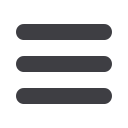

INFORMS Philadelphia – 2015
458
3 - Dynamic Allocations for Cooperative Games under Uncertainty
with Risk-averse Players
Nelson Uhan, Assistant Professor, United States Naval Academy,
Mathematics Department, Chauvenet Hall, Annapolis, MD,
21402, United States of America,
uhan@usna.edu, Alejandro
Toriello
We consider a class of cooperative games in which the costs of cooperation are
uncertain and evolve over time, and the players are risk averse. These games
generalize the classic linear production game, and as a result, model a variety of
cooperative settings. We give sufficient conditions for the existence of an
allocation in the strong sequential core - the set of allocations that distribute costs
as they are incurred and are stable against coalitional defections at any point in
time.
4 - Ambiguous Stochastic Programs with Variation Distance
Hamed Rahimian, PhD Student, The Ohio State University,
Integrated Systems Engineering, Columbus, OH, 43210,
United States of America,
rahimian.1@osu.edu, Guzin Bayraksan,
Tito Homem-de-mello
Ambiguous stochastic programs relax the assumption of known distributions in
stochastic programming and instead use an ambiguity set of distributions. We
focus on the variation distance to form the ambiguity set, examine the resulting
model properties, and propose a decomposition-based algorithm to solve it. We
characterize a minimal scenario tree, where the presence of every scenario is
critical in determining the optimal objective function.
WD14
14-Franklin 4, Marriott
Data Driven Optimization and Applications I
Sponsor: Optimization/Optimization Under Uncertainty
Sponsored Session
Chair: Michael Kim, University of Toronto, 5 King’s College Road,
Toronto, Canada,
mikekim@mie.utoronto.ca1 - Robust Optimization with Learning
Andrew Lim, National University of Singapore/Department of
Decision Sciences, Mochtar Riady Building, BIZ1 08-69, 15 Kent
Ridge Drive, Singapore, Singapore,
andrewlim@nus.edu.sg,
Michael Kim
We consider a robust optimization problem with learning in the setting of a
Bayesian mixture model and show how it is a natural framework for modeling
customer heterogeneity in business analytics applications. Asymptotic equivalence
to a mean-variance problem is established. More generally, we illustrate how
robust Bayesian models are a natural framework for combining concerns for
robustness and learning about uncertainty sets.
2 - Coordinating Pricing and Inventory Replenishment with
Nonparametric Demand Learning
Boxiao (Beryl) Chen, University of Michigan-Ann Arbor, 1205
Beal Avenue, Ann Arbor, MI, 48109, United States of America,
boxchen@umich.edu, Xiuli Chao, Hyun-Soo Ahn
We consider a finite horizon problem of dynamic pricing and inventory control
for a nonperishable product. We develop a data-driven policy that does not
require explicit information about the demand distribution and show that it is
asymptotically optimal and converges at the fastest possible speed.
3 - Data-driven Assortment Optimization
Velibor Misic, Massachusetts Institute of Technology,
77 Massachusetts Avenue, E40-147, Cambridge, MA, 02139,
United States of America,
vvmisic@mit.edu, Dimitris Bertsimas
We present a practical method for transforming limited historical transaction data
into effective assortment decisions. Our method consists of estimating a choice
model from the data by efficiently solving a large-scale linear program, and
finding the optimal assortment by solving a tractable mixed-integer program. Our
method leads to assortments that achieve near-optimal revenues and significantly
outperform assortments derived from other parametric and nonparametric
approaches.
4 - Geo-demand Estimation and Inventory Allocation in
Online Retailing
Long He, University of California, Berkeley, 1117 Etcheverry Hall,
Berkeley, CA, 94720, United States of America,
longhe@berkeley.edu, Zhiwei (Tony) Qin
For existing items, we first formulate the multi-dimensional geo-demand
distribution estimation problem for existing items as a robust low-rank tensor
recovery problem in a convex optimization framework. We also propose a tailored
algorithm based on the alternating direction augmented Lagrangian method. For
new items, we develop the estimation based on nested logit model and discuss the
inventory allocation policy in practice.
WD15
15-Franklin 5, Marriott
Optimization Methodology I
Contributed Session
Chair: David Valembois, Head Of Business Development, ENGIE,
Avenue Enstein, 2a, Louvain-la-Neuve, 1300, Belgium,
david.valembois@external.engie.com1 - Globally Solving Non-Convex Quadratic Programming Problems
via Linear Integer Programming Techniques
Wei Xia, Lehigh University, 312 Brodhead Ave., Bethlehem, PA,
18015, United States of America,
wex213@lehigh.edu,
Luis Zuluaga, Juan Vera
Quadratic programming (QP) problems are NP-hard optimization problems. We
propose an alternative way of globally solving nonconvex QPs by exploiting of the
Karush-Kuhn-Tucker conditions to linearize its objective. Then we reformulate
the problem as a mixed integer linear problem with binary variables to model the
KKT complementary constraints. We compare the performance of this solution
approach with the benchmark global QP solver QuadprogBB on a variety of test
QP instances.
2 - An Engie Novel Approach to the Solution of Thermal Unit
Commitment Problem with Coupling Constraints
Melodie Mouffe, ENGIE, Avenue Einstein, 2A, NewTech Center,
Louvain-la-Neuve, 1348, Belgium,
melodie.mouffe@engie.com,
Dimitri Tomanos
Engie developed PoweredPegase to optimize the commitment of its large number
of plants on a medium term horizon, while taking into account supply delivery,
storage and ancillary services. Getting to find a solution using a fine time
granularity is difficult to achieve, specifically when dealing with global
constraints. We propose a novel approach to find an accurate solution in a
reasonable amount of time, without performing any model approximation,
implementing a “smart” time decomposition.
3 - Poweredpegase : Engie’s Next-gen Software for Joint Gas and
Power Portfolio MT Optimization
David Valembois, Head Of Business Development, ENGIE,
Avenue Enstein, 2a, Louvain-la-Neuve, 1300, Belgium,
david.valembois@external.engie.comPoweredPegase is ENGIE’s next-gen software for joint gas & power portfolio MT
optimization. PP delivers reliable and accurate solutions in remarkable short time
thanks to disruptive “smart time” approach. PP open “working modules” allow
modifying models by integrating your specific context. If flexibility and accuracy
really matter, PoweredPegase is the answer. ENGIE (GDF-Suez) is the No. 1
Independent Energy Producer in the World. Winter is Coming–Optimize Your
Energy with PoweredPegase.
WD16
16-Franklin 6, Marriott
Game Theory V
Contributed Session
Shuo Zeng,University of Arizona, McClelland Hall 430,
1130 E. Helen Street, Tucson AZ 85721, United States of America,
shuozeng@email.arizona.edu1 - Strategic Delay in Networked Bargaining
Thanh Nguyen, Krannert School of Management, Purdue
University, West Lafayette, IN, United States of America,
nguye161@purdue.edu, Vijay Subramanian, Randall Berry
We study decentralized markets involving producers and consumers that are
facilitated by middlemen. We do this by analyzing a non-cooperative networked
bargaining game. We show that sunk cost problems and a heterogeneous network
can give rise to delay or failure in negotiation, and therefore, reduce the total
trade capacity of the network. In the limiting regime of extremely patient agents,
we provide a sharp characterization of the trade pattern and the segmentation of
these markets.
2 - A Joint Work on Knowledge Management and Operations
Management: How to Keep Firm-Specific Knowledge
Kai Luo, Assistant Professor, KEDGE Business School,
450 Avenue François Arago CS 90262, La Garde, France,
luokailk@sohu.com, Salomee Ruel, Sajjad Jasimuddin
Firm-specific knowledge (FSK) acquisition and maintenance is essential for a firm
to fill knowledge gaps and enhance its competitive advantage. We start with
qualitative research that identifies the existence and important features of the
FSK problem, followed by quantitative research formulated as a principal-agent
problem, providing closed-form solutions. We used our model to compare French
and American firm styles via 40 numerical examples and develop managerial
insights.
WD14