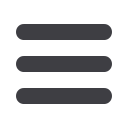

INFORMS Nashville – 2016
123
MA06
102A-MCC
Social Media Analytics
Sponsored: Data Mining
Sponsored Session
Chair: Julie Zhang, University of Massachusetts Lowell, One University
Avenue, Lowell, MA, 01854, United States,
Juheng_Zhang@uml.edu1 - Challenging The Spatial Homogeneity Of Online Reviews
Theodoros Lappas, Stevens University of Technology,
tlappas@stevens.eduThe popularity of online reviews has led to the emergence of large review-hosting
platforms that rank businesses by aggregating their reviews. The standard
aggregation approach naively considers only the review’s text, stars, and date,
while ignoring the reviewer’s circumstances. In this work we hypothesize that the
reviewer’s role as a local or visitor has a significant effect on the content and
valence of his review. Our study reveals significant differences between the two
populations and provides strong evidence against spatial homogeneity. We
provide a detailed analysis of our findings and discuss their implications for
consumers, business owners, and review-hosting platforms.
2 - Aspect Mining For Discovering Demand-side Knowledge In Online
Customer Reviews
Zhilei Qiao, Virginia Tech,
qzhilei@vt.edu,G. Alan Wang
Online reviews provide important demand-side knowledge from customers to
improve mobile app product quality. However, discovering and quantifying
potential app product new feature requests and defects from large amounts of
unstructured text is a nontrivial task. In this paper, we propose a Latent Domain-
Side Knowledge Analysis (LDSKA) that identifies the most critical app product
new features and corresponding product feature status, simultaneously.
Experimental results demonstrate that our proposed model outperforms existing
LDA model. Our research has significant managerial implications for app
developers, app customers and app platform providers.
3 - Strategically Information Disclosure On Social Media
Julie Zhang, University of Massachusetts Lowell,
Juheng_Zhang@uml.eduWith the prevalence of social media, more firms are using social media to disclose
financial information. Unlike 10-k forms, firms have freedom to choose when and
how to disclose “good” or “bad” news on social media. There are strategic
behaviors of firms in information disclosure on social media. We investigate the
strategic information disclosure of firms on social media.
4 - On Predicting Social Protest Using Social Media
Rostyslav Korolov, Doctoral Student, Rensselaer Polytechnic
Institute, 110 Eighth street, Troy, NY, 12180, United States,
korolr@rpi.edu, Di Lu, Jingjing Wang, Guangyu Zhou,
Claire Bonial, Clare Voss, Lance Kaplan, William A Wallace,
Jiawei Han, Heng Ji
We study the possibility of predicting a social protest based on social media
messaging. We suggest that the frequency of text concerning the stages in the
process of mobilization may be used to predict an imminent protest. We utilized
several Natural Language Processing techniques to identify mobilization in social
media. Our experiments with Twitter data collected before and during the 2015
Baltimore events show a correlation over time between volume of Twitter
communications related to mobilization and occurrences of protest, thereby
enabling estimation of the likelihood of a protest.
MA07
102B-MCC
Business Process Intelligence
Sponsored: Data Mining
Sponsored Session
Chair: Zhe Shan, University of Cincinnati, Cincinnati, OH,
United States,
zhe.shan@uc.edu1 - Tree-based Models For Longitudinal Data
Peng Wang, University of Cincinnati,
wangp9@ucmail.uc.eduDan Liu, Brittany Green
Classification and regression tree (CART) has been broadly applied due to its
simplicity of explanation, automatic variable selection, visualization and
interpretation. Previous algorithms for constructing CART for longitudinal data
suffer from the computational difficulties in estimation of covariance matrix at
each node. We proposed to utilize the quadratic inference function (QIF) and
developed a new criterion, named RSSQ, to select the best splits. The proposed
approach incorporates correlation wihout estimating the correlation parameters.
Therefore we could improve the efficiency of the partition results and prediction
accuracy. This is joint work with Dan Liu and Brittany Green
2 - Mining Process Patterns Via Electronic Medical Record
Audit Logs
He Zhang, University of South Florida,
hezhang@usf.eduMining the process patterns in the access logs from information systems can
provide useful insights for the workow patterns. One important issue in process
mining is that the workow is usually highly dynamic and the access logs are
noisy. We presenta framework to analyze process models with noisy data at an
abstract level. We implement our approach using several months of data from a
large academic medical center. Empirical results show that our framework can
extract the process models effectively.
3 - Feature Selection For Quality Assessment Of Predicted
Protein Structures
Shokoufeh Mirzaei, California State Polytechnic University -
Pomona, CA,
smirzaei@cpp.eduIn the context of computational biology a scoring function determines the quality
of a predicted protein structure. The Goal of this paper is to find a subset of
protein features that are critical in identifying native protein structures in order to
develop a scoring function. In pursuit of this goal, our method of research consists
of 1) identify a set of protein features suggested by the literature, 2) use a variety
of feature selection methods to select the best subset of features 3) use deep
learning techniques in machine learning to identify new features. The final
Outcome of this research is a subset of protein features and a new scoring
function which predicts the quality of protein models.
4 - Healthcare Fraud Analysis Using Sequential Data Mining
Babak Zafari, Babson College, Boston, MA, United States,
zafari.babak@gmail.comIt is estimated that at least three percent of annual health care spending is lost to
overpayments. However, the large size and complexity of the health care system
make comprehensive auditing infeasible. This resulted in the use of data mining
approaches to detect unusual payments. In this talk, we propose the use of
pattern discovery methods to find the anamolies in the medical claims payment
data.
MA08
103A-MCC
Crowd-based Innovation
Invited: Business Model Innovation
Invited Session
Chair: Bilal Gokpinar, UCL, London, United Kingdom,
b.gokpinar@ucl.ac.uk1 - Experience Breadth And Problem-solving In Crowdsourcing
Contests: An Empirical Investigation
Anant Mishra, George Mason University, Fairfax, VA, 22030,
United States,
amishra6@gmu.edu,Nirup M Menon, Shun Ye
Online crowdsourcing contests have become a popular mechanism for addressing
challenging problems. In this study, we use a multi-dimensional classification
scheme to represent a contestant’s breadth of experience on a crowdsourcing
platform, and examine how it impacts her performance in a contest. Using
detailed archival data from TopCoder, a crowdsourcing platform that hosts
contests across software development problem domains (e.g., architecture, design,
testing), our results demonstrate that a contestant’s breadth of experience has a
nuanced relationship with her performance in a contest.
2 - Spatial Distribution Of Alternative Finance
Mingfeng Lin, University of Arizona, 1130 E. Helen St, Tucson, AZ,
85721, United States,
mingfeng@eller.arizona.edu, Bryan Zhang
Will online alternative finance help reduce the geographical imbalance of capital
that the literature has long documented of the traditional finance? Are funding
distributions geographically more equitable online, and are there differences
across different types of crowdfunding forms? We answer these questions by
leveraging detailed transactions data from multiple major crowdfunding platforms
in the UK. We compare the spatial flow in online debt crowdfunding to bank
lending, and online equity crowdfunding to venture capital and private equity
financing. We also compare the funding flow between different types of
crowdfunding. Results show some surprising and interesting patterns.
3 - The Role Of Customer Investor Involvement In
Crowdfunding Success
Philipp Cornelius, University College London, London, United
Kingdom,
philipp.cornelius.12@ucl.ac.uk, Bilal Gokpinar
Entrepreneurs and organisations increasingly use crowdfunding to fund
innovation projects through a large number of customer investments. The
growing literature on the topic has predominantly studied crowdfunding in terms
of its financing mechanism. The involvement of customers during crowdfunding,
however, goes beyond the provision of capital. As investors and prospective
product customers, crowdfunders want to influence crowdfunding campaigns.
Can project creators benefit from this or does an increased influence of the crowd
push products into too many directions and deter other customers from funding?
MA08