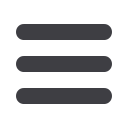

INFORMS Nashville – 2016
117
SD68
Mockingbird 4- Omni
Process Monitoring, Diagnosis, and Prognosis in
Complex Systems
Sponsored: Quality, Statistics and Reliability
Sponsored Session
Chair: Qiang Zhou, City University, 1, Kowloon, 1, Hong Kong,
q.zhou@cityu.edu.hkCo-Chair: Li Zeng, Texas A&M University, College Station, TX,
United States,
lizeng@tamu.edu1 - High Dimensional Process Monitoring Using Sparse Principal
Component Analysis
Mohammad Nabhan, Georgia Institute of Technology,
nabhan@gatech.edu, Jianjun Shi
Dimension reduction techniques, such as PCA and PLS, have been used for
process monitoring in statistical process control. However, in high dimensional
settings they suffer from inconsistency and interpretability issues. Sparse principal
component analysis (SPCA) has been shown to be more consistent in these
settings. Due to its sparse nature, it allows for better interpretation. This article
proposes a monitoring and diagnostics scheme utilizing SPCA to reduce the
dimensionality of the data while improving interpretability. The method is
effective under certain stipulations on the spatial structure of the data streams.
The proposed method is validated through simulation and a case study.
2 - Monitoring Low-e Glass Manufacturing Using Optical Profiles
Qian Wu, Texas A&M University, College Station, TX, 77840,
United States,
hi_qianwu@tamu.edu, Li Zeng
In this study we develop a method for process monitoring using optical profiles
collected from low-E glass products. The proposed method uses a piecewise
polynomial mixed-effect model to characterize the complex shape of optical
profiles and a T2 control chart to monitor the estimated random effects for change
detection. We investigate a potential problem caused by high correlations of
random effects in implementing this method and propose a remedy based on
regressor transformation for this issue. A case study will be shown, indicating the
proposed method fits the real optical profiles and performs well in process
monitoring.
3 - Remaining Useful Life Prediction In Populations
With Heterogeneity
Raed Kontar, University of Wisconsin - Madison, Madison, WI,
United States,
alkontar@wisc.eduDegradation signal data used for prognosis are often imbalanced as most units are
reliable and only few tend to fail at early stages of their life cycle. Such
imbalanced data may hinder accurate remaining useful life (RUL) prediction
especially in terms of detecting pre-mature failures as early as possible. . In this
paper, we propose a degradation signal-based RUL prediction method to address
the imbalance issue in the data. The proposed method introduces a mixture prior
distribution to capture the characteristics of different groups within the same
population and provides an efficient and effective online prediction method for
the in-service unit under monitoring.
4 - Statistical Monitoring And Fault Diagnosis Of Vibration Signal
Based On Wavelet Transform
Wei Fan, City University of Hong Kong, Kowloon, Hong Kong,
weifan8-c@my.cityu.edu.hk,Qiang Zhou
To effectively monitor and detect the early fault of rolling bearing, a wavelet-
based statistical process control method is proposed and studied. The vibration
signal is decomposed by orthonormal wavelet transform. The generalized
likelihood ratio test is taken into consideration to detect the shift of the wavelet
coefficients. To increase the detection power of the small shift, the proposed
control chart takes the exponentially weighted moving average of the logarithm
of the likelihood ratio. Both the simulation studies and the experimental cases
show the effectiveness of the proposed method.
SD69
Old Hickory- Omni
Pierskalla II
Award Session
Chair: Baris Ata, University of Chicago, Booth School of Business
Co-Chair: Anton Skaro, Northwestern University, Feinberg School
of Medicine
Co-Chair: Sridhar Tayur, Carnegie Mellon University, Tepper School
of Business
1 - Pierskalla Award
Vikram Tiwari, Vanderbilt University Medical Center, Nashville, TN,
Contact:
vikram.tiwari@vanderbilt.eduThe Health Applications Society of INFORMS sponsors an annual competition for
the Pierskalla Award, which recognizes research excellence in the field of health
care management science. The award is named after Dr. William Pierskalla to
recognize his contribution and dedication to improving health services delivery
through operations research. The Pierskalla award information can be found on
the website at:
https://www.informs.org/Community/HAS/Pierskalla-Award2 - Online Decision-Making with High-Dimensional Covariates
Hamsa Bastani, Mohsen Bayati, Stanford University, Stanford, CA,
bayati@stanford.eduBig data has enabled decision-makers to tailor treatment decisions based on their
clinical information. This involves learning a model of decision rewards
conditional on individual patient covariates. These covariates are high-
dimensional; typically only a small subset of the observed features are predictive
of a decision’s success. We formulate this problem as a multi-armed bandit with
high-dimensional covariates, and present a new efficient bandit algorithm based
on the LASSO estimator. Our analysis establishes that our algorithm achieves
near-optimal performance in comparison to an oracle that knows all the problem
parameters. The key step in our analysis is proving a new oracle inequality that
guarantees the convergence of the LASSO estimator despite the non-i.i.d. data
induced by the bandit policy. We illustrate the practical relevance of our
algorithm by evaluating it on a real-world clinical problem of warfarin dosing. A
patient’s optimal warfarin dosage depends on the patient’s genetic and medical
records. We show that our algorithm outperforms existing bandit methods as well
as physicians to correctly dose patients.
3 - Do Mandatory Overtime Laws Improve Quality? Staffing Decisions
and Operational Flexibility of NursingHomes
Lauren Xiaoyuan Lu, University of North Carolina, Chapel Hill,
NC,
lauren_lu@unc.edu, Susan Feng Lu
During the 2000s, over a dozen U.S. states passed laws that prohibit health care
employers from mandating overtime for nurses. Using a nationwide panel dataset
from 2004 to 2012, we find that these mandatory overtime laws reduced the
service quality of nursing homes, as measured by an increase in deficiency
citations. This outcome can be explained by two undesirable changes in the
staffing hours of registered nurses: decreased hours of permanent nurses and
increased hours of contract nurses per resident day. We observe that the increase
in deficiency citations concentrates in the domains of administration and quality
of care rather than quality of life, and the severity levels of the increased citations
tend to be minor rather than major. We also find that the laws’ negative effect on
quality is more severe in nursing homes with higher percentage of Medicare-
covered residents. These observations are consistent with the predictions of a
stochastic staffing model that incorporates demand uncertainty and operational
flexibility. Further, we rule out an alternative hypothesis that the quality decline
is induced by an increase in nurse wages.
4 - Data-Driven Incentive Design in the Medicare Shared Savings
Program
Anil Aswani, UC Berkeley, Berkeley, CA,
aaswani@berkeley.edu,
Zuo-Jun Shen, Auyon Saddiq
The Medicare Shared Savings Program (MSSP) was created to control escalating
Medicare spending by incentivizing providers to deliver healthcare more
efficiently. Providers that enroll in the MSSP earn bonus payments for reducing
spending to below a risk-adjusted financial benchmark. To generate savings, a
provider must invest to improve efficiency, which is a cost that is absorbed
entirely by the provider under the current contract. This has proven to be
challenging for the MSSP, with a majority of participating providers unable to
generate savings. In this paper, we formulate the MSSP as a principal-agent model
and take a data-driven approach to redesigning the MSSP contract. We propose a
new type of contract that includes a performance-based subsidy that partially
reimburses the provider’s investment. We prove that there exists a subsidized
contract that dominates the current MSSP contract by producing a strictly higher
expected payoff for Medicare and the provider. We then present a maximum
likelihood approach for estimating the parameters of the principal-agent model,
using a dataset containing the financial performance of providers.
SD69