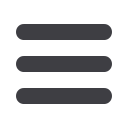

INFORMS Nashville – 2016
200
MC52
214-MCC
Panel: Pro Bono Analytics
Sponsored: Public Sector OR
Sponsored Session
Moderator: David T. Hunt, Oliver Wyman, One University Square,
Princeton, NJ, 08540, United States,
david.hunt@oliverwyman.com1 - Pro Bono Analytics
David T. Hunt, Oliver Wyman, One University Square, Princeton,
NJ, 08540, United States,
david.hunt@oliverwyman.comPro Bono Analytics is an initiative within INFORMS to match members willing to
volunteer their OR and analytical skills with non-profit organizations working in
underserved and developing communities. Panelists include Nashville area non-
profit organizations and Pro Bono Analytics volunteers discussing how analytics
can provide positive impacts for topics ranging from improving operations at a
homeless shelter to understanding the inventory needs for supplies in a low-
income school district.
2 - Panelist:
Matthew Brondum, US Army Corps of Engineers, Vicksburg, MS,
United States,
mcb345@cornell.edu3 - Panelist:
Joel Wright, PENCIL Foundation, Nashville, TN, United States,
jwright@pencilfd.org4 - Panelist:
Cindy Corona Rivera, Hands On Nashville, Nashville, TN,
Cindy@hon.org5 – Panelist:
Anna Danandeh, Verizon, Waltham, MA,
annadanandeh@mail.usf.eduMC53
Music Row 1- Omni
Panel: Emerging Themes in Startup Product,
Supply Chain & Technology Management
Sponsored: Technology, Innovation Management
& Entrepreneurship
Sponsored Session
Moderator: Nitin Joglekar, Boston University Questrom School of
Business, 595 Commonwealth Avenue, Boston, MA, 02215,
United States,
joglekar@bu.edu1 - Panelist:
Nitin Joglekar, Boston University Questrom School of Business,
joglekar@bu.eduThis panel showcases alternative themes and research approaches being pursued
by a select set of emerging scholars in the startup product, supply chain &
technology management research domain.
2 - Panelist:
Jennifer Bailey, Babson College,
jbailey@babson.edu3 - Panelist:
Jianxi Luo, Singapore University of Technology & Design,
luo@sutd.edu.sg4 - Panelist:
Joel Wooten, University of South Carolina,
joel.wooten@moore.sc.edu5 - Panelist:
Onesun Steve Yoo, University College London,
onesun.yoo@ucl.ac.uk6 - Panelist:
Meyyappan Narayanan, Lakehead University, Thunder Bay, ON,
Canada,
meyyappan.narayanan@lakeheadu.caMC54
Music Row 2- Omni
Service Innovation in the Cognitive Era
Invited: Service Science
Invited Session
Chair: Changrui Ren, IBM Research - China, IBM Research - China,
Beijing, 100193, China,
rencr@cn.ibm.com1 - Enterprise Cloud Garbage Collector
Sai Zeng, IBM T.J. Watson Research Center, Yorktown Heights, NY,
United States,
saizeng@us.ibm.com,Christopher Young,
Karin Murthy
Infrastructure as a Service (IaaS) clouds empowers the agility to provision servers.
Recent findings indicate that this agility led to a situation where 1 in 3 data center
servers is a zombie server, aka server is running but does not do any useful work.
In this paper, we present Enterprise Cloud Garbage Collector, a tool that detects
zombie severs. It establishes dependency between users/clients and servers by
constructing a weighted reference model based on application knowledge. In the
situation of insufficient application knowledge, it supplements its dependency
results with a machine learning model trained on resource utilization data.
2 - Big Data Fueled Supply Risk Management: Sensing, Prediction,
Evaluation And Mitigation
Changrui Ren, IBM Research - China, Beijing, China,
rencr@cn.ibm.com, Miao He, Qinhua Wang
Supplier risks jeopardize on-time or complete delivery of supply in a supply
chain. This talk will introduce a big data fueled approach to monitor and manage
supply risks, which includes a big data analytics component, a simulation
component and an optimization component. The big data analytics component
senses and predicts supply disruptions with internally (operational) and external
(environmental) data. The simulation component supports risk evaluation to
convert predicted risk severity to key performance indices (KPIs) such as cost and
stockout percentage. The optimization component assists the risk-hedging
decision-making.
MC55
Music Row 3- Omni
Inventory Management II
Contributed Session
Chair: Heinrich Kuhn, Catholic University of Eichstaett-Ingolstadt, Auf
der Schanz 49, Ingolstadt, 85049, Germany,
heinrich.kuhn@ku.de1 - Base-stock Models For Lost Sales - A Markovian Approach
Sang-Phil Kim, Assistant Professor, Winona State University, 175
W. Mark st., Somsen 406, Winona, MN, 55987, United States,
Ksphil@me.com, Yanyi Xu, Maqbool Dada, Arnab Bisi,
Suresh Chand
We consider the lost sales model with discrete demand. The inventory is reviewed
every T periods and an order is placed to bring the inventory position back to the
target base-stock level R, and is received after a lead time of L periods. Based on
the outstanding orders in the pipeline, we represent the state of the system as a
Markov chain. We show that the structure of the transition probability matrix is
recursive in R and L. This special structure is used to facilitate computation of the
stationary distribution. Analytical results complemented by numerical examples
reveal that neither the optimal base-stock nor the expected cost is monotone in L
for a given T.
2 - Capacity Usage Estimation Methodology For
Inventory Management
Ahmet Nuroglu, Yildiz Technical University, Barbaros Bulvari,
Yildiz-Istanbul, 34349, Turkey,
envernuroglu@gmail.com,
Fahrettin Eldemir
New analytical capacity usage estimation methodology for economic order
quantity (EOQ) model is proposed. In multiple item warehouse-space capacity
constrained EOQ model, by applying the randomized storage concept, capacity
usage is estimated from expected inventory occurrences instead of order
quantities. In joint replenishment problem under power of two (PoT) policy, the
capacity usage is estimated from average inventory occurrences which are the
function of PoT parameter of each item. The feasible optimal solutions are
simulated and validated.
MC52