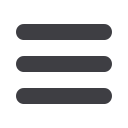
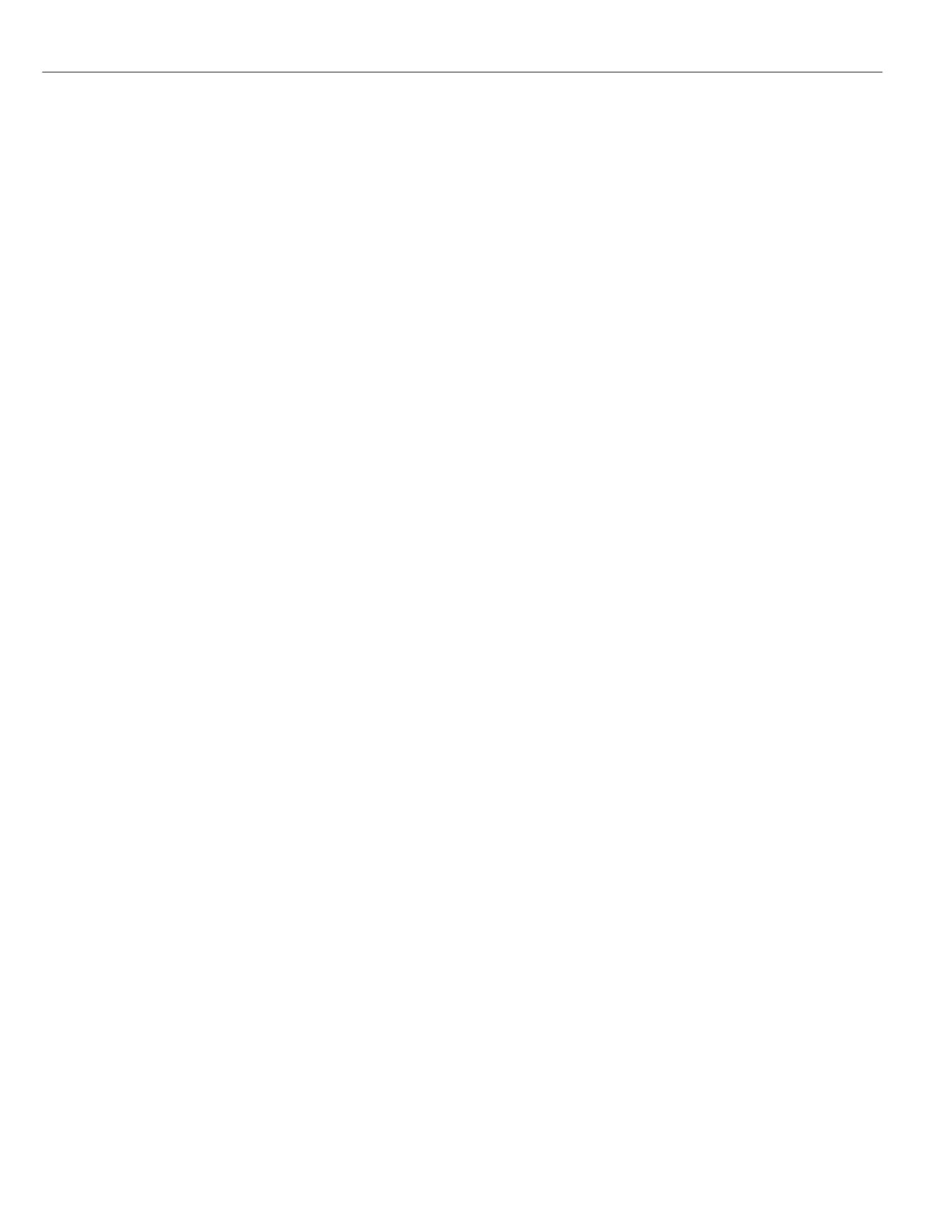
INFORMS Philadelphia – 2015
50
SA37
SA37
37-Room 414, Marriott
Health Care Modeling and Optimization I
Contributed Session
Chair: Michal Jakubczyk, Warsaw School of Economics, Al.
Niepodleglosci 162, Warsaw, 02-554, Poland,
michal.jakubczyk@sgh.waw.pl1 - The Optimal Timing of Medical Tests
Thomas Weber, Associate Professor, EPFL, CDM-ODY 3.01,
Station 5, Lausanne, VD, 1015, Switzerland,
thomas.weber@epfl.chThis paper considers the optimal timing of tests based on a known law of motion
for the statistical evolution of a random population prevalence. In a Bayesian
setting, we find for a given imperfect binary disease diagnostic and action
thresholds the optimal time to test and retest a potentially ill individual,
conditional on past test outcomes. The framework allows for complex disease
dynamics, including multiple populations, contagion, and stochastic lifetimes.
2 - A Fuzzy Approach to Modeling the Willingness-to-Pay for Health
and Supporting Decision Making
Michal Jakubczyk, Warsaw School of Economics, Al.
Niepodleglosci 162, Warsaw, 02-554, Poland,
michal.jakubczyk@sgh.waw.plChoosing between health technologies involves multiple criteria (e.g., effects &
cost), uncertainty, and often multiple alternatives. I advocate that, due to
peculiarity of health, fuzziness additionally needs to be introduced to model the
willingness-to-pay/accept (WTP/WTA). I show how to do that by redefining
notions typically used in health technology assessment. Properties of new
approach are discussed. Accounting for fuzziness additionally explains the WTP-
WTA disparity in this context.
3 - Emergency Department Length-of-stay Estimation using Time-
variant Predictors
Seung Yup Lee, Graduate Research Assistant, Wayne State
University, 4815 4th St., Detroit, MI, 48202, United States of
America,
seung.lee@wayne.edu, Ratna Babu Chinnam, Alper
Murat, Evrim Dalkiran
The accurate length-of-stay (LOS) estimation for patients in emergency
departments (ED) is a pre-requisite for quality resource coordination between ED
and inpatient wards. We investigate how time-variant levels of crowding in ED
can be captured and incorporated in LOS estimation models by using vector
autoregression (VAR). We will also report results and insights from testing the
models on data from VA Medical Centers.
4 - An Integrated Framework to Model the Trajectories of
Chronic Conditions
Adel Alaeddini, University of Texas at San Antonio (UTSA),
One UTSA Circle, San Antonio, United States of America,
adel.alaeddini@utsa.eduAny medical condition that requires long term monitoring and management to
control symptoms and shape the course of the disease is known as chronic
conditions. Nearly 45% of the general population has 1 chronic condition or
more. This accounts for more than 75% percent of health care expenditures. We
present an integrated probabilistic framework for modeling the trajectories of
chronic conditions. The proposed methodology will be validated using a large
dataset from a medical center in Texas.
SA38
38-Room 415, Marriott
Big Data I
Contributed Session
Chair: Ellick Chan, Exponent, 149 Commonwealth Dr., Menlo Park,
CA, 94025, United States of America,
echan@exponent.com1 - A Structural Service Model for Describing and Designing
Services with Data
Chie-Hyeon Lim, Post-doc, POSTECH, Engineering Building
#4-316, Pohang, 790-784, Korea, Republic of,
arachon@postech.ac.kr,Min-Jun Kim, Kwang-jae Kim,
Paul Maglio
Using big data effectively in service design requires having a model that describes
the service in question along with the data in use. In this talk, we propose a
generic structural service model to describe a service with a set of predefined
variables, facilitating design of services that use big data. The variables include
service objective, indicators, customer and context variables, and delivery
contents. We discuss the model in the context of several case studies of service
design.
2 - Increasing Productivity and Minimizing Errors in
Spreadsheet Analytics
Larry LeBlanc, Professor, Owen Graduate School of Management,
Vanderbilt University, 401 21st Avenue South,
Nashville, TN, 37203, United States of America,
larry.leblanc@owen.vanderbilt.edu,Thomas Grossman,
Michael Bartolacci
Spreadsheets have proliferated for business analytics, and spreadsheet errors can
result in poor supply chain, manufacturing, or investment decisions, including the
failure to identify good opportunities. We examine potential problem areas for
spreadsheet design and suggest alternative design approaches that seek to increase
productivity and reduce the likelihood of errors. Even careful analysts might send
their spreadsheet to assistants for updating, and s/he might need these guidelines
3 - A Practical Big Data Precision Marketing – Cross-Selling Mobile
Bank to Internet Bank
Jian Xu, IBM, Diamond Bld, ZGC Software Park, Beijing, China,
xujianx@cn.ibm.com, Ming Xie, Yuhang Liu, Zhen Huang,
Tianzhi Zhao, Yuhui Fu
The bank wants improve mobile bank users and transform customers from online
bank channel to mobile bank. Mobile bank represents the future E-channel. Large
amount of data is integrated and analyzed on E-channel users’ behavior. The
users’ online behaviors are also considered. We build the cross-selling model to
identify the potential customers who are more likely to become mobile bank
users, and improve the marketing success rate significantly.
4 - Forecasting Unemployment Rate by using Ensemble Hybrid
Ann- Bayesian Model Combination
Farzad Radmehr, West Virginia University, 900 Willowdale Road,
Morgantown, WV, United States of America,
fradmehr@mix.wvu.eduThe goal of this paper is to predict the future data by using ensemble Bayesian
model. Our dataset is UK unemployment rate from Floros C. paper in
2005(Floros, 2005). In this paper, the Bayesian Ensemble Model Combination
(BMC) will be proposed. For this purpose, we run ANN multiple times and these
results will be the initial values for BMC. Then by giving the weight to each value,
we predict the new value. The goal is to compare the values in BMC and ANN.
5 - Deep Learning Approaches to Digging Data Out of Digitized
Paper Documents
Ellick Chan, Exponent, 149 Commonwealth Dr., Menlo Park, CA,
94025, United States of America,
echan@exponent.com,Glen Depalma
Many organizations scan paper documents for fast search, however, existing
search approaches generally require carefully crafted search terms to find
documents. In this talk, we discuss deep learning approaches for OCR and search.
We use computer vision to improve OCR accuracy and apply deep learning using
Google’s Word2Vec natural language processing (NLP) to identify topics of interest
automatically. We’ve processed more than 300 boxes of documents with our
techniques.
SA39
39-Room 100, CC
Game Theoretic Models in Operations and
Marketing Interface
Cluster: Operations/Marketing Interface
Invited Session
Chair: Tao Li, Santa Clara University, 500 El Camino Real, Santa Clara,
CA, 95053, United States of America,
tli1@scu.edu1 - Online Manufacturer Referral to Heterogeneous Retailers
Gangshu Cai, Santa Clara University, OMIS Department, Lucas
Hall 216N, Santa Clara, CA, 95053, United States of America,
gcai@scu.edu, Hao Wu, Chwen Sheu, Jian Chen
Since the development of the Internet, thousands of manufacturers have been
referring consumers visiting their websites to some or all of their retailers.
Through a model with one manufacturer and two heterogeneous retailers, we
investigate whether it is an equilibrium for the manufacturer to refer consumers
exclusively to a retailer or nonexclusively to both retailers.
2 - Strategic Risk Management in Spot Market for Supply Chains
under Competition
Xuan Zhao, Associate Professor, Wilfrid Laurier University, 75
University Avenue West, Waterloo, ON, Waterloo, Canada,
xzhao@wlu.ca,Shanshan Ma, Wei Xing
This paper studies two risk management strategies related to spot market to
mitigate firms’ exposure to demand uncertainty, namely, operational hedging and
financial hedging. We provide insights on the dynamics of each hedging strategy
under competition.