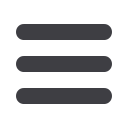

INFORMS Nashville – 2016
147
3 - Price Competition And Direct-to-consumer Advertising In
Prescription Drug Markets
Abhik Roy, Professor, Quinnipiac University, Department of
Marketing, School of Business (SB-DNF), Hamden, CT, 06518-
1949, United States,
abhik.roy@quinnipiac.eduMary Schramm
We examine the relationship between direct-to-consumer advertising (DTCA),
and interdependent pricing among firms marketing competing drugs to patients
within the same therapeutic area. We propose that DTCA is a coordinating
mechanism, where a firm signals its willingness to be a Stackelberg price leader by
spending heavily on advertising promoting the drug formulation, not just its own
brand within the category. Propositions are developed about the impact of ad
effectiveness, ad spending and substitutability on the occurrence of a Stackelberg
system. Evidence to support these propositions is provided through empirical
analysis of data from a number of prescription drug categories.
4 - A Dominant Retailer’s Strategic Response To More Efficient
Weak Retailer
Ehsan Bolandifar, Assistant Professor, Chinese University of Hong
Kong, 9/F, Cheng Yu Tung Buliding, No., 12, Chak Cheung Street,
Shatin, N.T., Hong Kong, 999077, Hong Kong,
ehsan@baf.cuhk.edu.hk, Zhong Chen, Fuqiang Zhang
We construct a multi-stage model to study the strategic interaction between
national brand manufacturer, a dominant and weak retailers. We show that more
efficiency on the weak retailer’s end makes the dominant retailer reduce its joint
advertisement level for the national brand and offers lower market prices, while it
also receives a discount form the national brand manufacturer. We also show that
manufacturer does not always benefit from improvement in its operational
efficiency of its retailers. Similarly, dominant retailer does not always benefit from
cheaper store brand procurement costs.
5 - Direct Sales, Agent Selling or Reselling? Firms’ Channel Structure
With Consumers’ Channel Preference
Libo Sun, PhD Student, University of Science and Technology of
China, 96# Jinzhai Road, Hefei, Anhui, PR China, Hefei, 230026,
China,
libosun@mail.ustc.edu.cn, Yugang Yu
Numerous firms exert themselves to adopt multiple channels to sell products.
However, even when facing identical products, consumers’ choices among these
channels could be diverse due to channel preference. We use a stylized theoretical
model to answer two key questions faced by a monopoly manufacturer: (1) how
does consumers channel preference (CCP) affect its channel structure, namely,
when should the manufacturer choose a sin-gle-channel and when should it
adopt a dual-channel instead? Furthermore, should the manufacturer choose an
independent reseller or an agent platform when it intends to leverage external
force? We respectively derive the manufacturer’s optimal channel deci-sions
when consumers show positive and negative CCP to the manufacturer’s direct
channel. We find that: (1) compared with centralized case, the manufacturer
prefers to adopt dual-channel in a larger area in decentralized case; (2) the
manufacturer will choose a dual-channel either when consumers’ CCP to direct
channel is positive or negative enough; (3) the exact forms of dual channel
depends on the thresholds of the agent fee charged by the platform.
MA94
5th Avenue Lobby-MCC
Technology Tutorial: MathWorks/Artelys
Technology Tutorial
1 - MathWorks: Data Analytics With MATLAB
Mary Fenelon, MathWorks, Natick, MA,
United States,
mary.fenelon@mathworks.comMATLAB has evolved to become a platform for predictive and prescriptive
analytics. Engineering, Finance, Data Science, and IT teams are using MATLAB to
build today’s advanced analytics systems ranging from risk analysis to predictive
maintenance and telematics to advanced driver assistance systems and sensor
analytics. Join us to see how MATLAB can help you: • Access, explore, and
analyze data stored in files, on the web, and from data warehouses • Clean,
explore, visualize, and combine complex multivariate data sets • Prototype, test,
and refine predictive models using machine learning methods • Build and solve
prescriptive models and analyze results • Share your results with others We’ll
highlight our newest features for Big Data, machine learning, deep learning, and
optimization through examples such as load forecasting, Monte Carlo simulation,
predictive maintenance, and embedded sensor analytics.
2 - Artelys: Solving Large Least-Squares Models With The Artelys
Knitro Nonlinear Optimization Solver
Richard Waltz, Artelys, 150 N Michigan Avenue, Suite 800,
Chicago, IL, 60601, United States,
Richard.waltz@artelys.comArtelys Knitro is the premier solver for nonlinear optimization problems. This
software demonstration will highlight the latest Knitro developments, including a
new specialized API, as well as enhanced algorithms, for large-scale nonlinear
least-squares models. We will demonstrate how to solve least-squares models
using Knitro through a variety of interfaces such as R, MATLAB and C/C++, and
also provide some benchmarking results. In addition, we will summarize some of
the other recent developments in Knitro.
Monday, 10:00AM - 10:50AM
Monday Plenary
Davidson Ballroom-MCC
Philip McCord Morse Lecture: Margaret L. Brandeau
Plenary Session
Chair: Mike Magazine, University of Cincinnati, Cincinnati, OH
45221-0130,
mike.magazine@uc.edu1 - Public Health Preparedness: Answering (Largely Unanswerable)
Questions With Operations Research
Margaret Brandeau, Stanford University, Stanford, CA,
United States,
brandeau@stanford.eduPublic health security - achieved by effectively preventing, detecting, and
responding to events that affect public health such as bioterrorism, disasters, and
naturally occurring disease outbreaks - is a key aspect of national security.
However, effective public health preparedness depends on answering largely
unanswerable questions. For example: What is the chance of a bioterror attack in
the United States in the next five years? What is the chance of an anthrax attack?
What might be the location and magnitude of such an attack? This talk describes
how OR-based analyses can provide insight into complex public health
preparedness planning problems - and thus support good decisions.
Monday, 11:00AM - 12:30PM
MB01
101A-MCC
Data Mining Under Uncertainty
Sponsored: Data Mining
Sponsored Session
Chair: Erhun Kundakcioglu, Ozyegin University, Nisantepe District
Orman Street / Cekmekoy, Istanbul, 34794, Turkey,
erhun.kundakcioglu@ozyegin.edu.tr1 - Approximation Algorithms For Solving Large-scale
Classification Problems
Neng Fan, University of Arizona,
nfan@email.arizona.eduTo deal the classification of data with uncertainties, the distributionally robust
optimization models are proposed for the support vector machines. First the
problems are reformulated as semidefinite programs or second order cone
programs. To solve these problems on large-scale data sets, we design a stochastic
subgradient algorithm. The numerical experiments will be presented to show the
efficiency of our algorithms.
2 - Margin Maximization Via Benders Decomposition To Solve
Multiple Instance Learning Problems
Emel Seyma Kucukasci, Istanbul Commerce University,
Istanbul, 34840, Turkey,
eskucukasci@ticaret.edu.trEmel Seyma Kucukasci, Bogazici University, Istanbul, 34342,
Turkey,
eskucukasci@ticaret.edu.tr,Mustafa Gokce Baydogan
Multiple instance learning (MIL) aims to solve classification problem where bags
of instances form the input data. Margin maximization model of MIL classification
is a MINLP problem. We develop a Benders decomposition algorithm for MINLP
solution to deal with large datasets. A hybrid approach combining Benders
decomposition and bagging procedure is proposed to test the generalizability of
the results. Computational results on publicly-available molecular activity
prediction, image annotation and text classification datasets are also provided.
MB01