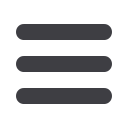

INFORMS Nashville – 2016
142
MA60
Cumberland 2- Omni
Fleet and Marketplace Optimization for Mobility-on-
Demand (MOD) Systems
Sponsored: TSL, Urban Transportation
Sponsored Session
Chair: Samitha Samaranayake, Cornell University, 317 Hollister Hall,
Ithaca, NY, 14853, United States,
samitha@alum.mit.edu1 - Queueing-theoretical Models For Mobility-on-demand Systems:
Theory And Algorithms
Frederico Rossi, Stanford University, Stanford, CA, United States,
frossi2@stanford.edu, Marco Pavone
In this talk I will present recent advances towards modeling and controlling
autonomous mobility-on-demand (AMoD) systems, an emerging mode of
personal transportation wherein robotic, self-driving vehicles transport customers
on-demand. First, I will present queueing-theoretical models inspired by the
theory of Jackson and BCMP queueing networks. Such models provide structural
insights about the performance of AMoD systems and guidelines for the design of
routing algorithms for the robotic vehicles. Then, I will discuss large-scale
coordination algorithms for AMoD systems that are aimed at throughput
maximization and can handle congestion and charging constraints.
2 - Fleet Management In Mobility-on-Demand Systems With
Shared Rides
Samitha Samaranayake, Cornell University,
samitha@alum.mit.eduWe consider a MoD system with ridesharing between passengers. Inherent to the
formulation are two important attributes: (i) the need to rebalance empty vehicles
and (ii) the ability to identify lucrative ridesharing corridors via trip chaining. We
present a mixed-integer linear programming (MILP) formulation of the problem
and show how a heuristic (feasible) solution to the problem can be obtained in
polynomial-time by independently solving the ride-matching and rebalancing
problems. This approximate solution can be used as a initial guess when solving
the coupled problem via an MILP solver.
3 - Dynamic Pricing In Ride-share Platforms
Siddhartha Banerjee, Cornell University,
sbanerjee@cornell.eduMuch of the success of ride-sharing platforms like Lyft and Uber is ascribed to
their ability to do fast-timescale dynamic pricing - where prices can react to
instantaneous system state, and across very small geographic areas. We explore
the value of such dynamic pricing via a model which combines a queueing model
for the dynamics of the platform’s operations with strategic models of both
passenger and driver behavior. In particular, we suggest that dynamic pricing may
not be better than the optimal static price, but rather, allows the platform to
realize the optimal price with limited knowledge of system parameters. Joint
work with Ramesh Johari, Carlos Riquelme, and the data science team at Lyft.
4 - Marketplace Optimization At Uber
Robert Phillips, Uber, Palo Alto, CA, United States,
robert.phillips@uber.comThe rapid acceleration of the sharing economy has introduced a myriad of
challenges for two-sided marketplaces. This talk will address how optimization
and machine learning are powering the dynamic marketplace at Uber, a platform
that has connected over one billion riders and drivers across more than 60
countries. Topics that will be surveyed include dynamic pricing, matching riders
in uberPOOL, and real-time on-demand delivery services.
MA61
Cumberland 3- Omni
Intermodal Transportation
Sponsored: Railway Applications
Sponsored Session
Chair: Mike D Prince, BNSF Railway, Fort Worth
1 - Intermodal Empty Railcar Distribution Optimization
Shantih Spanton, CSX Transportation, Jacksonville, FL,
Shantih_Spanton@csx.com, Jagadish Jampani
Optimization models to effectively reposition the empty railcars. The forecasting
model predicts the future demand for the containers and trailers, which is subse-
quently translated into railcars. This demand data is converted into equivalent
number of railcars which is input into the optimization model. In addition, train
profiles, network and terminal attributes are input into the optimization model.
The model also predicts when and where the loaded railcars will become avail-
able in the selected optimization time horizon. This optimization model is
embedded with a real time tool that is used by the intermodal railcar distribution
team.
2 - Intermodal Hub Simulation
Mike Prince, BNSF Railway, Contact:
mike.prince@bnsf.comIntermodal hubs are the facilities at which BNSF Railway’s intermodal trains
interface with customers. This presentation will discuss an AnyLogic simulation
model that was developed for the purpose of assisting in the capital expansion
planning process for these facilities.
3 - Utilizing Rail Information In Intermodal Operations
Georgi Tasev, Schneider, Contact:
TasevG@Schneider.comAccurate train ETA information is critical to intermodal dray operations and
directly influences the ability to serve customers effectively. In this session, we
will review how Schneider uses train information provided by our rail partners
to optimize key operations, such as appointment setting and dispatch. In addi-
tion, we will cover the analysis that was completed to study the accuracy of rail
ETA information at key time intervals. Lastly, we will discuss the implementation
and results of building a direct feed for train ETA information into Schneider’s
system.
MA62
Cumberland 4- Omni
Determinants of Aviation Strategies and Market
Outcomes
Sponsored: Aviation Applications
Sponsored Session
Chair:SufficMartin E Dresner, University of Maryland-College Park, R H
Smith School of Business, College Park, MD, 20742, United States,
mdresner@rhsmith.umd.edu1 - The Impact Of Predicted Quality On Customer’s Quality
Assurance Behaviors In The Us Airline Industry
Woohyun Cho, University of New Orleans, New Orleans, LA,
United States,
wcho@uno.eduDong-jun Min, Pamela Kennett-Hensel
We empirically examine the drivers of customers’ voluntary quality assurance
behaviors (QAB). Using survey data and archival data from the US airline
industry, we show that whereas an increase in predicted quality of airlines
departure operations (e.g., on-time performance and flight frequencies) leads to a
decrease in the level of QAB (i.e., customer wait time for their flight at the
airport), an increase in price leads to an increase in QAB. Our finding also
indicates that the expense of exercising QAB reduces QAB. We emphasize the
importance of properly measuring the impact of predicted quality and price on
the customer’s role, as it may help share the cost of managing quality with their
customers.
2 - Passenger Facility Charge Vs. Airport Improvement
Program Funds: A Dynamic Network Dea Analysis For
U.S. Airport Financing
Bo Zou, University of Illinois at Chicago, 2073 Engineering
Research Facility, 842 West Taylor Street, Chicago, IL,n
Univ60607, United States,
bzou@uic.eduYoung-Tae Chang, Hyosoo Park, Nabin Kafle
Passenger Facility Charge (PFC) and the Airport Improvement Program (AIP) are
two major sources to finance U.S. airports. This paper develops a novel dynamic
network DEA framework to investigate the substitutability between PFC and AIP
funds. We find that the studied U.S. airports can substitute PFC for 8-35% of the
current AIP funds and contribute significantly to the proposed plan of the US
congress to cut AIP funding. In addition, the amount of PFC-for-AIP funds
substitution negatively correlates with the productive efficiency of airports. The
findings send an important message for future policy reforms on U.S. airport
financing.
3 - Measuring Competition Intensity And Product Differentiation:
Evidence From The Airline Industry
Benny Mantin,University of Waterloo,
bmantin@uwaterloo.caDavid Gillen, Tuba Delibasi
Measuring the degree of competition in markets is essential for policy and
decision makers. Commonly used structural indices (e.g., HHI) overlook how
firms compete with each other and the intensity of the competition. We propose a
new competition measure: Schedule (Temporal) Differentiation Metric, STDM,
which encapsulates firms’ market shares as well as the degree of overlap and
substitution between the competing services—critical elements in service
industries. We demonstrate the STDM using aviation markets revealing a
significant improvement in explaining prices, how the effect varies across fare
percentiles, and how the insights change with the business models of the
competing firms.
MA60