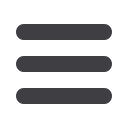

S489
ESTRO 36
_______________________________________________________________________________________________
decreasing pre- or mid-therapy
K
1
spatial heterogeneity,
higher but decreasing pre- or mid-therapy overall
V
b
parameter value, and lower pre-therapy
V
b
spatial
heterogeneity.
Figure shows selected results of Kaplan-Meier analyses
that illustrates prognostic power of some imaging
biomarkers based on FLT PET parametric images.
Conclusion
Worse outcome after radiotherapy was significantly
associated with higher pre- or mid-therapy overall K
i
.
Additionally, we found that various imaging biomarkers
derived from vascular parameters or their change through
the therapy, contains even stronger prognostic
information than the FLT transport parameter, which
justify use of kinetic analysis.
PO-0890 PET-based radiobiological modeling of changes
in tumor hypoxia during chemoradiotherapy
M. Crispin Ortuzar
1
, M. Grkovski
1
, B.J. Beattie
1
, N.Y.
Lee
2
, N. Riaz
2
, J.L. Humm
1
, J. Jeong
1
, A. Fontanella
1
,
J.O. Deasy
1
1
Memorial Sloan Kettering Cancer Center, Medical
Physics, New York, USA
2
Memorial Sloan Kettering Cancer Center, Radiation
Oncology, New York, USA
Purpose or Objective
To develop a mechanistic radiobiological model of tumor
control probability (TCP) for predicting changes in tumor
hypoxia during chemoradiotherapy, based on pre-
treatment imaging of perfusion and hypoxia with
18
F-
Fluoromisonidazole (FMISO) dynamic PET and of glucose
metabolism with
18
F-Fluorodeoxyglucose (FDG) PET.
Material and Methods
The mechanistic prediction is based on a radiobiological
TCP model describing the interplay between tumor cell
proliferation and hypoxia (Jeong et al., PMB 2013). The
study presented here (see Sup. Figure 1) focuses on a
cohort of 35 head and neck cancer patients treated with
chemoradiotherapy which received baseline FDG PET and
FMISO dynamic PET, and intra-treatment FMISO dynamic
PET scans, and which excluded subjects having a
significant increase in hypoxia during treatment. The
model is used to predict the radiobiological evolution of
each tumor voxel of the baseline image up until the intra-
treatment scan (9.2±3.4 days). The main inputs to the
model are the initial fractions of proliferative and hypoxic
tumor cells in each voxel, obtained from an approximate
solution to a system of linear equations relating cell
fractions to voxel-level FDG uptake, perfusion (FMISO K
1
)
and hypoxia (FMISO k
3
). For each lesion, the predicted
levels of intra-treatment hypoxia are compared to the
measured k
3
from the intra-treatment scan. A single global
parameter (the average fraction of extremely hypoxic
cells that take up FMISO) is determined from a training
subset of 29 lesions by minimizing the average discrepancy
between each lesion’s measured and predicted intra-
treatment k
3
histograms (Cramér-von Mises criterion). A
validation subset of 10 lesions is held out to test the
resulting model.
Results
The average fraction of extremely hypoxic cells that take
up FMISO is 0.15 (95% CI 0.05 – 0.30 on bootstrap). In the
training subset, the model predicts the mean, median and
standard deviation of each lesion’s intra-treatment k
3
histograms (Pearson’s linear correlation coefficients
between predicted and measured values of ρ=0.62, 0.60
and 0.69 respectively, all with positive 95% CI on bootstrap
– see Sup. Table 1). In the validation subset, only the
predictions of the intra-treatment mean and median k
3
of
each lesion are significant (ρ=0.59 and 0.60 respectively).