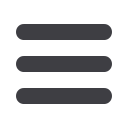

INFORMS Nashville – 2016
152
MB12
104B-MCC
Joint Session APS/Optimization: Advances in Causal
Inference Using Optimization
Sponsored: Optimization, Integer and Discrete Optimization
Sponsored Session
Chair: Nathan Kallus, Cornell University and Cornell Tech, 111 8th
Avenue #302, New York, NY, 10011, United States,
kallus@cornell.eduCo-Chair: Juan Pablo Vielma, Massachusetts Institute of Technology,
Cambridge, MA, United States,
jvielma@mit.eduCo-Chair: Jose R. Zubizarreta, Columbia University, New York, NY,
United States,
zubizarreta@columbia.edu1 - Multivariate Matching Methods For Causal Inference That Are
Balance-variance Pareto Optimal And Optimal Kernel Matching
Nathan Kallus, Assistant Professor, Cornell University and Cornell
Tech, 111 8th Avenue #302, New York, NY, 10011, United States,
kallus@cornell.eduWe present minimax and Bayesian optimality criteria for non-parametric
matching for causal inference. These lead to extensions of existing methods,
including nearest-neighbor (Cochran 1953) and coarsened exact matching (Iacus
et al. 2011), that optimally and automatically adjust balance vis-à-vis matched
sample variance. We develop a new optimal matching method we call optimal
kernel matching (OKM), whose superiority we demonstrate theoretically (optimal
rates) and empirically (with real data). We connect our theory to equal percent
bias reduction (Rubin 1976), which we generalize to non-linear response
functions, showing OKM can achieve uniform error reduction in non-linear
settings.
2 - Large-scale Optimal Matching For Design-based Inference Using
Integer Programming
Jose R. Zubizarreta, Columbia, New York, NY, United States,
zubizarreta@columbia.edu, Juan Pablo Vielma
In observational studies in business research and empirical operations
management, matching methods are often used to approximate the ideal study
that would be conducted if it were possible to do it by controlled
experimentation. In this paper, we present an alternative approach to matching
using integer programming, discuss its theoretical properties, and illustrate its
performance in real-world data sets.
3 - Leveraging Multiple Outcomes In Matched Observational Studies
Colin Fogarty, Massachusetts Institute of Technology, Cambridge,
MA, United States,
colin.b.fogarty@gmail.comWe demonstrate that when performing multiple comparisons in an observational
study, the loss in power from controlling the familywise error rate can, through
the solution of a quadratically constrained linear program, be attenuated when
assessing the robustness of the study’s findings to unmeasured confounding. We
show that this allows for uniform improvements in the power of a sensitivity
analysis both for the overall null across outcomes and for outcome-specific null
hypotheses when compared to combining individual sensitivity analyses. We
illustrate our method through an example examining the impact of smoking on
naphthalene levels in the body.
MB13
104C-MCC
Uncertain Linear Optimization
Sponsored: Optimization, Global Optimization
Sponsored Session
Chair: Jiming Peng, associate professor, University of Houston, 4800
Calhoun Road, Houston, TX, 77204, United States,
jopeng@uh.edu1 - Assessing Systemic Risk In Financial Market Under
Uncertain Liabilities
Jiming Peng, University of Houston,
jopeng@uh.eduWe consider the linear optimization model for assessing the systemic risk in a
financial network where only partial information on the coefficient data matrix in
available. We develop iterative procedures to identify the worst-case and the best-
case. Our theoretical analysis and numerical experiments illustrate that the
potential risk caused by the failure of a single bank in the network is much more
severe than what’s have been estimated in the literature.
2 - Vulnerability Analysis Of Financial Networks
Aein Khabazian, University of Houston,
aeinkhabazian@gmail.comJiming Peng
In this paper, we analyze the vulnerability of a financial network based on the
linear optimization model introduced by Eisenberg and Noe (2001), where the
right hand side of the constraints is subject to market shock and only partial
information regarding the liability matrix is revealed. We conduct a new
sensitivity analysis to characterize the conditions under which a single bank is
solvent, default or bankrupted, and estimate the probability that some financial
institute in the network will be bankrupted under mild assumptions on the
market shock and the network structure. We also present some numerical
experiments to verify the theoretical conclusions in the paper.
3 - A Copositive Perspective On Two-stage Adjustable Robust Linear
Programming
Guanglin Xu, University of IOWA,
guanglin-xu@uiowa.eduWe consider a two-stage adjustable robust linear optimization problem in which
the right-hand side is uncertain and belongs to a convex and compact uncertainty
set. We propose a copositive representation for the two-stage problem. We then
provide a tractable inner approximation for the copositive program, which leads
to a better performance compared to the well-known affine-rule policy. We show
the effectiveness of our approach on several numerical examples.
MB14
104D-MCC
Data Analytics
Sponsored: Analytics
Sponsored Session
Chair: Harrison Schramm, CANA Advisors, 1, Pacific Grove, CA, 93950,
United States,
harrison.schramm@gmail.com1 - Robust Non Parametric Tests To Identify Treatment Effects
Noor E. Alam. M.D., Northeastern University,
md.alam@neu.eduWe proposed a number of non-parametric robust testing tools to handle
uncertainty in detecting treatment effect from observational studies data. In this
work, we present an alternative to the standard non-parametric hypothesis tests
by leveraging the power of discrete optimization technique. Its been found that
our tests are robust to the choice of experimenter.
2 - Linear Probability Models And Big Data: Prediction, Inference,
And Selection Bias
Galit Shmueli, National Tsing Hua University, Hsinchu, Taiwan,
galit.shmueli@iss.nthu.edu.tw, Suneel Babu Chatla
Linear probability models (LPM) - linear regression models applied to a binary
outcome - are used in various fields. We perform a simulation study to evaluate
the pros and cons of LPMs compared to logit and probit, especially with Big Data.
We consider common uses of binary outcome models: inference and estimation,
prediction and classification, and selection bias. We find that coefficient directions,
statistical significance and marginal effects yield results similar to logit and probit.
LPM coefficients are consistent up to a multiplicative scalar. For classification and
selection bias, LPM is on par with logit/probit in terms of class separation and
ranking, but lacking for propensities
3 - Managing Brokers For The Sales Of A Complex New Product
Vahideh Abedi, California State University Fullerton, Fullerton,
CA, United States,
vabedi@fullerton.edu, Rahul Bhaskar
Firms introducing a new product typically rely on sales efforts of brokers to
enhance sales. Customers make their purchase decision not only based on the
word of mouth they have received from other customers about the product, but
also based on the collective information received from the brokers. Therefore,
brokers act synergistically to generate sales while competing. We develop an
analytical framework for this sales process and show how it can facilitate
important managerial decision making.
MB15
104E-MCC
Stochastic and First-order Methods for Data Analysis
Invited: Modeling and Methodologies in Big Data
Invited Session
Chair: Guanghui Lan, Gatech, Atlanta, GA, United States,
george.lan@isye.gatech.edu1 - An Optimal Randomized Incremental Gradient Method
Yi Zhou, Georgia Institute of Technology,
yizhou@gatech.eduWe introduce a deterministic primal-dual gradient (PDG) method that can
achieve the optimal black-box iteration complexity for solving finite-sum convex
optimization problems using a primal-dual termination criterion. We also develop
a randomized version (RPDG) method, which needs to compute the gradient of
only one randomly selected smooth component at each iteration, but can possibly
achieve better complexity than PDG in terms of the total number of gradient
evaluations. We also show that the complexity of the RPDG method is not
improvable by developing a new lower complexity bound for a general class of
randomized methods for solving large-scale finite-sum convex optimization
problems.
MB12