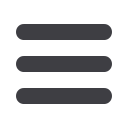

INFORMS Nashville – 2016
199
MC48
210-MCC
New Insights from Social Media: Empirical Study,
Field Experiment, and Algorithm Development
Invited: Social Media Analytics
Invited Session
Chair: Yen-Yao Wang, Michigan State University,
632 Bogue Street, Room N204, Okemos, MI, 48824, United States,
wangyen@broad.msu.edu1 - Social Media Engagement, Product Evaluations, And Demand
Spillover In The Automobile Industry
Yen-Yao Wang, Michigan State University,
wangyen@broad.msu.edu, Anjana Susarla, Roger Calantone,
Yingda Lu
Online Word of Mouth (WOM) is an important aspect of consumer-firm
relationship and a leading indicator of product performance. However, prior
research focuses considerably on the static view of it. This paper examines the
dynamics of the spillover effects in online WOM in the US automobile industry.
To measure online WOM, we focus specifically on customers’ test drive
experience. We collected data from around 1000 different social media platforms
for 32 car brands from 2009 to 2015. We used a Bayesian modeling framework
and estimated the model using Markov Chain Monte Carlo (MCMC) methods.
2 - The Dark Side Of Positive Social Influence
Che-Wei Liu, University of Maryland, College Park, MD, Un
ited States,
cwliu@rhsmith.umd.edu, Ritu Agarwal,
Guodong (Gordon) Gao
Social influence has been widely used to transform behaviors. However, when
individuals are pressured to conform to behavior of the majority, would it lead to
an unexpected backfire effect? We conducted a randomized field experiment of
more than 10,000 individuals for a two-month period on an online physical
activity community to examine the dark side of social influence. We studied the
effect of social norms on users’ goal setting and goal achievement behavior. While
social influence increased the rate of goal setting, strikingly, we also observe the
dark side of social influence. Our findings have important implications for the
design of interventions in the context of mHealth technologies.
3 - Influencers In Social Media – An Assessment Of Algorithmic
Approaches In Big Data Environments
Shih-Hui Hsiao, Lawrence Technological University,
Southfield, MI, United States,
suade0904@msn.com, Ram Pakath
Growing social media usage, accompanied by explosive growth in related Big
Data, has resulted in increasing interest in finding automated ways of discovering
influencers (i.e., opinion leaders) in online social interactions. Beginning 2008,
about two dozen variants of six basic approaches have been proposed. Yet, there
is no comprehensive study investigating the relative efficacy of these methods in
specific settings. We investigate and report on the relative performances of 15
methods on 9 twitter data sets ranging in size from tens of thousands to hundreds
of thousands of tweets.
4 - Natural Language Processing: From Text Mining To
Social Media Analysis
Chih-Hao Ku, Assistant Professor, Lawrence Technological
University, 21000 W 10 Mile Rd, M309A, Southfield, MI, 48331,
United States,
cku@ltu.edu,Yung-Chun Chang
Today, the number of digital reports, e.g., crime reports and social media data
derived from Twitter, LinkedIn, and Facebook are growing rapidly. However, this
immerse amount of digital data post challenges and opportunities for data
analysis. The rise of social media has drawn interest on text mining and social
media analysis, e.g., sentiment analysis. Natural language processing (NLP) is an
important component for those analyses. We report here on research work on
text mining, sentiment analysis, and future trend using NLP techniques.
MC49
211-MCC
Estimating Sentiments Using Social Media
Invited: Social Media Analytics
Invited Session
Chair: Subodha Kumar, Mays Business School, Texas A&M University,
301-F, Wehner, 4217 TAMU, Mays Business School, College Station,
TX, 77843, United States,
skumar@mays.tamu.edu1 - The Effects Of Social Media Sentiment On Engagement
Rakesh Reddy Mallipeddi, Mays Business School, Texas A&M
University, College Station, TX, United States,
rmallipeddi@mays.tamu.edu,Ramkumar Janakiraman,
Subodha Kumar, Seema Gupta
In this study, we propose an econometric model to examine the drivers of social
media engagement. Set in the context of national elections, we examine the
impacts of tweeting behavior of the candidates contesting in an election on the
social media engagement with their constituents. To meet our objectives, we
assemble a novel candidate-level data of social media engagement.
2 - The Effect Of “online following” On Contributions To Open Source
Communities
Xiaowei Mei, University of Florida,
xiaowei.mei@warrington.ufl.edu, Mahdi Moqri, Liangfei Qiu,
Subhajyoti Bandyopadhyay
We estimate the effect of “online following,” a basic form of online social
interaction, on members’ contributions in open source software (OSS)
communities. We employ a panel vector autoregression model using individual
level data across time in GitHub to achieve identification of causal effects, while
controlling for individual heterogeneity and time effects. We find that the
following behavior of others has a significant positive effect on users’ contribution
level to the platform even after controlling for the aforementioned factors. On the
other hand, users’ contribution level also has a significant positive effect on their
following behavior.
3 - Manipulation For Competition: An Empirical Investigation Of
Click Farming
Jingchuan Pu, University of Florida,
jingchuan@ufl.eduLiangfei Qiu, Hsing K Cheng
Anytime there’s a monetary value added to clicks, there’s going to be people
going to the dark side. This research focuses on the economic incentive of the
click farming, a popular form of click fraud which is widely conducted by sellers
or content generators. We cooperate with a website that uses an algorithm to
detect the robot or unreal viewing activity, a black list is built for the suspicious
user account which may be used as click farming. Using the detection results as a
proxy of the content generators’ click farming activity, we test the impact of
potential incentive, like the status of this content, content generator and the
competition environment on the content generators’ click farming activity.
MC51
213-MCC
Pricing and Revenue Management Applications
Sponsored: Manufacturing & Service Oper Mgmt
Sponsored Session
Chair: N. Bora Keskin, Duke University, Durham, NC, United States,
bora.keskin@duke.edu1 - Pricing Reservations: Dealing With No-shows
Jaelynn Oh, University of Utah,
jaelynn.oh@business.utah.eduWe study two remedies to deal with reservation no-shows: charging no-show fees
and price discrimination. We also study how to allocate capacity between
reservations and walk-ins.
2 - Trade Credit And Lifetime Value Of A Newsvendor Buyer
Meisam Soltani-koopa, Queen’s University, Kingston, ON, Canada,
15msk3@queensu.ca,Yuri Levin, Mikhail Nediak,
Anton Ovchinnikov
Trade credit typically appears as a grace period for invoice payment. It helps
retailers overcome temporary cash shortages as an alternative to seeking
financing from banks. We consider a supply chain with one supplier and one
repeated newsvendor retailer where the supplier maximizes its long-term profit
by offering the retailer a financing and wholesale price contract. In each period,
the retailer decides about the quantity to order and the amount of money to
borrow from the supplier or a bank. We study the lifetime value of the retailer
using a dynamic Stackelberg game, from the perspective of the supplier who, as a
leader, takes into account the long-term view of its relationship with the retailer.
3 - Asymptotically Optimal Markdown Policies For Demand Learning
Hongfan Chen, University of Chicago,
hongfan.chen@chicagobooth.edu, N. Bora Keskin
Consider a firm selling a product to a population of customers with
heterogeneous valuations. In this setting, we develop guidelines for designing
markdown policies and derive asymptotically optimal performance guarantees.
(Joint work with Bora Keskin)
MC51