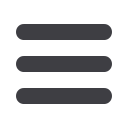

INFORMS Nashville – 2016
153
2 - Decentralized Primal-dual Gradient Method
Soomin Lee, Georgia Institute of Technology, Atlanta, GA,
United States,
soomin.lee@isye.gatech.eduWe present a decentralized primal-dual gradient method for optimizing a class of
finite-sum convex optimization problem whose objective function is given by the
summation of m smooth components together with other relatively simple terms.
The smooth components are distributed over a network of m agents with time-
varying topology, but all agents share common components whose structure is
suitable for efficiently computing the proximal operator. In our method, each
agent alternatively updates its primal and dual estimates by computing the primal
and dual proximal operator, and by communicating these estimates with other
agents in the network. We provide convergence results of this method.
3 - Decomposing Linearly Constrained Nonconvex Problems By
A Proximal Primal Dual Approach
Mangyi Hong, Iowa State University,
mingyi@iastate.eduWe propose a new decomposition approach named the proximal primal dual
algorithm (Prox-PDA) for smooth nonconvex linearly constrained optimization
problems. We show that whenever the penalty parameter in the augmented
Lagrangian is larger than a given threshold, the Prox-PDA converges to the set of
stationary solutions, globally and in a sublinear manner. Interestingly, when
applying a variant of the Prox-PDA to the problem of distributed nonconvex
optimization (over a connected undirected graph), the resulting algorithm
coincides with the popular EXTRA algorithm, which is only known to work in
convex cases.
MB16
105A-MCC
Data-driven and Robust Optimization
Sponsored: Optimization, Optimization Under Uncertainty
Sponsored Session
Chair: Linwei Xin, U of Illinois at Urbana-Champaign, Urbana, IL,
61801, United States,
lxin@illinois.edu1 - Distributionally Robust Stochastic Optimization With
Wasserstein Distance
Rui Gao, Georgia Institute of Technology,
rgao32@gatech.edu,
Anton J Kleywegt
We consider a distributionally robust stochastic optimization (DRSO) problem, in
which the ambiguity set contains all the distributions that are close to the
nominal distribution in terms of Wasserstein distance and satisfies certain
correlation structure. Comparing to the widely-used -divergence and moment
method, Wasserstein distance yields a more reasonable worst-case distribution.
We derive a tractable dual reformulation of the DRSO by constructing the worst-
case distribution explicitly via the first-order optimality condition.
2 - Robust Extreme Event Analysis
Clementine Mottet, Boston University,
cmottet@bu.edu,Henry Lam
We propose a robust optimization approach to estimate extreme event
performance measures. This approach aims to alleviate the issue of model
misspecification encountered by conventional statistical methods that is amplified
by a lack of data typically occurring in the tail region. We demonstrate the use of
shape constraints to mitigate this issue and develop a solution scheme for the
resulting optimizations. We show some numerical results and compare our
approach to extreme value theory.
3 - Data-driven Optimization Of Reward-risk Ratio Measures
Ran Ji, George Mason University, Fairfax, VA, 22030,
United States,
jiran@gwu.edu, Miguel Lejeune
We study a class of distributionally robust optimization problems with ambiguous
expectation constraints on reward-risk ratios. We develop a reformulation and
algorithmic framework based on the Wasserstein metric to model ambiguity and
to derive probabilistic guarantees that the ambiguity set contains the true
probability distribution. The reformulation phase involves the derivation of the
support function of the ambiguity set and the concave conjugate of the ratio
function. We design bisection algorithms to efficiently solve the reformulation.
We specify new ambiguous portfolio optimization models for various ratios.
Computational results will be presented.
4 - Two-stage Distributionally Robust Unit Commitment Using
Moment Information
Yuanyuan Guo, University of Michigan,
yuanyg@umich.eduRuiwei Jiang
As the renewable energy takes a growing share of the electricity markets, a
considerable number of new renewable generators (e.g., wind and solar farms)
are incorporated into daily power system operations. Because of fluctuating
weather conditions or a lack of complete historical data, it can be challenging to
accurately estimate the joint probability distribution of the renewable energy. In
this paper, based on a small amount of historical data, we propose a two-stage
distributionally robust unit commitment model that considers a set of plausible
probability distributions. This model is less conservative than classical robust unit
commitment models.
MB17
105B-MCC
Risk Measures on Stochastic Programs
Sponsored: Optimization, Optimization Under Uncertainty
Sponsored Session
Chair: Saravanan Venkatachalam, Wayne State University,
42 W. Warren Ave, Detroit, MI, 48202, United States,
saravanan.v@wayne.edu1 - A Computational Study Of Recent Approaches To Risk-averse
Stochastic Optimization
Alexander Vinel, Auburn University, 3301 Shelby Center, Auburn,
AL, 36849, United States,
alexander.vinel@auburn.eduWe present a computational study evaluating some recent approaches to risk-
averse stochastic optimization. We focus on the classes of coherent and convex
measures of risk, including higher-moment coherent measures and certainty-
equivalent convex measures. While the bigger part of the study is devoted to
portfolio optimization model, other problems with real-life data are considered.
Our main goal is to evaluate the performance of various recently proposed
techniques and determine the properties that can be used in guiding the specific
choices of decision criteria in practice.
2 - Risk Parity In The Context Of Risk-averse Stochastic Optimization
Nasrin Mohabbati Kalejahi, PhD Student, Auburn University,
Auburn, AL, United States,
nasrin@auburn.edu,Alexander Vinel
The concept of risk parity has been recently studied in the area of financial
portfolio management. The idea behind it is to promote diversification in the
portfolio by ensuring that each asset is equally contributing to the total risk. In
this work we propose to consider risk parity in the context of modern risk
measure theory, by studying risk parity based on conditional value-at-risk and
other coherent measures. We are interested in evaluating the quality of the
decisions that arise from this stochastic optimization framework in both financial
and engineering applications.
3 - Computational Study For Two-stage Stochastic 0-1 Integer
Programs With Absolute Semi Deviation Risk Measure
Saravanan Venkatachalam, Wayne State University,
Saravanan.v@wayne.edu,Lewis Ntaimo
We present a methodology for absolute semi-deviation (ASD) risk-measure for
stochastic 0-1 programs. ASD risk-measure models lack the typical block structure
amenable for decomposition. The proposed methodology uses information from
expected excess, and uses cutting planes based on sub-gradient information.
Computational results for a supply chain application will be presented.
4 - Decomposition For Multistage Stochastic Programs With Quantile
And Deviation Risk Measures
Prasad Parab, PhD Student, Texas A&M University, College Station,
TX, United States,
prasaddparab@tamu.edu, Lewis Ntaimo
We present decomposition for multistage stochastic linear programs (MSLPs) with
quantile and deviation mean-risk measures. Incorporating certain risk measures
makes MSLPs very difficult to decompose and solve. In particular, we study
stochastic decomposition based algorithms for MSLPs with quantile deviation and
absolute semideviation risk measures. A comparative study of the two mean-risk
measures will be presented.
MB17