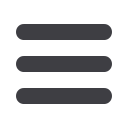

INFORMS Nashville – 2016
197
2 - When Do Bidders Anticipate Regret During Auctions?
Empirical Evidence From Ebay
A. Serdar Simsek, The University of Texas at Dallas, Richardson,
TX, United States,
Serdar.Simsek@utdallas.edu, Ozalp Ozer,
Meisam Hejazi Nia
We developed a structural model that accounts for bidders’ learning and their
anticipation of winner and loser regrets in auctions. Using a large data set from
eBay, we quantify in which product categories bidders anticipate regret and show
how our results can be used to increase eBay’s revenue significantly
3 - Optimal Pricing In Social Networks Under Asymmetric Information
Yang Zhang, Tsinghua University,
yangzhanguser@mail.tsinghua.edu.cn, Ying-ju Chen
We study the optimal pricing of products / services in social networks, where
customers are strategic and their consumptions exhibit local externality. Our
model concerns the information asymmetry — Consumers know about their local
network characteristics while the selling firm has only knowledge of global
network. The network model we employ embeds random network and scale free
network as special cases. We characterize the optimal menu for the firm, the
induced sales, and their properties with regard to the network structure.
MC43
208A-MCC
Decision Analysis Arcade I
Sponsored: Decision Analysis
Sponsored Session
Chair: Joshua Woodruff, University of Texas, Austin, TX, United States,
joshua.woodruff@utexas.edu1 - Assessment Of Drug Development Options: If, When And
How Much?
Ozgur Ozkan, Decision Science Director, Astrazeneca
Pharmaceuticals, Gaithersburg, MD, United States,
Ozgur.Ozkan@astrazeneca.comNew approaches in clinical trial design and changing regulatory and payer
environment make it a challenging task to compare different drug development
paths. We will describe a modeling approach to assess different options in time,
risk and value dimensions. This will cover uncertainties around success measures,
clinical trial timelines and market share expectations. Ideas of eliciting
information from subject matter experts as well as combining expert opinion with
statistical estimates will be shared. The talk will also reflect on our experience of
utilizing different metrics and visualizations to communicate with stakeholders.
2 - Optimal Discretization For Decision Analysis
Joshua Woodruff, University of Texas,
joshua.woodruff@utexas.eduOptimal discretization is a new method to discretize uncertainties. By using
optimization techniques to discretize uncertainties, it is possible to create robust
discretizations that are more accurate. Our method produces more accurate
project certain equivalents which will improve decision quality. We use non-
linear optimization to select and assign probabilities to candidate percentiles for
each model uncertainty. With optimal discretization we found we can use the
model information and potential distributions of the uncertainties to find
discretizations that yield certain equivalent errors that can be orders of magnitude
better than other discretization methods we tested.
3 - Network Interdiction In Competitive Market Entry And
Product Design
Benjamin Harris, Northeastern University, 360 Huntington
Avenue, Boston, MA, 02115, United States,
harris.be@husky.neu.edu,Sagar S Kamarthi
Optimal strategy for product design in the Internet of Things (IoT) must consider
input beyond that of stakeholders and customers and include the highly
connected infrastructure on which the product will be released. Firms involved in
the IoT need to develop strategy and risk mitigation techniques and remain
competitive. This research will enable firms to identify optimal strategies under
current requirement and market conditions, as well as analyze changes in strategy
if a requirements space is changed. As a result, product designers and firm
leadership can anticipate and respond to market and industrial changes with
increased fidelity and predictive power through network model insights.
MC44
208B-MCC
Panel: Howard Raiffa: Celebration of His Life
and Achievement
Sponsored: Decision Analysis
Sponsored Session
Moderator: Jeffrey Keisler, University of Massachusetts - Boston,
100 Morrissey Boulevard, Boston, MA, 02125, United States,
jeff.keisler@umb.edu1 - Howard Raiffa: Celebration Of His Life And Achievement
Jeffrey Keisler, University of Massachusetts, Boston, MA,
United States,
jeff.keisler@umb.eduColleagues of Howard Raiffa will discuss aspects of his life, contributions and
legacy.
2 - Panelist
David Bell, Harvard Business School, Morgan Hall 171, Boston, MA,
02163, United States,
dbell@hbs.edu3 - Panelist
Ralph Keeney, Duke University, Fuqua School of Business,
San Francisco, CA, 94111, United States,
keeneyr@aol.com4 - Panelist
Detlof Von Winterfeldt, University of Southern California, USC,
Los angeles, CA, 90089, United States,
winterfe@usc.eduMC45
209A-MCC
Optimization via Simulation under Input Uncertainty
Sponsored: Simulation
Sponsored Session
Chair: Eunhye Song, Northwestern University,
2145 Sheridan Road, Evanston, IL, 60208, United States,
eunhyesong2016@u.northwestern.edu1 - Data-driven Construction Of Uncertainty Sets For Joint Chance-
constrained Programs
Jeff Hong, City University of Hong Kong,
jeffhong@cityu.edu.hkHenry Lam, Zhiyuan Huang
We study the use of robust optimization (RO) in approximating joint chance-
constrained programs (CCP), in situations where only limited data, or Monte
Carlo samples, are available in inferring the underlying probability distributions.
We introduce a procedure to construct uncertainty set in the RO problem that
translates into provable statistical guarantees for the joint CCP. This procedure
relies on learning the high probability region of the data and controlling the
region’s size via a reformulation as quantile estimation. We show some
encouraging numerical results.
2 - Asymptotics Of Risk Formulations For Simulation Optimization
Under Input Uncertainty
Di Wu, Georgia Institute of Technology, Atlanta, GA, United States,
dwu80@gatech.edu,Enlu Zhou
Input distributions are the distributions (of stochastic uncertainty) that drive a
simulation process. Since input distributions are usually estimated from finite
data, optimizing the model may yield solutions that perform poorly under the
true input distributions. To hedge against the risk of input uncertainty, we
minimize the risk measures of mean output with respect to the unknown
parameters’ posterior distribution. We establish the consistency and the
asymptotic normality of risk formulations, and show that when the input data has
a small size, the risk formulations are essentially seeking a tradeoff between
average performance and the risk of actual performance.
3 - An Optimization-based Approach To Input Uncertainty Via The
Empirical Likelihood
Henry Lam, University of Michigan,
khlam@umich.eduHuajie Qian
We study a simulation-optimization-based approach in constructing statistically
accurate confidence bounds for stochastic simulation under nonparametric input
uncertainty. This approach utilizes the empirical likelihood method that converts
the computation of confidence bounds into a pair of optimizations over the
uncertain input distributions, with a suitable weighted-average divergence
constraint calibrated with a chi-square quantile. We present the theory giving rise
to the constraint and the calibration, and compare the performance of our
optimization algorithm with existing standard methods such as the bootstrap.
MC45