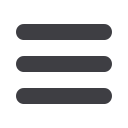
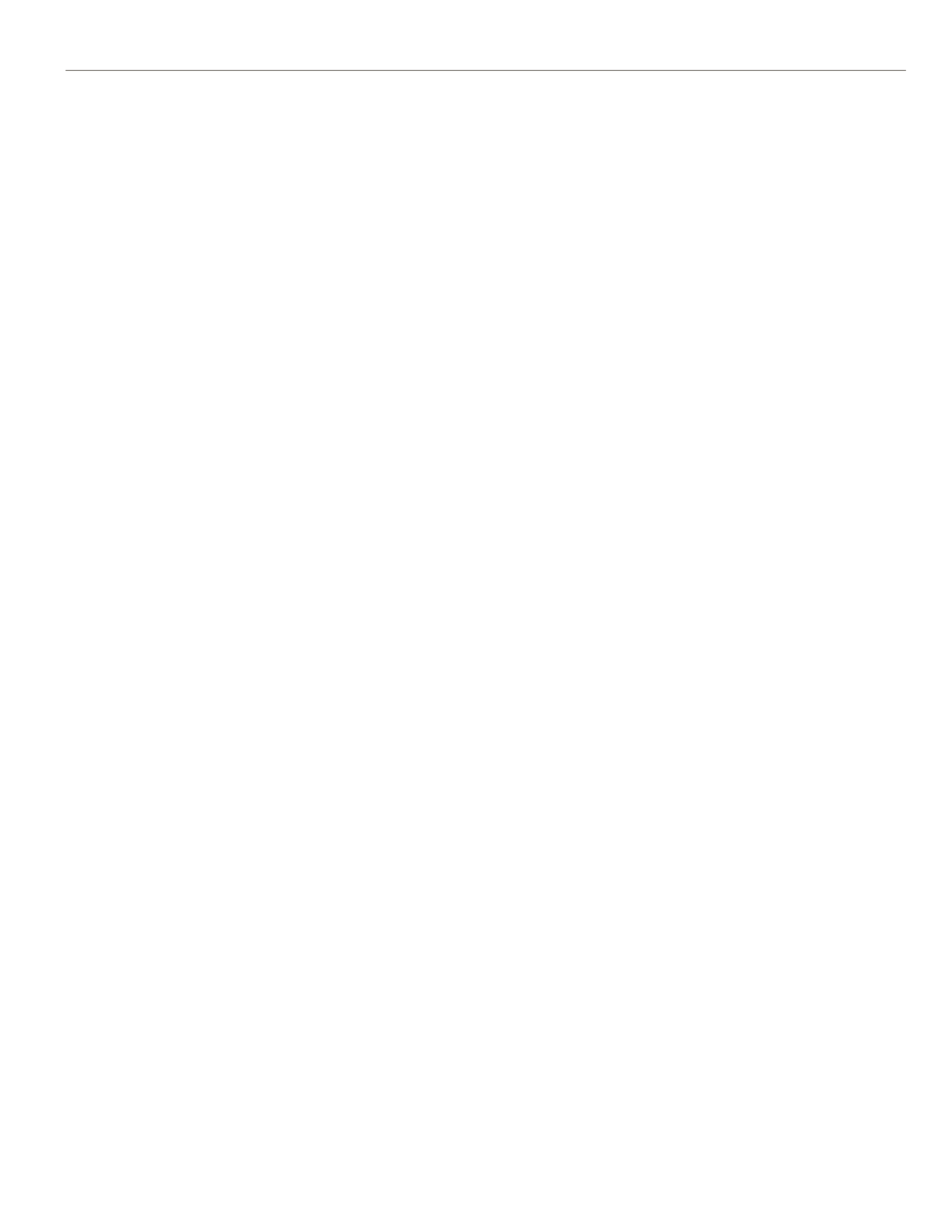
INFORMS Philadelphia – 2015
45
SA25
SA23
23-Franklin 13, Marriott
Queueing Models
Sponsor: Applied Probability
Sponsored Session
Chair: Alexander Stolyar, Lehigh University, 200 W. Packer Ave, Room
484, Bethlehem, United States of America,
als714@lehigh.edu1 - Diffusion Approximations for Large-scale Buffered Systems
Tonghoon Suk, Georgia Institute of Technology, 755 Ferst Drive,
NW, Atlanta, GA, 30305, United States of America,
tonghoon.suk@gmail.comMotivated by a desire to design stochastic systems satisfying certain quality of
service, we establish mean-field limit theorems to approximate systems. We start
with a simple model consisting of n buffers and a server, and develop diffusion
approximations for the system with the randomized LQF scheduling algorithm.
We achieve this by allowing the number of sampled buffers d = d(n) to depend on
the number of buffers n, which yields an asymptotic decoupling of the queue
length processes.
2 - Stability Verification: A Monte-Carlo Approach
Neil Walton, University of Amsterdam, Science Park 904,
Amsterdam, Netherlands,
n.s.walton@uva.nl, Brendan Patch,
Michel Mandjes
We implement a form of simulated-annealing as a method for detecting if a
queueing system is unstable for a given set of loads.
3 - A Large-Scale Service System with Packing Constraints:
Greedy-Random Algorithm with Sub-Linear Error
Alexander Stolyar, Lehigh University, 200 W. Packer Ave, Room
484, Bethlehem, PA, United States of America,
als714@lehigh.edu,Yuan Zhong
There are multiple input flows of different customer types and infinite number of
servers. Each server may simultaneously serve multiple customers, subject to
general “packing constraints”. Each customer leaves after an independent random
service time. We consider a version of a Greedy-Random customer assignment
(packing) algorithm, and prove that it has an asymptotic competitive ratio 1, as
the input flow rates grow to infinity.
4 - Heavy-traffic Behavior of the Maxweight Algorithm in a Switch
with Non-uniform Traffic
R. Srikant, University of Illinois Urbana-Champaign, CSL 107,
Urbana, IL, United States of America,
rsrikant@illinois.edu,
Siva Theja Maguluri
We consider a switch operating under the MaxWeight scheduling algorithm. The
traffic pattern is assumed to be such that all ports are saturated, but otherwise can
be non-uniform. It is shown that the heavy-traffic scaled queue length under
MaxWeight is within a factor of two compared to the smallest achievable queue
length.
SA24
24-Room 401, Marriott
Data Mining for Decision Making
Sponsor: Artificial Intelligence
Sponsored Session
Chair: Iljoo Kim, Assistant Professor, Saint Josephís University,
347 Mandeville Hall, 5600 City Avenue, Philadelphia, PA, 19131,
United States of America,
ikim@sju.edu1 - Rating Corporate Bonds using Deanfis Analysis –
A Muli-modeling Approach
Rashmi Malhotra, Associate Professor, Saint Joseph’s University,
5600 City Avenue, Philadelphia, PA, 19131, United States of
America,
rmalhotr@sju.edu,Davinder Malhotra
This paper illustrates the use of data envelopment analysis (DEA) and Adaptive
Neuro-Fuzzy Inference Systems (ANFIS) to differentiate between bonds with
credit ratings. This study measures the relative performance of corporate bonds
using DEA. The ANFIS model creates a rule-based system that can aid the
decision-maker in making decisions regarding the implications of a decision. This
study proposes a modeling technique that jointly uses the two techniques to
benefit from the two methodologies.
2 - Mining Hidden Organizational Structures from Meeting Records
Jiexun Li, Assistant Professor, Oregon State University, College of
Business, Corvallis, OR, 97331, United States of America,
jiexun.li@oregonstate.edu,Zhaohui Wu, Bin Zhu
Organizations often contain complex structures formed by social relationships.
This study introduces an approach to finding hidden structures by mining
meeting records. Using text-mining techniques, we extract information about
persons and their opinions on topics. We conducted cluster analysis and network
analysis to uncover hidden structures within the organization. Our preliminary
study shows promising results. We are in the process of improving our approach
and conducting more analyses.
3 - An Analytical Framework for Intelligent
Reviewer Recommendation
Harry Wang, Association Professor, University of Delaware, 42
Amstel Ave, Newark, DE, 19716, United States of America,
hjwang@udel.edu, Kunpeng Zhang, Sean Kilgallon
Recruiting reviewers for academic conferences and journals is a daunting task for
conference organizers and journal editors. In this paper, we propose an intelligent
approach for reviewer identification based on techniques such as text mining,
social network analysis, and recommender system. We collect data from online
paper repositories and research social network sites. We evaluate our approach by
conducting user studies and experiments.
4 - Semi-supervised Article Selection for Medical Systematic
Reviews
Jun Liu, Assistant Professor, Dakota State University, 422 SW 8th
Street, Apt. 16, Madison, SD, 57042, United States of America,
jun.liu@dsu.edu, Prem Timsina, Omar El-gayar
We developed a semi-supervised learning based classifier to identify articles that
can be included in of medical systematic reviews. Through an empirical study, we
demonstrated that semi-supervised approach is a viable technique for selecting
articles for systematic reviews when only a few number of training samples are
available, and a combination of semi-supervised and active learning can further
optimize the article selection process.
SA25
25-Room 402, Marriott
Business Analytics and Innovation
Sponsor: Information Systems
Sponsored Session
Chair: Yan Huang, Assistant Professor, Stephen M. Ross School of
Business, University of Michigan, 701 Tappan St. R5322, Ann Arbor,
MI, 48109, United States of America,
yphuang@umich.edu1 - Who Hath the Crystal Ball? Antecedents of Advanced Analytics
Adoption in Firms
Ajit Sharma, Ross School of Business, 701 Tappan Street, Ann
Arbor, MI, United States of America,
asharmaz@umich.edu,Mayuram Krishnan, Yan Huang
It is increasingly evident that the ability to gain forward-looking insight from
advanced analytics will differentiate the winners from the losers. However, it is
not clear what differentiates firms that are able to leverage these new
technologies from those that do not. In this paper we attempt to answer this
question by empirically investigating the antecedents of predictive analytics usage
within firms. We present our findings and discuss managerial implications.
2 - Gain from Loss: Crowdsourcing Contests Bring Favorable
Superstar Effect
Shunyuan Zhang, Tepper School of Business, Carnegie Mellon
University, 5000 Forbes Avenue, Pittsburgh, PA, 15213, United
States of America,
shunyuaz@andrew.cmu.edu, Param Vir Singh,
Anindya Ghose
We report favorable superstar effect, which contradicts with adverse superstar
effect in Economic literature, and argue the unique crowdsourcing setting is the
cause. Competing with superstars decreases one’s chance of winning but leads to
an improved performance in the next competition. Contestants are self-selected
to solve the trade-off problem. Our work suggests a promising crowdsourcing
practice that generates spill-over effects and identifies participants who are more
capable of learning.