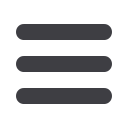

INFORMS Nashville – 2016
164
3 - Using Early Click Information In Online Flash Sales Campaigns
Stefano Nasini, Assistant Professor, IESEG School of Management,
Lille/Paris, France,
s.nasini@ieseg.fr, Victor Martínez de Albéniz,
Arnau Planas
In online flash sales, products are heavily discounted during very short sales
periods. There is significant uncertainty over product sales, that can be reduced
using the chain of sequential decisions that customers take in the website. We
build a statistical model based on four layers of conditional probabilities: from (1)
clicks to the main webpage to (2) clicks to a particular campaign to (3) request for
information of a specific product to (4) final purchase decision. We use
information from clicks occurring in the first hours of a campaign to reoptimize
prices. We finally test our model with real data.
4 - Offline Assortment Optimization In The Presence Of An
Online Channel
Srikanth Jagabathula, NYU Stern School of Business, New York,
NY, United States,
sjagabat@stern.nyu.edu,Daria Dzyabura
Firms are increasingly selling through both offline and online channels. The
offline offerings allow the customers to physically evaluate the products and, as a
result, impact the demand in both channels. Given this, we address how firms
should select an offline assortment to maximize profits across both channels; we
call this the showcase decision problem. We introduce a new model to
incorporate the impact of physical evaluation on consumer preferences. We
validate this model using a conjoint study; propose algorithms, with
approximation guarantees, to determine the profit/sales maximizing assortments;
and demonstrate up to 40% improvement in profits on real-world data.
MB48
210-MCC
Marketing Insights from Social Media
Invited: Social Media Analytics
Invited Session
Chair: David A. Schweidel, Emory University, 1300 Clifton Road NE,
Atlanta, GA, 30322, United States,
dschweidel@emory.edu1 - Is All That Twitters Gold? Effects Of Online Chatter On Stock
Market Returns And Stock Market Volatility
Abhishek Borah, University of Washington,
abhi7@uw.eduThis study uses natural language processing to extract various dimensions across
different sources of Tweets and ascertain their importance. The authors evaluate
the effect of Twitter on both 1) Stock Market Returns using a Multivariate
Dynamic Descriptive Panel Data Model, and 2) Stock Market Volatility using a
Multivariate GARCH model. The authors find that 1) Tweets predict stock market
returns and volatility in stock returns 2) Sentiment is the most important
dimension and spillover effects between volatility in tweets and stock returns
differ in sign depending on the sentiment of tweets, and 3) Firms’ new product
announcements affect tweets.
2 - Social TV, Advertising, And Sales
Beth L. Fossen, Kelley School of Business, Indiana University,
Bloomington, IN, United States,
bfossen@indiana.eduDavid A Schweidel
The rapid growth of social TV - defined as the integration of social media with
television programming - has outpaced the field’s understanding of how
marketers can extract value out of such activities. In this research, we explore the
relationship between social TV, television advertising, and sales by investigating
how viewer engagement with television programs and advertisements impacts
online shopping behavior. This work aims to address (1) if online chatter about
television advertisements spurs sales for the advertised brand and (2) whether
television programs with high online social activity are more beneficial to
advertisers.
3 - Modeling Latent Homophily In Large-scale Social Networks:
A Markov Random Field Approach
Liye Ma, University of Maryland,
liyema@rhsmith.umd.eduThe rapid growth of social media platforms makes large scale social network data
commonplace. Inferring consumer preference and developing targeting strategies
using such data, however, remain challenging. In this study, we introduce a
modeling technique, Gaussian Markov Random Fields (GMRF), to model the
latent homophily of connected consumers. We show that GMRF can be applied to
networks of arbitrary topology, that its conditional independence property is
conceptually appealing, and that model parameters have intuitive interpretations.
We analyze different model configurations incorporating one or more GMRFs,
and demonstrate its application using a mobile network dataset.
4 - Deriving Brand Insights With Social Media Analytics
David A Schweidel, Emory University,
dschweidel@emory.eduInterest in social media continues to grow. While much research has focused on
the use of social media as a communication platform, limited work has probed the
viability of social media data as a source of marketing insights. In this research,
we examine ways in which brands may benefit from the analysis of social media
data. We consider two specific applications: assessing brand health and identifying
shifts in online word of mouth.
MB49
211-MCC
Predicting Business Outcomes Using Social Media
Invited: Social Media Analytics
Invited Session
Chair: Youran Fu, University of Pennsylvania, Philadelphia, PA,
United States,
youranfu@wharton.upenn.edu1 - The Operational Value Of Social Media Information
Ruomeng Cui, Kelley School of Business, Indiana University,
cuir@indiana.edu,Santiago Gallino, Antonio Moreno-Garcia,
Dennis Zhang
We empirically explore how social media information helps sales forecasting.
Using daily sales data from an online apparel company and publicly available
Facebook posts (users’ comments and likes data), we apply various machine
learning methods and find a statistically significant improvement in sales
forecasts.
2 - Stock Market Movement Prediction Using Disparate Data
Sources: A Probabilistic Prediction Model
Bin Weng, Auburn Univeristy, Auburn, AL, 36849,
United States,
bzw0018@auburn.edu, Hamidreza Dolatsara,
Fadel Mounir Megahed
The stock market prediction has attracted much attention from academia as well
as business. In recent years, social media and Internet search behavior are
considered as new sources that affect human’s behavior and decision-making. The
purpose of this study is to develop a probabilistic model to predict short-term
stock movement by comparing machine learning methods using disparate data
sources. This study not only uses traditional historic market data but also the data
from technical analysis, social media, and the internet. Finally, a stock prediction
tool with machine learning methods incorporated has been developed for
predicting the stock’s short-term movement with high accuracy.
3 - The Value Of Social Media Data In Color Trends Forecasting
Youran Fu, The Wharton School, University of Pennsylvania,
Philadelphia, PA, 19104, United States,
youranfu@wharton.upenn.edu,Marshall L Fisher
We partnered with a leading apparel retailer to investigate how to use social
media data to improve fashion color trend forecasting. We find that using fine-
grained Twitter data and a Google search volume index to predict product-color
sales three months out can significantly reduce forecast error compared to
conventional methods.
4 - Understanding The Role And Impact Of Discussions On The
Quality Of User Generated Content – The Case Of Wikipedia.
Srikar Velichety, University of Arizona,
srikarv@email.arizona.eduWe investigate the impact of discussions on the quality of peer-produced content.
Using a data science approach on the complete population of English language
articles in Wikipedia, we demonstrate the predictive and explanatory power of
discussions in article quality. We also compare and contrast the value of
discussion characteristics with the article characteristics.
Our results show that discussions add value to the predictive model by increasing
both the precision and recall. On the explanatory side, we find that discussions
drive both edits and diverse edits leading to better quality. Implications for theory
building and policy setting in peer-production environments are discussed.
MB48