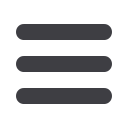

INFORMS Nashville – 2016
148
3 - Response Modeling With Semi-supervised Support
Vector Regression
Dongil Kim, Korea Institute of Industrial Technology, 89
Yangdaegiro-gil, Ipjang-myeon, Seobuk-gu, Cheonan, Korea,
Republic of,
dikim01@kitech.re.kr, Sungzoon Cho
Two-stage response model has been proposed to maximize a profit of a marketing
campaign by estimating the purchase amount of customers. In this paper, we
propose a response modeling with Semi-Supervised Support Vector Regression
(SS-SVR). In SS-SVR, label distributions of unlabeled data are estimated to
consider label uncertainty. Then, training data are generated by oversampling
from the unlabeled data and their estimated label distributions. Finally, a data
selection algorithm is employed to reduce the training complexity. The
experimental results conducted on a real-world marketing dataset showed that
the proposed method improved the model accuracy and expected profit,
efficiently.
4 - Support Vector Linear Regression With Multiple Instance Data
Ihsan Yanikoglu, Ozyegin University, Istanbul, Turkey,
ihsan.yanikoglu@ozyegin.edu.tr, Erhun Kundakcioglu
We present a Support Vector Regression (SVR) framework for multiple instance
(MI) data, which consists of bags of pattern vectors instead of individual
instances. This setting has interesting applications such as image annotation, drug
activity prediction, and causal inference over time. We provide formulations for
MI regression, prove the problem is NP-hard, propose and compare efficient
heuristics for the problem.
MB02
101B-MCC
Data Mining in Healthcare
Sponsored: Data Mining
Sponsored Session
Chair: Ramin Moghaddass, University of Miami, 1251 Memorial Drive,
MEB 308, Coral Gables, FL, 33146-0630, United States,
ramin@miami.edu1 - A Simple And Direct Projection Approach To Handling
Covariate Shift
Fulton Wang, MIT,
fultonw@mit.eduCovariate shift is commonplace in the healthcare setting - the training population,
for which labelled data is available, often differs in covariate distribution from the
test population, for which predictions must be made. Covariate shift can lower
test prediction accuracy even if the relation of covariates to outcomes is the same
in both populations. While past methods have searched for a subspace in which
the covariates of the two populations are similar, we instead propose a method
that directly finds a subspace with which high test prediction accuracy can be
achieved.
2 - Optimized Risk Scores In Healthcare Applications
Berk Ustun, MIT,
ustunb@mit.edu,Cynthia Rudin
Risk scores are simple models that let users quickly assess risk by adding,
subtracting, and multiplying a few small numbers. These models are widely used
in healthcare, but difficult to create because they need to be risk-calibrated, use
small integer coefficients, and obey operational constraints. We present a new
approach to fit risk scores by solving a discrete optimization problem. We
formulate the risk score problem as a MINLP, and present a cutting-plane
algorithm to recover its optimal solution by solving a MIP. We use our approach
to build optimized risk scores for two healthcare applications: (i) seizure
prediction in the ICU; (ii) ADHD screening.
3 - Making Impact Through Identifying Impactable Members
Margrét Bjarnadóttir, University of Maryland,
margret@rhsmith.umd.eduA large body of research focuses on identifying patients at risk, for example for
hospital readmission, appointment no-shows and declining health. However in
many cases interventions to avoid adverse outcomes prove unsuccessful as
patients may not be impactable, due to health status and/or the social
environment. We introduce the concept of jumpers: patients at risk of adverse
outcomes but who go undetected by traditional case management. We discuss the
application of data mining methods to identify these members in two different
settings: Diabetes management and Medicaid ED use management.
MB03
101C-MCC
Daniel H. Wagner Prize Competition II
Invited: Daniel H. Wagner Prize Competition
Invited Session
Chair: C. Allen Butler, Daniel H Wagner Associates, Inc., 2 Eaton Street,
Hampton, VA, 23669, United States,
Allen.Butler@va.wagner.com1 - Data-driven Optimization For Multi-disciplinary Staffing In Mayo
Clinic Improves Patient Experience
Mutafa Y. Sir, Mayo Clinic, 200 First Street SW, Rochester, MN,
55905, United States,
sir.mustafa@mayo.edu,David M Nestler,
Thomas R. Hellmich, Devashish Das, Micheal J Laughlin,
Michon Dohlman, Kalyan Pasupathy
Emergency Department (ED) patient volumes fluctuate throughout the day
leading to delays. Therefore, it is critical to match the staff capacity to the patient
demand. A data-driven approach applied regression trees to system-generated
data to produce an ideal patient volume representing ED load under optimal
staffing conditions. The ideal patient volume was then used to optimize multi-
disciplinary staffing levels. The new shift design significantly improved several
patient-centered metrics.
2 - Optimizing New Vehicle Inventory At General Motors
Robert Inman, General Motors, 30500 Mound Road, Warren, MI,
48092, United States,
robert.inman@gm.com,Michael Frick,
Thomas Hitchman, Robert Muiter, Jonathan Owen,
Gerald Takasaki
Getting inventory right enables GM to meet customer demand more efficiently.
Optimizing new vehicle inventory has two dimensions: determining how many
vehicles, and determining which vehicle configurations. Knowing the best
aggregate number of vehicles helps manage production and pricing. Knowing the
best mix of vehicles helps dealer ordering. Instead of finding “how many” to
provide a given fill rate, we find the inventory that maximizes aggregate variable
profit. Instead of determining “which vehicles” by simply ranking vehicle
configurations by sales, we apply a practical set-covering approach to span
customer demand.
MB04
101D-MCC
Topics in Power Generation Scheduling
Sponsored: Energy, Natural Res & the Environment,
Energy I Electricity
Sponsored Session
Chair: Yongpei Guan, University of Florida, 303 Weil Hall,
Gainesville, FL, 32611, United States,
guan@ise.ufl.edu1 - Stochastic Scheduling For Large Scale Wind Integrated
Power Systems
Lixin Tang, The Institute of Industrial Engineering and Logistics
Optimization, Northeastern University, Insititue of Industrial
Engineering and, Logistics Optimization, Northeastern, Shenyang,
110004, China,
lixintang@mail.neu.edu.cn, Jin Lang
We propose a stochastic optimization problem which takes into account the
volatility of large scale wind power integrated power systems. A scenario
generation method which contains the information of forecast error distribution
and fluctuation distribution for short-term wind power is proposed. The problem
is formulated as a MINLP model. A Lagrangian relaxation algorithm is developed
to solve the model.
2 - A Decomposition Approach For Hydropower Operation And
Maintenance Scheduling
Miguel F. Anjos, Professor and Canada Research Chair,
GERAD & Polytechnique Montreal, Montreal, QC, Canada,
miguel-f.anjos@polymtl.ca, Jesus A. Rodriguez, Pascal Côté,
Charles Audet
The generator maintenance scheduling problem is of great importance for power
generation companies not only to prevent costly generator breakdowns, but also
because of the impact of planned outages on the system operation. In
hydroelectricity generation, the solution of this scheduling problem is complicated
by uncertain water inflows, non-linear relationships between physical variables,
and multiple interdependencies in space and time. Since the simultaneous
solution of the hydro maintenance and operation problem is hard to achieve, we
propose a linearized formulation and a decomposition approach for this problem.
This work is based on the real case of Rio Tinto Aluminium in Canada.
MB02