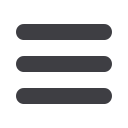

INFORMS Philadelphia – 2015
457
4 - Managing Cross-border E-commerce: A Multichannel
Integration Approach
Shikui Wu, Assistant Professor, Ryerson University, 350 Victoria
St., TRS 2-015, Toronto, Canada,
shikui.wu@ryerson.ca,
Yuanyuan Wu
This study adopts a multichannel integration approach to analyze and optimize
online and offline business strategies and operations for cross-border e-commerce,
including: pricing and inventory control; sales, deliveries and returns; and, online
and in-store customer service. It helps cross-border businesses in adapting their
strategies to market environment, streamlining their business processes, reducing
operational costs, mitigating market uncertainty, and improving customer
satisfaction.
WD11
11-Franklin 1, Marriott
Optimization Integer Programming III
Contributed Session
Chair: Carlos Eduardo De Andrade, Senior Inventive Scientist, AT&T
Labs Research, 200 Laurel Avenue South, A5-1E33, Middletwon, NJ,
07748, United States of America,
cea@research.att.com1 - Cardinality Constrained Portfolio Optimization via Mixed Integer
Linear Programming
Nasim Dehghan Hardoroudi, Aalto University School of Business,
Runeberginkatu 22-24, Helsinki, N/, 00100, Finland,
nasim.dehghan@gmail.com,Abolfazl Keshvari
Controlling the number of active assets (cardinality of the portfolio) in a mean-
variance portfolio problem is practically important but computationally difficult.
Such task is commonly presented as a mixed integer quadratic programming
(MIQP) problem. We propose a novel approach to reformulate such problem as a
MILP problem. Our proposed formulation can be solved by standard MILP solvers
much faster than the MIQP problem.
2 - On Linear Conic Relaxation of Discrete Quadratic Programming
Tiantian Nie, North Carolina State University, Department of
Industrial and Systems, Engineering, Raleigh, NC, 27695, United
States of America,
tnie@ncsu.edu,Shu-cherng Fang, Qi An
Discrete quadratic programming problems (DQP) appear widely in real-life
applications, but they are hard to solve. We proposed an RLT-based linear conic
relaxation of DQP. When the proposed relaxation problem has an optimal
solution with rank one or two, optimal solutions to the original DQP problem can
be explicitly generated. Numerical results indicate that the proposed relaxation
with a primal ADM procedure is capable of efficiently providing high-quality
lower bounds for DQP.
3 - Location Problem of Electric Vehicles’ Charge Stations
under Uncertainty
Rui Chen, IE Department of Tsinghua University,
ShunDe Building in Tsinghua University, Beijing, China,
chenruiest@163.comThe location of charging stations spreads adoption of electric vehicles by
consumer and it simultaneously affects the cost and revenue. This paper presents
a location problem for electric vehicles’ charge stations under fluctuation of
electricity price. An integer programming model is proposed to solve this problem
with uncertainty of demand. Then a hybrid algorithm is applied to and
numerically verified by an actual example.
4 - A Learning Framework for Feasibility Pump
Carlos Eduardo De Andrade, Senior Inventive Scientist, AT&T
Labs Research, 200 Laurel Avenue South, A5-1E33, Middletwon,
NJ, 07748, United States of America,
cea@research.att.com,
Shabbir Ahmed, Yufen Shao, George L. Nemhauser
We present a framework for finding feasible solutions for mixed integer programs.
We use the feasibility pump heuristic (FP) coupled with a learning framework
which is able to build a pool of projections and combine them using information
of previous projections. Preliminary results shows that this approach is able to
find feasible solutions for instances where the original FP fails.
WD12
12-Franklin 2, Marriott
Optimization Stochastic IV
Contributed Session
Chair: Jeremy Castaing, University of Michigan, IOE 1205 Beal Ave.,
Ann Arbor, MI, United States of America,
jctg@umich.edu1 - Application of Two-stage Risk-averse Optimization to Real-time
Interday Portfolio Management
Sitki Gulten, Assistant Professor, Stockton University, 101 Vera
King Farris Drive, Galloway, NJ, United States of America,
sitki.gulten@stockton.edu, Andrzej Ruszczynski
We describe a study of application of risk-averse optimization techniques to daily
portfolio management. First, we develop clustering methods for scenario tree
construction. Then, we construct a two-stage stochastic programming problem
with conditional measures of risk, which is used to re-balance the portfolio on a
rolling horizon basis, with transaction costs included. Finally, we present an
extensive simulation study on both intraday and interday real-world data of the
methodology.
2 - Stochastic Mixed Integer and Gradient Search Methods for
Constrained Problems
Larry Fenn, Hunter College, CUNY, 695 Park Avenue, New York,
NY, 10021, United States of America,
larry.fenn@gmail.com,Felisa Vazquez-abad
An ordinal variable b denotes resources. For each b, there is a control variable u
that yields a convex cost C(b,u). We seek minimal cost satisfying a constraint of
the form P(b,u) < a. Both functions are steady state averages of a stationary
complex process: function evaluations are very costly. We combine Fibonacci
search in b with gradient search in u to find the optimal solution and propose
sequential sampling for minimal computation. We analyze convergence and
discuss parallel computation.
3 - Optimally Scheduling Satellite Communications under Uncertainty
Jeremy Castaing, University of Michigan, IOE 1205 Beal Ave.,
Ann Arbor, MI, United States of America,
jctg@umich.edu,
Amy Cohn, James Cutler
We consider the problem of scheduling and managing the download of data from
collecting satellites to receiving ground stations under uncertainty of their
availability. We design models and heuristics to compute download schedules
over the planning horizon while addressing dynamics of collecting, storing, using,
and spilling both data and energy.
WD13
13-Franklin 3, Marriott
Stochastic Programming
Sponsor: Optimization/Optimization Under Uncertainty
Sponsored Session
Chair: Guzin Bayraksan, Associate Professor, The Ohio State University,
Integrated Systems Engineering, Columbus, OH, 43209,
United States of America,
bayraksan.1@osu.edu1 - Regularized Decomposition of High–dimensional Multistage
Stochastic Programs with Markov Uncertainty
Tsvetan Asamov, Princeton University, Sherrerd Hall,
Charlton Street, Princeton, NJ, 08544, United States of America,
tasamov@princeton.edu,Warren Powell
We develop a quadratic regularization approach for the solution of
high–dimensional multistage stochastic optimization problems. The resulting
algorithms are shown to converge to an optimal policy after a finite number of
iterations. Computational experiments are conducted using the setting of
optimizing energy storage over a large transmission grid. The numerical results
indicate that the proposed methods exhibit significantly faster convergence than
their classical counterparts.
2 - Multistage Stochastic Optimization with Application in Energy
Storage Control
Harsha Gangammanavar, University of Southern California,
3715, McClintock Avenue, GER 240, Los Angeles, CA, 90089,
United States of America,
gangamma@usc.edu, Suvrajeet Sen
In this talk we will present Time-staged Stochastic Decomposition, a sequential
sampling algorithm which is applicable to multistage stochastic linear programs,
in general, and control of distributed storage devices, in particular. The method is
focused on stage independent uncertainty and its special cases, including
autoregressive processes. We will present convergence properties of this
algorithm, and computational results comparing it with algorithms like
approximate dynamic programming.
WD13