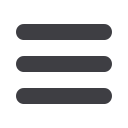

INFORMS Philadelphia – 2015
455
2 - Data Science Approach for Dealership Performance Analysis
Haidar Almohri, Wayne State University, Detroit, MI, 48243,
United States of America,
almohri@wayne.edu, Mark Colosimo,
Ratna Babu Chinnam
Due to the dynamics and complexity of the automotive market, managing
automotive dealership performance has always been a challenge for business
owners. We present a novel, data science approach for managing, assessing, and
enhancing dealership performance using data from dealerships across the United
States by first clustering the dealers. Results suggest that this new approach
surpasses the traditional approach employed by the industry.
3 - Overview of Supply Chain Risk Management and the Current
Issues of Closed-loop Supply Chain in China
Qiang Qiang, Pennsylvania State University, 30 E. Swedesford
Rd., Malvern, PA, 19335, United States of America,
qzq10@psu.eduThe path toward sustainability to demonstrate environmental and social
responsibility has led to an increasing attention to the lifecycle of a product with a
focus on value-added recovery activities. However, besides the risks encountered
in traditional supply chains (or, sometimes referred to as the forward supply
chain), the CLSC bears additional layer of risks if the products are not properly
recycled. In this talk, we will provide an overview of both supply chain and CLSC
risks in China.
4 - Supply Chain Risk
James Ang, Research Advisor, The Logistics Institute Asia Pacific,
National University of Singapore, #04-01 21 Heng Mui Keng
Terrace, Singapore, 119613, Singapore,
bizangsk@nus.edu.sgSupply chain risk analysis involves risk identification assessment. For the former,
the failure mode effect analysis is improved using fuzzy theory and grey system
theory. For the latter, simulation models of supply chains are built to
quantitatively assess impacts of risks in terms of production rate, inventory level,
and lead-time.
WD05
05-Room 305, Marriott
The Social Impacts of Social Media Analysis
Cluster: Social Media Analytics
Invited Session
Chair: Les Servi, The MITRE Corporation, 202 Burlington Road,
Bedford, MA, United States of America,
lservi@mitre.org1 - Modeling Human Behavior in the Context of Social Media During
Extreme Events
Yulia Tyshchuk, Researcher, USMA, 5 Winding Brook Dr.
Apt. 2J, Guilderland, NY, 12084, United States of America,
yulia.tyshchuk@gmail.comThis research examines how social science theory, Theory of Planned Behavior,
can explain human behavior in response to extreme events on social media.
Validation of this theory enables emergency response officials to create strategies
that facilitate public response to extreme events such as diffusion of critical
actionable information, providing confirmations, and taking the prescribed action.
Effective public response can save lives and reduce property damage.
2 - Social Media Whispers during IPO Quiet Period
Julie Zhang, Assistant Professor, University of Massachusetts
Lowell, One University Ave., Lowell, MA, 01854,
United States of America,
juheng_zhang@uml.eduWe investigate social media news for companies during their quiet periods for
IPO. In this study, we examine whether the stock price of a company on its IPO
day is associated with the volume and valence of its social media coverage during
quiet period. We demonstrate the relationship between a company’s stock price
PARK volatility in the first week of IPO and its social media coverage in the first
week and quiet period.
3 - Simulating Twitter Ego Networks
Inbal Yahav, Bar Ilan University, Bar Ilan University,
Ramat Gan, 5290002, Israel,
yoavac@gmail.com,Yoav Achiam
The network on the 2-level (followers, and their followers) Twitter social ego-
network is studied. A simulation based on A. Barabasi’s model is generated. The
collected data contains the followers’ names and a count of their followers. We
found that followers’ distribution follows a combination of power law and
exponential distributions. The distribution of followers per companies (having
more than 1000 followers) is steady over time, with a constant rate of new
followers and departing followers
WD06
06-Room 306, Marriott
Portfolio Analysis I
Contributed Session
Chair: Umit Saglam, Assistant Professor, East Tennessee State
University, Department of Management and Marketing,
College of Business and Technology, Johnson City, TN, 37614,
United States of America,
saglam@etsu.edu1 - Understanding Behaviors of Robust Portfolios
Woo Chang Kim, Associate Professor, KAIST, 291 Daehak-Ro,
Yuseong-Gu, Daejeon, Korea, Republic of,
wkim@kaist.ac.krRobust portfolio optimization has been developed to resolve the high sensitivity
to inputs of the Markowitz mean–variance model. Although much effort has been
put into forming robust portfolios, there have not been many attempts to analyze
the characteristics of portfolios formed from robust optimization. In this
presentation, we discuss the recent findings on the qualitative characteristics of
the robust portfolios such as higher moment controllability, factor tilting
behaviors, and robustness.
2 - Computing Near-optimal Value-at-risk Portfolios using Integer
Programming Techniques
Onur Babat, PhD Student, Lehigh University, 217 W. Packer Ave,
Bethlehem, PA, 18015, United States of America,
onur.babat@lehigh.edu, Juan Vera, Luis Zuluaga
VaR is a non-convex risk measure. It is well-known that the VaR portfolio
problem can be formulated as an integer program (IP), which can be difficult to
solve with current IP solvers for large-scale instances of the problem. To tackle
this drawback, we present an algorithm to compute near-optimal VaR portfolios
that takes advantage this IP formulation and provides a guarantee of the near-
optimality of the solution. Numerical results will be presented.
3 - Portfolio Optimization with Probabilistic Ratio Constraints
Ran Ji, PhD Candidate, The George Washington University,
2201 G St, NW, Funger 415H, Washington, DC, 20052, United
States of America,
jiran@gwmail.gwu.edu, Miguel Lejeune
We propose a class of stochastic portfolio optimization models with probabilistic
ratio constraints. The proposed probabilistic reward-risk ratio measures regard the
asset returns/losses as random variables to mitigate the estimation risk due to
mean return vectors. Each model includes a chance constraint with random
technology matrix. We expand a combinatorial modeling framework to represent
the feasible set of the chance constraint as a set of mixed-integer linear
inequalities.
4 - A Marginal Conditional Stochastic Dominance Based Model of
Enhanced Index Tracking
Qian Li, Professor, Xi’an Jiaotong University, No.74 West Yanta
Road, Xi’an, China,
lqian@mail.xjtu.edu.cn,Liang Bao
Stochastic dominance and mean-variance are two criteria used in optimal
portfolio selection. In this paper, we present an enhanced model for index
tracking with Marginal Conditional Stochastic Dominance (MCSD) rules. The
model is still in the optimization framework but combined with two levels of
MCSD criteria. By adopting an immunity based multiple objective optimization
algorithm, the solutions for the model are developed. The model is then applied
to 8 major markets.
5 - Revealed Preferences for Portfolio Selection –
Does Skewness Matter?
Umit Saglam, Assistant Professor, East Tennessee State University,
Department of Management and Marketing, College of Business
and Technology, Johnson City, TN, 37614,
United States of America,
saglam@etsu.edu, Merrill Liechty
In this study, we consider two competing descriptions of portfolio selection, the
traditional mean variance efficient portfolio versus a generalization allowing for
decision makers to consider skewness in their asset allocation. Our numerical
experiments are conducted on portfolio drawn from 30 different stocks from the
Dow Jones. Numerical results show that investors’ preferences are better
explained when skewness is taken into account.
WD06