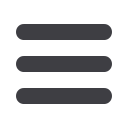

INFORMS Nashville – 2016
302
Tuesday, 1:30PM - 3:00PM
TC01
101A-MCC
Ensemble Methods in Data Mining
Sponsored: Data Mining
Sponsored Session
Chair: Waldyn Martinez, Miami University, 117 Country Club Dr.,
Oxford, OH, 45056, United States,
wmartine@cba.ua.edu1 - Ensemble Methods For Credit Risk Assessment
Youqin Pan, Salem State University, Bertolon School of Business,
352 Lafayette Street, Salem, MA, 01970, United States,
ypan@salemstate.eduMore and more banks and financial institutions have started to pay more
attention on credit risk due to the recent financial crisis. This paper aims at
improving predictive powers of the credit score models using bagging and
boosting algorithms.
2 - Multi-engine Out-of-sample Boosting
Meinolf Sellmann, Senior Manager, IBM Research,
1101 Kitchawan Rd, Yorktown Heights, NY, 10566, United States,
meinolf@us.ibm.comWe present a new machine learning method that combines ensemble learning
with meta-algorithmics, in particular algorithm portfolios. The result is a method
that automatically determines a collection of predictive models which may or may
not not consider the same concept class. To avoid over-fitting, these models are
never trained on examples from regions they are later used for, nor do we ever
combine predictions with each other. A portfolio method is used to select one and
only one predictor at runtime, which effectively serves as regularization
technique. Numerical results demonstrate that the new method massively
improves the state of the art in predictive modeling.
3 - Applying Directed Acyclic Graph-based Ensemble Method For
Analyzing Huge And Mixed Data In Mobile Manufacturing
Seonghyeon Kang, Samsung Electrotics, Suwon, Korea, Republic
of,
shyeon.kang@gmail.comIn mobile manufacturing, following the rapidly increasing deployment of sensing
to maintain high productivity and quality, the data that we have to analyze is
growing exponentially. However, in practical approach, constructing the
predictive model is difficult because of the huge size of data and the mixed
datatypes on the training phase. In this study, we propose the efficient ensemble
method to handle those problems in mobile devices manufacturing. The
effectiveness of the proposed method is demonstrated by real data from the
mobile plant in one of the leading mobile companies in South Korea.
4 - Reducing The Complexity Of Ensemble Methods For Use In Large
Scale Multidimensional Data
Waldyn Martinez, Assistant Professor of Business Analytics,
Miami University, 117 Country Club Dr., Oxford, OH, 45056,
United States,
martinwg@miamioh.eduEnsemble models refer to methods that combine a typically large number of fitted
values into a bundled prediction. A key challenge of using ensembles in large-
scale multidimensional data lies in their complexity and the computational
burden they create. Recent research effort in ensembles has concentrated in
reducing ensemble size, while maintaining their predictive accuracy. Here, we
propose a way to reduce the complexity of an ensemble solution by optimizing on
its margin distribution, while reducing their similarity. The proposed method
results in an ensemble that uses only a fraction of the original weak learners, with
improved or similar generalization performance.
TC02
101B-MCC
Data Mining in Medical and Brain Informatics I
Sponsored: Data Mining
Sponsored Session
Chair: Chun-An Chou, SUNY Binghamton, 4400 Vestal Parkway East,
Binghamton, NY, 13902, United States,
cachou@binghamton.eduCo-Chair: Sina Khanmohammadi, SUNY Binghamton, 4400 Vestal
Parkway East, Binghamton, NY, 13902, United States,
skhanmo1@binghamton.edu1 - Artificial Neurons Meet Real Neurons: Pattern Selectivity In V4
Reza Abbasi-Asl, University of California, Berkeley, CA, United
States,
abbasi@berkeley.edu, Yuansi Chen, Adam Bloniarz,
Jack L. Gallant, Bin Yu
Vision in humans and in non-human primates is mediated by a constellation of
hierarchically organized visual areas. One important area is V4 which has highly
nonlinear response properties. To better understand the filtering properties of V4
neurons we recorded from 71 well isolated cells stimulated with 4000-12000
static grayscale natural images. We fit predictive models of neuron spike rates
using transformations of natural images learned by a convolutional neural
network (CNN). Furthermore, we introduce new processes for interpreting such
models. We conclude that the V4 neurons are tuned to a remarkable diversity of
shapes such as curves, blobs, checkerboard patterns, and V1-like gratings.
2 - A New Adaptive Seizure Onset Detection Framework
Sina Khanmohammadi, SUNY Binghamton, 4400 Vestal Pkwy E,
SSIE Department, Binghamton, NY, 13902, United States,
skhanmo1@binghamton.edu, Chun-An Chou
In this study, we present a new adaptive distance-based seizure detection
algorithm that provides comparable performance to more complex seizure onset
detection methods in the literature using much less computational resources. The
proposed framework is validated using CHB-MIT dataset, which is one of the
most comprehensive scalp EEG recordings of pediatric epileptic patients.
3 - Efficient Heuristic For Large Scale Networked Data Classification
Daehan Won, University of Washington,
wondae@uw.eduNetworked data classification is a kind of data classification where each instance is
a constructed by networked structure. Similar to the current data classification, it
involve huge computation time and over fitting results when the input networks
have complicated structure with large size of nodes and links. To overcome those
drawbacks, we present a new mathematical model based on the node selection
scheme and provide a heuristic algorithm to solve the proposed math. model
which is NP-hard. As a demonstration, we provide investigation results based on
the human brain networks as well as simulated data set.
TC03
101C-MCC
Panel: Publication Tips
Sponsored: Junior Faculty JFIG
Sponsored Session
Moderator: Anahita Khojandi, University of Tennessee, Knoxville, TN,
United States,
anahitakhojandi@gmail.com1 - Panel Discussion: Tips For Successful Publication From
Journal Editors
Anahita Khojandi, University of Tennessee, Knoxville, TN,
United States,
khojandi@utk.eduThe panelists consist of past and current editors from top journals, including
Management Science, Operations Research, INFORMS Journal on Computing
and Decision Analysis. The editors will share tips on how to get your paper
successfully published, from selecting the right journal and preparing the
manuscript, to revising the paper and responding to reviewers’ comments. They
will also answer questions pertaining to writing and publication.
2 - Panelist
Alice Smith, Auburn University, 3301 Shelby Center, Auburn, AL,
36849, United States,
smithae@auburn.edu3 - Panelist
Jay Simon, American University, 4400 Massachusetts Avenue, NW,
Washington, DC, 20016, United States,
jaysimon@american.edu4 - Panelist
Alice Smith, Auburn University, Auburn, AL, United States,
smithae@auburn.edu5 - Panelist
Douglas Shier, Clemson University, Clemson, SC, United States,
shierd@clemson.edu6 - Panelist
Serguei Netessine, Insead, Singapore, Singapore,
serguei.netessine@insead.eduTC01