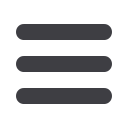

INFORMS Nashville – 2016
124
MA09
103B-MCC
Energy System Design and Optimization
Invited: Energy Systems Management
Invited Session
Chair: Kai Pan, University of Florida, 303 Weil Hall, Gainesville, FL,
32611, United States,
kpan@ufl.edu1 - An Asynchronous Dual Decomposition Algorithm For Stochastic
Unit Commitment
Ignacio Aravena, PhD Student, Université catholique de Louvain,
Voie du Roman Pays 34 bte L1.03.01, Louvain-la-Neuve, 1348,
Belgium,
ignacio.aravena@uclouvain.be, Anthony Papavasiliou
We present an asynchronous dual decomposition algorithm for solving
transmission constrained stochastic unit commitment (UC) under multi-area
renewable production uncertainty with a sub-hourly resolution. Dual iterations
rely upon asynchronous subgradient methods, while primal candidates are
recovered from dual subproblem solutions. The algorithm is implemented on a
high performance computing cluster and its performance is compared to a
deterministic UC model with exogenous reserve targets. The superior
performance of stochastic UC in terms of expected cost, reliability, and run time is
demonstrated on an industrial scale test case of the Central Western European
region.
2 - Valid Inequalities For Hydro Genco Self-scheduling Optimization
Minseok Ryu, University of Michigan, Ann Arbor, MI,
United States,
msryu@umich.edu, Antonio J. Conejo, Ruiwei Jiang
We study on a self-scheduling optimization problem for a hydro generation
company (GENCO) that manages a set of interconnected hydro reservoirs. We
consider a class of key physical and operating characteristics of hydro reservoirs
and generators in practical settings, including the minimum up/down time, the
prohibited operating zones, and the nonlinear performance curves. We employ a
mixed-integer linear programming (MILP) approach to formulate or approximate
these characteristics. The MILP approach facilitates the use of many efficient
computational tools, e.g., optimization solvers and valid inequalities. Finally,
numerical experiments are conducted based on a real-world case study.
3 - A Bi-level Decision Dependent Stochastic Programming Model
For Generation Investment Planning
Yiduo Zhan, University of Central Florida,
yzhan@knights.ucf.eduQipeng Zheng
A multistage bilevel decision dependent stochastic model is presented to tackle
the generation investment planning problem. This model addresses both
exogenous and endogenous uncertainties. The upper-level focuses on a long-term
generation planning problem. The lower-level represents an electricity pricing
problem that addresses the market clearing consideration with local transmission
network. A linear reformulation solution approach is developed for nonlinear
terms. The optimization model is implemented to CPLEX with C++. Real-world
scenarios are tested.
4 - Optimal Bidding Strategy For Electricity Market Participants
Considering Wind And Price Uncertainties
Kai Pan, University of Florida, 411 Weil Hall, Gainesville, FL,
32611, United States,
kpan@ufl.edu,Yongpei Guan,
Jean-Paul Watson
An optimal bidding strategy is derived for independent power producers (IPPs) by
attending both day-ahead (DA) and real-time (RT) markets as a price taker. The
IPP submits an offer of generation amounts to the DA market, for which a
multistage adaptive optimization setting is explored for submitting RT market
offers for each hour as a recourse by utilizing the more accurate forecasting of
renewable output and RT price as the forecast range shrinks. This proposed
strategy is theoretically justified of its significant advantages over existing
alternative ones. The numerical studies show the promising future of adapting the
proposed strategy and verify the effectiveness of the proposed cutting planes.
MA10
103C-MCC
SpORts: Bracketology
Sponsored: SpORts
Sponsored Session
Chair: Laura Albert McLay, University of Wisconsin-Madison,
1513 University Avenue, Madison, WI, 53706, United States,
laura@engr.wisc.edu1 - A Modified Logistic Regression Markov Chain Model For Ranking
College Basketball And Football Teams And Forecasting
Game Outcomes
Laura Albert McLay, University of Wisconsin-Madison,
laura@engr.wisc.eduSelecting the teams for the College Football Playoff for NCAA Division IA men’s
football is a controversial process performed by the selection committee. We
present a method for forecasting the four team playoff weeks before the selection
committee makes this decision. Our method uses a modified logistic
regression/Markov chain model for rating the teams, predicting the outcomes of
the unplayed games, and simulating the unplayed games in the remainder of the
season to forecast the teams that will be selected for the four team playoff. You
can check out the methodology and results at
http://bracketology.engr.wisc.edu/2 - Sampling From The 9,223,372,036,854,775,808 Possible
Brackets In The Ncaa Men’s Basketball Tournament Using The
Power Model
Arash Khatibi, University of Illinois, Urbana, IL, 61802,
United States,
khatibi2@illinois.edu, Douglas M King,
Maryam Kazerooni, Sheldon H Jacobson
This paper proposes the Power Model to estimate the winning seed distribution
out of 9,223,372,036,854,775,808 possible brackets for the NCAA basketball
tournament. The Power Model incorporates both the possibility of upsets and the
better performance of stronger seeds by quantifying the relative strength of each
pair of teams as a power function of their seed numbers. The Power Model is
assessed based on the aggregate performance of one million brackets, which are
generated for the five most recent tournaments (2012-2016) and scored using the
ESPN scoring system.
3 - Predicting The Other Bracket Analysis Of The Selection Process
For The National Invitation Tournament
Stephen Hill, University of North Carolina - Wilmington,
hills@uncw.eduIn this work the selection process for college basketball’s National Invitation
Tournament (NIT) is examined. Using historical selection data, models are
constructed from variables that are shown to be strong predictors of NIT
tournament selection. Model quality is also assessed.
MA11
104A-MCC
Network Optimization Models and Applications I
Sponsored: Optimization, Network Optimization
Sponsored Session
Chair: Jorge A Sefair, Arizona State Univerity, 699 S. Mill Ave., BYENG
330, Tempe, AZ, 85281, United States,
jorge.sefair@asu.edu1 - Optimizing The Recovery Of Disrupted Multi-echelon Assembly
Supply Chain Networks
Huy Q Nguyen, Rensselaer Polytechnic Institute, 110 8th Street,
5119 Center for Industrial Innovation, Troy, NY, 12180,
United States,
nguyeh7@rpi.edu,Thomas Sharkey,
John E. Mitchell, William (Al) Wallace
We consider the problem of recovering multi-echelon assembly supply chain
networks from large-scale disruptive events. Each supplier within this network
assembles a component from a series of sub-components received from other
suppliers. We show that scheduling rules applied locally at each supplier can
optimize key recovery metrics including minimizing the maximum tardiness of
any order of the final product of the supply chain network and minimizing the
time to recover from the event. Our approaches are applied to a data set modeling
an industry partner’s supply chain.
MA09