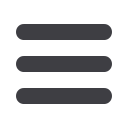

INFORMS Nashville – 2016
121
SD93
Davidson Ballroom C-MCC
Panel: Trends in Service Systems Research Funded
by NSF: Overview of Opportunities for the
Human-technology Frontier
Panel Session
Moderator: Alexandra Medina-Borja, NSF/ UPRM, PFI: BIC - Smart
Service Systems, Falls Church, VA, United States,
amedinab@nsf.gov1 - Trends In Service Systems Research funded byNSF: Overview Of
Opportunities For The human-technology frontier
Alexandra Medina-Borja, US National Science Foundation,
Arlington, VA, 2, United States,
alexandra.medinaborja@upr.eduAn overview of interdisciplinary funding opportunities for researchers modeling
the interaction between humans and engineered systems that could enable the
smart service systems of the future. Requirements and opportunities will be
discussed by one of the NSF cognizant program officers in this program.
2 - Panelist
David Mendonca, Rensselaer Polytechnic Institute, New York, NY, 0,
United States,
mendod@rpi.eduMonday, 8:00AM - 9:30AM
MA01
101A-MCC
Learning from Uncertain Data with High
Dimensionality
Sponsored: Data Mining
Sponsored Session
Chair: Neng Fan, University of Arizona, Engineering Bldg, Room 312,
1127 E. James E. Rogers Way, Tucson, AZ, 85721, United States,
nfan@email.arizona.edu1 - Multiclass Support Vector Machines With Labeling Uncertainty
Wanlu Gu, University of Arizona,
wanlugu@email.arizona.eduThe multiclass support vector machines (SVMs) is an extension of the
conventional SVM in machine learning, and it builds the classification
hyperplanes based on a set of training data points. In practice, the real collected
data may have noise or uncertainty. In this talk, we consider the observed data
with noise on the labels, and construct models and algorithms to learn from this
type of uncertain data. To model the uncertainty, the noise probability is assumed
to the labeling noise from one class to the others. Then some novel optimization
models are proposed and also validated through numerical experiments to check
the difference with noise free models.
2 - Sparse Support Vector Machines With Data Uncertainty
Ammon Washburn, University of Arizona,
wammonj@email.arizona.eduData with high dimensionality and uncertainty comes about when there are too
many features and the data is unreliable, replicated or missing. Without taking
these issues properly into account, classification models will overfit the training
data. In order to deal with these two problems, sparse representations and
chance-constrained programming have emerged separately. We will show how to
implement both ideas by modifying Support Vector Machines in a way that is not
overly conservative which we call Decoupled Margin-Moment SVM. Numerical
experiments are performed on collected pancreatic cancer data.
3 - Graph Clustering Of Data With Uncertainties
Yujia Zhang, University of Arizona,
yujiazhang@email.arizona.eduIn this talk, we will review models and algorithms for clustering of data with
uncertainties. First, the methods to model data uncertainty will be reviewed.
Second, we mainly concentrate on the graph models for clustering. Finally,
algorithms for solving these models will be reviewed and compared.
4 - Constrained Clustering Of Uncertain Data
Derya Dinler, PhD Candidate, Middle East Technical University,
ODTU Endustri Muhendisligi Bolumu, Cankaya, Ankara, 06800,
Turkey,
dinler@metu.edu.tr, Mustafa Kemal Tural
We consider a constrained clustering problem where the locations of the data
objects are subject to uncertainty. Each uncertainty set is assumed to be either a
closed convex bounded polygon or a closed disk. The final clustering is expected
to be in accordance with a given number of instance level constraints. We propose
a mixed-integer second order cone programming formulation for the considered
clustering problem which is only able to solve small-size instances. For larger
instances, approaches from the semi-supervised (constrained) clustering literature
are modified and compared in terms of computational time and quality.
MA02
101B-MCC
New Advancements in Using Data Analytics for
Healthcare Applications
Sponsored: Data Mining
Sponsored Session
Chair: Talayeh Razzaghi, Clemson University, 100 McAdams Hall,
Clemson, SC, 29634, United States,
talayeh.razzaghi@gmail.com1 - Using Density To Identify Fixations In Gaze Data:
Optimization-based Formulations And Algorithms
Andrew C Trapp, Worcester Polytechnic Institute,
atrapp@wpi.eduEye tracking is an increasingly common technology with a variety of practical
uses. Eye-tracking gaze data can be categorized into two main events: fixations,
which represent attention, whereas saccades occur between fixation events. We
propose a novel manner to identify fixations based on their density, which
concerns both the fixation duration as well as its inter-point proximity. We
develop two mixed-integer nonlinear programming formulations and
corresponding algorithms to recover the densest fixations in a data set. Our
approach is parameterized by a unique value that controls for the degree of
desired density. We conclude by discussing computational results and insights on
real data sets.
2 - Leveraging Longitudinal Healthcare Data For
Inverse Classification
Michael Lash, University of Iowa,
michael-lash@uiowa.edu,Nick Street
Inverse classification is the process of manipulating a test point to minimize the
predicted probability of a specific class label. Such a process has been shown to be
beneficial to healthcare-related problems such as lifestyle modification and
treatment recommendations. Past work in this area has focused on single
snapshots in time, which does not account for the history or behavioral changes
of patients. In this work we incorporate longitudinal information into our inverse
classification model and demonstrate its effectiveness in mitigating the long-term
risk of cardiovascular disease.
3 - Stability And Performance Of Healthcare Access Supply-demand
Systems Affected by Stochastic Time Delays
Sara Nourazari, California State University-Long Beach,
Bellflower Boulevard, Long Beach, CA, 90840, United States,
Sara.Nourazari@csulb.edu,Rifat Sipahi, James Benneyan
Time delays are an inevitable aspect of many healthcare supply-demand systems
and can potentially lead to undesirable outcomes and decision making challenges.
We propose an approach to characterize “expected” stability maps of healthcare
access supply-demand systems affected by random time delays following a known
probability distribution. This study aims to enable broader insight into the effects
of random process delays, and across a wide range of test applications in
healthcare queue management, demonstrates minimized undesirable oscillatory
behaviors and improved system performance.
MA03
101C-MCC
Daniel H. Wagner Prize Competition I
Invited: Daniel H. Wagner Prize Competition
Invited Session
Chair: C. Allen Butler, Daniel H Wagner Associates, Inc., 2 Eaton Street,
Hampton, VA, 23669, United States,
Allen.Butler@va.wagner.com1 - Calibrated Route Finder – Social, Environmental And
Cost-effective Truck Routing
Mikael Ronnqvist, Professor, Universite Laval, Pavillion Adrien-
Pouliot, Bureau 3345, 1065 Avenue De La Medecine, Quebec, QC,
G1V OA6, Canada,
mikael.ronnqvist@gmc.ulaval.caGunnar Svenson, Patrik Flisberg, Lars-Erik Jönsson
Finding the best route with many conflicting objectives is very difficult. The
online system Calibrated Route Finder has been developed in collaboration
among many companies and organizations and successfully addresses the
problem. A key component is an inverse optimization process that establishes
more than 100 weights to balance social values, environmental impacts, traffic
safety, stress, fuel consumption, CO2 emissions, and costs. In addition,
methodological and analytic developments now enable measurement and
inclusion of perceived hilliness and curviness as well as strict rules where to drive.
The system has been in operations since 2009 and is today used by about 100
companies.
MA03