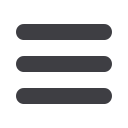

INFORMS Philadelphia – 2015
461
3 - A Storage Grid as a Network of “Internet of Things”
Ehsan Shirazi, West Virginia University, Morgantown, WV, 26505,
United States of America,
ehshirazi@mix.wvu.eduThis work focuses on a high-density storage system in which each grid works as
an agent. Combining the decentralized, agent-based control with supervisory
oversight provides a higher level of efficiency of product movement within the
storage grid. Metrics in the research focus on variations from theoretical number
of movements.
4 - Framework of Multiple Stochastic Decision Process in a System
Toshikazu Aiyama, Professor, Tokyo Metropolitan University,
1-1 Minami Ohsawa, HachiOhji, Japan,
tyaiyama@yahoo.comConsider multiple stochastic decision process operating in a system. A system is
open to its environment; thus allowing external variability. We will concentrate
on a two-process system on this research. First we will present various types. Next
we will analyze some characteristics of each types. Some elementary numerical
results are presented to illustrate the implication of more than one process in a
system.
WD23
23-Franklin 13, Marriott
Queueing Approximation and Simulation
Sponsor: Applied Probability
Sponsored Session
Chair: John Hasenbein, Mechanical Engineering, University of Texas at
Austin, Austin, TX, United States of America,
jhas@mail.utexas.edu1 - Exact Simulation of Non-stationary Queues
Mohammad Mousavi, Assistant Professor, University of
Pittsburgh, 1048 Benedum Hall, Pittsburgh, PA, 15212,
United States of America,
mousavi@pitt.eduWe discuss the challenges that arise in the planning simulations of systems with
time dependent arrival and service rates. Estimating how far back in time a
simulation must be initialized is an essential problem in planning simulations. We
propound using reflected Brownian motion (RBM) with time-dependent drift and
volatility as a guide for estimating this initialization time. We develop an exact
simulation method for RBM with time-dependent drift and volatility.
2 - Heavy-traffic Limits for a Fork-join Network in the
Halfin – Whitt Regime
Hognyuan Lu, Penn State University, 355 Leonhard Bldg,
University Park, PA, 16802, United States of America,
hzl142@psu.edu,Guodong Pang
We study a multi-server fork-join network with non-exchangeable
synchronization (NES), where all parallel service stations are operating in the
Halfin-Whitt regime under the non-idling FCFS discipline. The NES requires that
completed tasks are only synchronized if they are associated with the same job.
We prove FWLLN and FCLT for the number of tasks in each waiting buffer for
synchronization, jointly with the number of tasks in each parallel service station
and the number of synchronized jobs.
3 - Optimal Stock Allocation for Production-inventory Systems with
Multiple Impatient Customer Classes
Yasar Levent Kocaga, Assistant Professor Of Operations
Management, Yeshiva University, 500 West 185th Street, New
York, NY, 10033, United States of America,
kocaga@yu.edu,
Yen-Ming Lee
We address the production and inventory control of a make-to-stock system with
multiple impatient customer classes. We assume Poisson demand and exponential
production times. Demand not satisfied immediately is backordered; but waits
only up to an exponentially distributed amount of time, and is cancelled if not
satisfied within this time. We show that the threshold inventory rationing policy
is still optimal under certain conditions including a requirement on the order of
abandonment rates.
4 - Routing and Scheduling in Fluid Gurvich Networks
John Hasenbein, Mechanical Engineering, University of Texas at
Austin, Austin, TX, United States of America,
jhas@mail.utexas.edu, Arda Sisbot
We examine a class of networks in which fluids may be routed to different classes
at the same server. Extending previous work on single-station systems, we show
that optimal policies in tandem Gurvich networks can exhibit interesting counter-
intuitive behavior.
WD24
24-Room 401, Marriott
Artificial Intelligence I
Contributed Session
Chair: Stanislaus Solomon, Assistant Professor Of Supply Chain
Management, Sam Houston State University, 236S SHB,
Department of Management and Marketing, Huntsville, TX, 77340,
United States of America,
solomon@shsu.edu1 - Active Learning for Relevance Vector Machine Regression
Youngdoo Son, PhD Candidate, Seoul National University,
1 Gwankak-ro, Gwanak-gu, Seoul, Korea, Republic of,
hand02@snu.ac.kr, Jaewook Lee
In this paper, we propose a novel active learning procedure for relevance vector
machine regression. First, we propose the transductive relevance vector machine
which uses both labeled and unlabeled data points to construct the model. Then,
we suggest three simple querying strategies which exploit the characteristic of
relevance vector machine regression. The proposed method showed significantly
better performance than the benchmark, random selections, with both of artificial
and real data sets.
2 - Rethinking Principal Component Analysis in EEG Classification
Xiaoxia Li, North Dakota State University, 124 East Bison Court,
Fargo, ND, 58102, United States of America,
xiaoxia.li@ndsu.eduPrincipal Component Analysis (PCA) is considered to be a powerful tool in
dimension reduction. However, it is worth thinking of the suitability of
application for EEG signal data. Two EEG datasets collected from alcoholic and
control groups were used to test the prediction accuracy before and after PCA
transformation with SVM and KNN methods. Based on the classification results,
we found that PCA is not valid in EEG signal processing. We also concern that
other factors might be confounding.
3 - Regret Transfer and Parameter Optimization
Noam Brown, Carnegie Mellon University, 5000 Forbes Ave,
Pittsburgh, PA, 15213, United States of America,
noamb@andrew.cmu.eduRegret matching is a widely-used algorithm for learning how to act. We begin by
proving that regrets on actions in one game can be transferred and discounted to
warm start the regrets for solving a different game with same structure but
different payoffs that are a function of parameters. This provides, to our
knowledge, the first principled warm-starting method for no-regret learning. We
then leverage this warm starting to optimize a parameter vector for a player in a
two-player zero-sum game.
4 - Dynamic Programming Approximations for Stochastic Resource
Planning under Partial Observation
Stanislaus Solomon, Assistant Professor Of Supply Chain
Management, Sam Houston State University, 236S SHB,
Department of Management and Marketing, Huntsville, TX,
77340, United States of America,
solomon@shsu.edu,
Cipriano Santos, Haitao Li, Keith Womer
Assigning heterogeneous resources to jobs in a stochastic environment requires a
sequential decision process. This research focuses on developing an approximate
dynamic programming algorithm to address the problem scenario, where the
information required to make decisions at each epoch is only partially observable.
Hewlett-Packard’s resource planning problem will be studied as an application.
WD25
25-Room 402, Marriott
Joint Session AI/ICS: AI Planning and Operations
Research
Sponsor: Artificial Intelligence
Sponsored Session
Chair: Chris Beck, University of Toronto, 5 King’s College Rd,
University of Toronto, Toronto, ON, M5S3G8, Canada,
jcb@mie.utoronto.ca1 - Heuristic Search in Dual Space for Constrained Stochastic
Shortest Path Problems
Sylvie Thiebaux, ANU & NICTA, 7, London Circuit, Canberra,
Australia,
Sylvie.Thiebaux@anu.edu.au,Felipe Trevizan,
Pedro Santana, Brian Williams
Constrained Stochastic Shortest Path Problems are a natural model for planning
under uncertainty for resource-bounded agents with multiple competing
objectives. We present i-dual, a heuristic search algorithm that generates optimal
stochastic policies for these problems. It incrementally generates and explores
promising regions of the ‘dual’ space of policy occupation measures, guided by a
lower bound on the value function. Our experiments show significant run-time
improvements over LP methods.
WD25