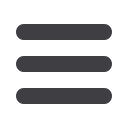

S483
ESTRO 36
_______________________________________________________________________________________________
Subsequently, the 2D slices were binned in 10 equidistant
bins according to the 1D diaphragm position (amplitude
binning). To account for outliers, we developed a strategy
that sets the inclusion range such that 95% of the
diaphragm positions are included, while the peak-to-peak
range is minimized (denoted Min95). We compared this
with two frequently used strategies (Fig.1): one that
selects the maximum inhale and exhale position as range
(MaxIE), not discarding outliers, and one that selects the
mean inhale and exhale position as inclusion range
(MeanIE).
The strategies were evaluated based on the following
parameters:
•
* Data included (DI); the fraction of data used
for reconstruction after exclusion of outliers.
•
* Reconstruction completeness (RC); the
fraction of the 110 (11 slices x 10 bins)
bin/slice combinations in the 4D data set that
are filled.
•
* Intra-bin variation (IBV); the standard error of
the mean diaphragm position inside a bin/slice
combination.
•
* Peak-to-peak range (PP);
•
* Image smoothness (S); assessed by quantifying
how well a parabola fits the diaphragm shape
in a sagittal plane of the reconstructed 4DMRI,
per bin (S = R
2
adj
averaged over all bins). S
ranges from 0 (discontinuous diaphragm shape;
artefacts) to 1 (smooth shape; no artefacts).
A low DI indicates underestimation of motion amplitude.
A low IBV indicates high binning precision. Low RC, low S
and high IBV result in image artefacts, e.g. discontinuities
between reconstructed slices.
A paired Wilcoxon’s signed rank test was used to test
differences in parameters between binning strategies.
Results
Excluding only 5% of images during amplitude binning, the
developed Min95 strategy outperformed the MaxIE
strategy with a 9.5% higher mean RC, 5.6 mm lower mean
PP and virtually the same mean IBV and S (all significant,
Table 1).
The MeanIE strategy with a mean DI of 76.4%, severely
underestimated the motion amplitude even though it had
a higher S, higher RC and lower IBV compared to MaxIE.
The Min95 strategy outperformed the MeanIE strategy with
an 18.6% higher mean DI.
Conclusion
Our novel binnin g strategy for 4DMRI outperformed the
classical strategies, resulting in a 4DMRI with h igh
precision and fewer artefacts in the presence of irregular
breathing.
PO-0882 Proxy-free slow-pitch helical 4DCT
reconstruction
R. Werner
1
, C. Hofmann
2
, T. Gauer
3
1
University Medical Center Hamburg-Eppendorf,
Department of Computational Neuroscience, Hamburg,
Germany
2
Siemens Healthcare, Imaging & Therapy Systems,
Forchheim, Germany
3
University Medical Center Hamburg-Eppendorf,
Department of Radiotherapy and Radio-Oncology,
Hamburg, Germany
Purpose or Objective
Standard 4DCT protocols correlate external breathing
signals (exploiting e.g. surface tracking devices or
abdominal belts) to raw or reconstructed image data to
allow for reconstruction of a series of CT volumes at
different breathing phases. From a radiotherapy (RT)
workflow perspective, dealing with external devices for
breathing signal recording is cumbersome. Moreover, if
the respiratory signal is corrupted, 4DCT reconstruction is
not possible at all. At this, proxy-free reconstruction – i.e.
4DCT reconstruction without using an external breathing
signal – could improve RT workflows. We present a novel
approach for slow-pitch helical 4DCT reconstruction and
illustrate its feasibility.
Material and Methods
Similar to standard external breathing signal-driven slow-
pitch helical CT we assume a sufficiently low pitch and
gantry rotation time to be given to ensure existence of
appropriate raw data for reconstruction of image data at
each z position and desired breathing phase. We then
pursue a three-step process: (1) image-based derivation of
a differential breathing signal; (2) correlation of the
extracted breathing signal to raw data; and (3) integration
and minimization of an artifact-metric into the final (here:
phase-based) reconstruction process. For the crucial step
(1), we initially reconstruct slices at a series of z-positions
and points in time and determine (slice wise, averaged
over a specific region of interest) the change Δ
torso
/Δt in
chest wall height. As Δ
torso
/Δt can be considered as
derivation of the desired breathing signal (figure 1); its
zero-crossings represent the end-inspiration (and the end-
expiration) breathing phases to be correlated to the raw
data.
Feasibility of the afore-mentioned approach is
investigated using routinely acquired 4DCT lung and liver
data sets. A detailed analysis of motion dynamics and
image artifacts is performed in proxy-free reconstructed
4DCT data sets of three patients and resulting numbers are
compared to corresponding standard external breathing
curve-driven phase-based (PB) reconstructions based on
the same 4DCT raw data plus RPM breathing signal.