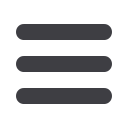

INFORMS Nashville – 2016
120
SD86
GIbson Board Room-Omni
Manufacturing IV
Contributed Session
Chair: Ali AlArjani, PhD Candidate, University of Wisconsin -
Milwaukee, 4848 N. Lydell Ave, Apt 141, Milwaukee, WI, 53217,
United States,
alarjan2@uwm.edu1 - Setting Optimal Planned Leadtimes In A Configure To Order
Manufacturing System
Sjors Jansen, PhD Candidate, Eindhoven University of Technology
(TU/e), P.O. Box 513, Paviljoen E13, Eindhoven, 5600MB,
Netherlands,
s.w.f.jansen@tue.nl, Zumbul Atan, Ton de Kok,
Ivo Adan
We study the production planning in Configure To Order (CTO) manufacturing
systems. The system consists of multiple stages that converge to one final
assembly stage. Leadtimes per stage are stochastic due to extensive testing at the
end of each stage. Our goal is to determine optimal planned leadtimes for each
stage such that the total expected production costs are minimized. We derive
Newsvendor equations for each individual stage. This set of equations is solved
and the exact optimal planned leadtimes for each stage are obtained. These
equations give an important insight in the dynamics of the system, since they
indicate to what extend a specific stage can be blamed for the lateness of the final
product.
2 - Introduction Of A Motor Assembly Test Bed To Verify
Manufacturing Technology
Jungryul Bae, Korea Institute of Industrial Technology (KITECH),
Seoul, Korea, Republic of,
somanythat@naver.com, SeHwan Ahn,
YongJu Cho, Chul Kim, Hyunchul Tae
A Testbed is a place of verifying newly developed manufacturing technologies
before apply it in practice. We built a motor-assembly Testbed that comprises
seven connected facilities in a line. We have tested several manufacturing
technologies including simulation, quality management, and IoTs on the Testbed.
In this presentation, we aim to introduce our Testbed and share our experience.
The final goal of the Testbed is to enhance the localization ratio of a convergence
of the IoTs and manufacturing technology.Keywords: Connected Smart Factory
(CSF), Testbed, IoTs, manufacturing technology.
3 - Modeling The Impact Of Product Variety On Inventory: Application
To Strategic Assembly Sequencing And Supply Chain Design
Jeonghan Ko, University of Michigan; Ajou University, 1205 Beal
Ave., Industrial & Operations Engineering, Ann Arbor, MI, 4810,
United States,
jeonghan@umich.edu, Heng Kuang
This paper models the impact of variety on assembly supply chain design when
limited commonality exists between products. We derive theorems on the impact
of product variety on safety inventory, and provide a measure to approximate the
impact. The theorems and new measure are applied in two problems: optimal
process sequencing and optimal assembly decomposition. We prove that to
prioritize the process with a smaller number of variants will reduce the supply
chain cost no matter the commonality is.
4 - Finite Capacity Material Requirement Planning System For Supply
Chain Network
Benyaphorn Paopongchuang, Sirindhorn International Institute of
Technology, Pathum Thani, 12121, Thailand,
bp322@njit.eduBenyaphorn Paopongchuang, New Jersey Institute of Technology,
Newark, NJ, 07102, United States,
bp322@njit.edu, Pisal Yenradee
Available Finite Capacity Material Requirement Planning (FCMRP) systems have
some limitations. They are designed to determine production and purchasing
plans in only one factory not in a multi-level supply chain network. Most systems
lack of optimization capabilities. In addition, they do not manage bottleneck
effectively. The proposed algorithm tries to develop FCMRP system that also
considers finite capacity of some key suppliers and customers.
5 - Similarity Coefficient Model For Solving an Oil Global Facility
Location Problem
Ali AlArjani, PhD Candidate, University of Wisconsin - Milwaukee,
4848 N lydell Ave, Milwaukee, WI, 53217, United States,
alarjan2@uwm.eduSolve an oil global facility location problem by a new similarity coefficient model
for cluster analysis that model ranks multiple countries and cluster them in
groups each group have similar attributes.
SD91
Davidson Ballroom A-MCC
Joint Session HAS/MSOM-HC: Statistical Decision-
Making with Applications in Healthcare
Sponsored: Manufacturing & Service Oper Mgmt,
Healthcare Operations
Sponsored Session
Chair: Mohsen Bayati, Stanford University, Stanford, CA, United States,
bayati@stanford.eduCo-Chair: Hamsa Bastani, Stanford University, 10 Comstock Circle,
Apt 304, Stanford, CA, 94305, United States,
hsridhar@stanford.edu1 - Approximation Methods For Adaptive Clinical Trial Design
John R Birge, University of Chicago,
John.Birge@ChicagoBooth.edu2 - An Analytics Approach To Designing Drug Therapies For Cancer
John M Silberholz, MIT, Cambridge, MA, 02139, United States,
josilber@mit.edu, Dimitris Bertsimas
We present a data-driven approach to planning clinical trials and designing novel
drug therapies for metastatic breast cancer (MBC). First, we describe construction
of a large database of MBC clinical trial results and tools to help clinicians
visualize the data. Next, we use statistical models to predict efficacy and toxicity
outcomes of trials before they are run, with implications for selecting between
multiple drug therapies for testing. Finally, we use optimization models to design
novel therapies that strike a balance between maximizing patient outcomes and
learning about new drugs; initial evaluation suggests these models may improve
trial outcomes compared to current practice.
3 - Online Decision-making With High-dimensional Covariates
Hamsa Sridhar Bastani, Stanford University, 10 Comstock Circle,
Apt 304, Stanford, CA, 94305, United States,
hsridhar@stanford.edu,Mohsen Bayati
Big data has enabled decision-makers to personalize choices based on an
individual’s observed characteristics. We formulate this problem as a multi-armed
bandit with high-dimensional covariates, and present a new efficient algorithm
that provably achieves near-optimal performance. The key step in our analysis is
proving convergence of the LASSO estimator despite non-iid data induced by the
bandit policy. We evaluate our algorithm using a real patient dataset on warfarin
dosing; here, a patient’s optimal dosage depends on her genetic profile and
medical records. Our algorithm outperforms existing bandit methods as well as
physicians to correctly dose a majority of patients.
4 - Estimating Average Treatment Effects In High-dimensional
Observational Studies
Stefan Wager, Stanford University, Stanford, CA, United States,
swager@stanford.edu,Susan Athey, Guido Imbens
There are many studies where researchers are interested in estimating average
treatment effects and are willing to rely on the unconfoundedness assumption,
which requires that treatment assignment is as good as random conditional on
pre-treatment variables. The unconfoundedness assumption is often more
plausible if a large number of pre-treatment variables are included in the analysis,
but this can worsen the finite sample properties of existing approaches to
estimation. In this paper, we propose a new method for estimating average
treatment effects in high dimensions that achieves the semi-parametric efficiency
bound without requiring any modeling assumptions on the propensity score.
SD92
Davidson Ballroom B-MCC
INFORMS Optimization Society Prize Session
Award Session
Chair: Suvrajeet Sen, University of Southern California, 3715
McClintock Ave, Los Angeles, CA, 90089, United States,
s.sen@usc.edu1 - Optimization SocietyAwards
Suvrajeet Sen, University of Southern California, 3715 McClintock
Ave, Los Angeles, CA, 90089, United States,
s.sen@usc.eduThe Optimization Society sponsors four awards annually. They are a) the
Khachiyan Prize for lifetime contributions in optimization, b) the Farkas Prize for
exceptional mid-career accomplishments, c) the Young Optimization Researcher
award, and finally, d) the student paper prize competition. These awards are
highly competitive and coveted, and this session is dedicated to congratulating the
winners, and their lasting contributions to optimization. The award winners will
present brief overviews of their prize-winning contributions.
SD86