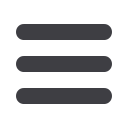

INFORMS Nashville – 2016
128
MA20
106C-MCC
Healthcare Analytics: Big Data, Little Evidence
Invited: Tutorial
Invited Session
Chair: Joris van de Klundert, Erasmus University, Institute of Health
Policy & Management, 2983 HE Ridderkerk, Netherlands,
vandeklundert@bmg.eur.n
1 - Healthcare Analytics: Big Data, Little Evidence
Joris van de Klundert, Erasmus University, Institute of Health
Policy & Management, 2983 HE Ridderkerk, Netherlands,
vandeklundert@bmg.eur.n
While the healthcare sector contributes more than ten percent of GDP in most
developed countries and is approaching twenty percent in the US, it remains a
relatively modest area in the field of Operations Research, Management Science,
and Analytics. There is considerable room for a larger and more valuable
contribution, especially in view of the important advancements in information
technology taking place in healthcare across the globe, which are already
contributing to reducing the global burden of disease. In order for Analytics
professionals and scientists to reach the full contribution potential of their
discipline, it is beneficial to understand the dominant research paradigms and
results of clinical and health sciences research. These sciences are rooted in
empirical evidence, in empirical data, thus offering connection opportunities. In
this tutorial we review the current position of Analytics as covered in the
Operations Research and Management Science literature, and outline a path for
the science of Analytics to enlarge its contribution to the health of populations.
MA21
107A-MCC
Applications of Health Systems Analytics
Sponsored: Health Applications
Sponsored Session
Chair: Muge Capan, Christiana Care Health System, Value Institute,
4755 Ogletown-Stanton Road, 2nd Floor, Suite 2E55, Newark, DE,
19718, United States,
Muge.Capan@ChristianaCare.org1 - Sepsis: Sepsis Early Prediction Support Implementation System
Nisha Nataraj, North Carolina State University, NC, United States,
nnatara@ncsu.edu,Julie Ivy, Muge Capan, Ryan Arnold,
James R Wilson
Sepsis can be broadly defined as an infection plus systemic manifestations of the
infection. It remains the most expensive condition in hospitals as well as one of
the leading causes of in-hospital mortality. Using a discrete-event simulation
framework, this research aims to develop personalized intervention policies for
patients with sepsis. Specifically, we focus on the toll comorbidities and pre-
existing conditions take on the manner in which sepsis presents and the
associated impact they have on decision making.
2 - Validation And Implementation Of Early Warning System To
Synthesize Acuity, Clinical Judgment, And Workload
Stephen Hoover, Christiana Care Health System, Value Institute,
Newark, DE, United States,
Stephen.Hoover@ChristianaCare.orgMuge Capan, Justin M Glasgow, Susan Mascioli, Eric V. Jackson
We present the validation and implementation of a clinical early warning system.
An analytical framework was developed to quantify physiological deterioration
that results in adverse events by integrating a published Early Warning Score and
a new Nurse Screening Assessment tool. Survival Analysis and Monte Carlo
methods were used to validate our approach. Relevant system costs and workload
implications were analyzed. Findings indicate potential for reduction in variation
of care and prevention of unnecessary ICU transfers. Iterative implementation
processes highlighted the importance of multidisciplinary teams and systemwide
education when modifying complex healthcare systems.
3 - Patient Access To Specialty Endocrinology Care
Henry Ballout, University of Michigan, Ann Arbor, MI,
United States,
haballou@umich.edu, Pranjal Singh, Amy Cohn,
Amy E. Rothberg
Recurrent patient visits add tremendous complexity to modeling capacity
utilization for healthcare professionals. To assist an endocrinology clinic at the
University of Michigan, we present a temporal database that takes prospective
patient appointment and provider availability data and enables capacity analysis
through a compilation of daily snapshots. Using this database, we investigate the
clinics issues regarding access and adherence to the program’s highly structured
timeline.
4 - Robust Optimization Framework To Account For Prediction Errors
For Cancer Diagnosis
Selin Merdan, University of Michigan,
smerdan@umich.eduMultiple diagnostic tests are often available for diagnosing diseases such as cancer;
however, how best to use these tests to render a diagnosis is challenging because
there is often a tradeoff between the benefits of diagnosis and the harms and costs
associated with the diagnostic tests themselves. We present a robust optimization
model for determining the optimal assignment of composite diagnostic tests based
on individual patient risk factors to achieve an optimal balance between the
benefits and harms of diagnostic tests. We further provide a specific example in
the context of radiologic imaging to detect metastatic prostate cancer.
MA22
107B-MCC
Healthcare Policy, Personalized Treatment, and
Coalitions: Operations Research Approaches
Invited: ORinformed Healthcare Policies
Invited Session
Chair: Pooyan Kazemian, Harvard Medical School,
25 Shattuck Street, Boston, MA, 02115, United States,
pooyan.kazemian@mgh.harvard.eduCo-Chair: Mark P. Van Oyen, University of Michigan, Ann Arbor, MI,
United States,
vanoyen@umich.edu1 - Depression Care Management: Personalized Assessment To
Cost-effective Population Interventions
Shan Liu, Assistant Professor, University of Washington, Seattle,
WA, 98195, United States,
liushan@uw.eduShuai Huang, Ying Lin, Xuelu Yang, Jiaqi Huang, Weiwei Shang
Depression affects 1 out of 10 Americans. While electronic health record (EHR)
provides an unprecedented information infrastructure, we need a system
perspective and an associated computational platform, and a seamless integration
with decision-analytic models to link the design of personalized disease
interventions to cost-effective population management. We are developing
methods to 1) analyze and predict the heterogeneous depression trajectories of a
patient population from EHR, 2) characterize the hidden disease processes and
design personal intervention schedules, and 3) evaluate their cost-effectiveness to
monitor and treat depression across a range of care scenarios.
2 - National Policy Impact Of High-cost Biologics For
Ophthalmologic Use
David W. Hutton, University of Michigan,
dwhutton@umich.eduIn the last decade, high-cost biologics have taken center stage in the treatment of
complex degenerative eye diseases. Biologics for ophthalmologic use consume
one-sixth of Medicare’s part B drug budget. We examine how OR-based tools can
be used to forecast and evaluate the national policy impact of these therapies (on
both outcomes and costs). We see how different parties frame the problems
differently and make different modeling choices. We discuss how different ways
of using OR-based tools can influence policy. We explore open challenges
evaluating the value of information and understanding how to evaluate decisions
made jointly between patients and providers.
3 - On Personalized Allocation Of Treatments
Mohsen Bayati, Stanford University,
bayati@stanford.edu,
Hamsa Sridhar Bastani, Khashayar Khosravi
Growing availability of data has enabled practitioners to tailor medical treatments
at the individual-level. This involves learning a model of decision outcomes
conditional on individual-specific covariates or features. Recently, “contextual
bandits” have been introduced as a framework to study these online decision
making problems. In this talk we discuss statistical challenges that arise in such
data-driven allocation of treatments.
4 - From Incident To Inpatient: How Healthcare Coalitions Can
Improve Community Response
Jonathan Helm, Indiana University,
helmj@indiana.eduAlex Mills, Andres Jola-Sanchez, Mohan V Tatikonda,
Bobby Courtney
Healthcare coalitions are a new type of organization that can coordinate casualty
distribution among available hospitals in a metropolitan area after a multiple
casualty incident. Using data from a major metro area, we show the value of
different types of coordination regarding hospitals’ capacity information. We find
that, while coalitions were initially create for large disasters, significant value
comes in coordinate metropolitan area hospital resources on a much smaller
scale. We also identify what types of information are most valuable for these
organizations to collect and disseminate. This leads to interesting policy
implication for the further funding and creation of such coalitions.
MA20