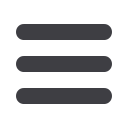

INFORMS Nashville – 2016
163
MB45
209A-MCC
Advances in Simulation Optimization
Sponsored: Simulation
Sponsored Session
Chair: Weiwei Chen, Assistant Professor, Rutgers Business Schoo
l, 1 Washington Park, Newark, NJ, 07901, United States,
wchen@business.rutgers.eduCo-Chair: Siyang Gao, City University of Hong Kong, City University of
Hong Kong, Kowloon, Hong Kong,
siyangao@cityu.edu.hk1 - Generalized Likelihood Ratio Method For Stochastic Derivative
Estimation
Yijie Peng, Fudan University,
pengy10@fudan.edu.cn,Michael Fu,
Jianqiang Hu
We propose a generalized likelihood ratio method for stochastic derivative
estimation in a general framework that can handle discontinuities in both the
sample performance and sample path. The classical likelihood ratio method is a
special case where the parameter does not appear in the sample performance. In
addition, the new method generalizes the push-out likelihood ratio method. The
framework also includes most settings where infinitesimal perturbation analysis
applies, although the actual estimator differs in general. Examples demonstrate
the proposed method works for a broad set of applications, many of which cannot
be handled by existing methods.
2 - Advanced Simulation Optimization Approach
Loo Hay Lee, National University of Singapore,
iseleelh@nus.edu.sg,Chun-Hung Chen
In this talk, we will present some potential research topics in simulation
optimization and discuss some of the preliminary work.
3 - Challenges In Applying Ranking And Selection After Search
David Eckman, Cornell University, Ithaca, NY, United States,
dje88@cornell.edu, Shane Henderson
It is often appealing to reuse simulation replications taken during a simulation
optimization search as input into a ranking-and-selection procedure. However,
even when replications are i.i.d. and independent across systems, we show that
when the search uses the observed performance of explored systems to identify
new systems, conducting ranking-and-selection procedures that reuse the search
replications can result in probabilities of correct (and good) selection below the
prespecified level. We also show a similar deterioration in the guarantees of
subset-selection procedures.
4 - A Partition-based Random Search For Stochastic Constrained
Optimization Via Simulation
Weiwei Chen, Rutgers Business School,
wchen@business.rutgers.edu, Siyang Gao
This research focuses on the global optimization over finite solution space with
deterministic objective function and stochastic constraints. Due to the random
noise observed in the constraints, the feasibility of a solution is unknown and can
be best evaluated by simulation. We propose a partitioning scheme to explore the
solution space and develop a feasibility detection procedure for the sampled
solutions. A partition-based random search approach with multi-constraint
feasibility detection (PRS-MFD) is then proposed to search for the optimal
solution. The efficiency of PRS-MFD is shown by numerical experiments, and it is
proved to converge to the set of global optima with probability one.
MB46
209B-MCC
Revenue Management and Assortment Optimization
Sponsored: Revenue Management & Pricing”
Sponsored Session
Chair: Hamid Nazerzadeh, University of Southern California,
Marshall School of Business, Los Angeles, CA, 90089, United States,
nazerzad@marshall.usc.edu1 - Real-time Optimization Of Personalized Assortments
Negin Golrezaei, University of Southern California,
golrezae@usc.eduMotivated by the availability of real-time data on customer characteristics, we
consider the problem of personalizing the assortment of products for each arriving
customer. Using actual sales data from an online retailer, we demonstrate that
personalization based on each customer’s location can lead to over 10%
improvements in revenue compared to a policy that treats all customers the same.
We propose a family of index-based policies that effectively coordinate the real-
time assortment decisions with the back-end supply chain constraints. We allow
the demand process to be arbitrary and prove that our algorithms achieve an
optimal competitive ratio.
2 - Online Personalized Resource Allocation With Customer Choice
Van-Anh Truong, Columbia University, New York, NY, United
States,
vt2196@columbia.edu, Guillermo Gallego, Anran Li,
Xinshang Wang
We introduce a general model of resource allocation with customer choice. This
problem has a number of applications, including personalized assortment
optimization, revenue management of parallel flights, and web- and mobile-based
appointment scheduling. We derive online algorithms that are asymptotically
optimal and achieve the best constant relative performance guarantees for this
class of problems.
3 - Assortment Personalization In High Dimension
Madeleine Udell, Cornell University, Ithaca, NY, United States,
udell@cornell.edu, Nathan Kallus
We show how to perform assortment personalization in sublinear time by
imposing a natural low rank structure on the problem. In the static setting, we
show that this model can be efficiently learned from surprisingly few interactions.
In the dynamic setting, we show that structure-aware dynamic assortment
personalization can have regret that is an order of magnitude smaller than
structure-ignorant approaches.
4 - Position Auctions With Search Cost
Heng Zhang, University of Southern California,
Heng.Zhang.2019@marshall.usc.edu, Leon Yang Chu,
Hamid Nazerzadeh
Companies such as eBay, Amazon, and Google have created e-commerce
platforms that connect online sellers and online users. In this work, we study how
these platforms should rank the products displayed to their users. We present a
general model that captures several important aspects of these environments
including consumer’s search cost. Our analysis highlights the inefficiencies that
can be created due to the asymmetry of information among the sellers and the
platform. We present an optimal mechanism as well as a simple near-optimal
heuristic.
MB47
209C-MCC
Multi-product Revenue Management
Sponsored: Revenue Management & Pricing
Sponsored Session
Chair: David Simchi-Levi, Massachusetts Institute of Technology,
Cambridge, MA, United States,
dslevi@mit.eduCo-Chair: He Wang, MIT, 77 Massachusetts Ave, Cambrdige, MA,
02139, United States,
wanghe@mit.edu1 - Reaping The Benefits Of Bundling Under High Production Costs
Will Ma, MIT,
willma353@gmail.com,David Simchi-Levi
It has long been known that selling different goods in a single bundle can
significantly increase revenue, but that this is no longer the case if the goods have
high production costs. We introduce a simple pricing scheme, called Pure
Bundling with Disposal for Cost (PBDC), that captures the benefits of bundling
under high costs, extracting all of the surplus in settings where previous simple
mechanisms could not. We also prove a theoretical guarantee on the performance
of PBDC that holds for arbitrary independent valuation distributions, by adopting
and improving techniques from mechanism design literature. Finally, we perform
extensive numerical experiments which support the efficacy of PBDC.
2 - New Algorithms And Guarantees For Assortment Optimization
Under General Choice
Clark Pixton, MIT, Cambridge, MA, United States,
cpixton@mit.edu, David Simchi-Levi
We present new algorithms for static assortment optimization which apply to
general choice models. We show theoretical guarantees, and demonstrate
performance via computational experiments. These algorithms sit between the
work of Jagabathula (2016) and the choice model assortment optimization
literature.
MB47