 24061, United States of America,
kamali@vt.edu,Douglas Bish
In this research we seek to systematically investigate triage and transport of
casualties in the aftermath of a mass-casualty incident. We study the structure of
the optimal policies using novel models that incorporate available resources,
quantity, and mix of casualties. Insights gained allow us to derive special cases
that can be solved to optimality with simple heuristics. We compare results from
our models to that of other models in the literature and the current practices.
2 - A Study on the Spatial Spread and Optimal Control of the 2014-
2015 Ebola Outbreak in West Africa
Esra Buyuktahtakin, Assistant Professor, Wichita State University,
1845 N Fairmount, Wichita, KS, 67260, Wichita, United States of
America,
Esra.Buyuktahtakin@wichita.edu, Eyyub Kibis,
Emmanuel Des-bordes
We develop an optimization approach to capture the disease dynamics of the
deadly Ebola virus. We illustrate our model on a case study from Guinea, Liberia,
and Sierra Leone. Numerical results demonstrate the accuracy of our predictions
and suggest that the model can be used as a decision-making tool to optimally
allocate resources for epidemic prevention and control.