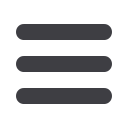

INFORMS Nashville – 2016
307
TC15
104E-MCC
Joint Session AI/SMA: Social Media Analytics and
Big Network Data
Sponsored: Artificial Intelligence
Sponsored Session
Chair: Bin Zhang, Assistant Professor, University of Arizona, 1130 E.
Helen St, Tucson, AZ, 85715, United States,
binzhang@arizona.edu1 - Analyzing Heterogeneity Of User Participation Behavior In Online
News Article Propagation
Devi Bhattacharya, University of Arizona,
dbhattac@email.arizona.eduThe session will illustrate the diversity in users’ article sharing behavior on social
media. The literature on social media based propagation although considerable
generally undercompensates heterogeneity in users’ behavior incidental to the
source or type of content being propagated. In contrast, our research provides
extensive empirical evidence of multiplicity in users’ article sharing behavior by
analyzing 5 different facets of user participation. Our research uses network
theoretic and statistical techniques on a Twitter dataset of 35 news providers
collected over 9 months to provide critical insights essential for modeling users’
news article sharing behavior on social media.
2 - Knowledge Discovery Using Disease Comorbidity Networks
Karthik Srinivasan, University of Arizona, Tucson, AZ, 85719,
United States,
karthiks@email.arizona.edu,Faiz Currim,
Sudha Ram
Comorbidity or disease co-occurrence is the simultaneous presence of two or
more diseases. A disease comorbidity network (DCN) can be constructed based on
repeated evidence of two or more diseases co-occurring in anonymized patient
visit datasets. We report on an empirical study using a large dataset of electronic
health records to explore the characteristics of this network including community
formation and structural measures. Our network analysis is used to reinforce
existing medical knowledge about comorbidity and to reveal previously unknown
comorbidity relationships.
3 - Social Networks In Online Games: The Impact Of Peer Influences
On Repeat Purchase
Bin Zhang, University of Arizona,
binzhang@arizona.edu,Ruibin Geng, Xi Chen
Consumers’ purchase decisions can be affected by both direct and indirect peer
influences. However, there is no existing work about how these two types of
influence impact repeat-purchase of. In this study, we fill such gap by
investigating the interdependent repeat-purchase of online game players
embedded in a social network. We build a new hierarchical Bayesian model that
can support response variable following Poisson distribution and simultaneously
include both types of peer influence, direct and indirect. Our empirical study
yields interesting findings that peer influences have a significant impact on repeat
purchase, but the directions of direct and indirect influences are different.
TC16
105A-MCC
Inverse Optimization: Applications
Sponsored: Optimization, Optimization Under Uncertainty
Sponsored Session
Chair: Taewoo Lee, Rice University, 1, Houston, TX, United States,
taewoo.lee@rice.edu1 - Inverse Optimization For The Analysis Of Competitive Markets:
An Application To The North American Fuel Market
Michael Pavlin, Wilfrid Laurier University,
mpavlin@wlu.caIn this talk we consider conditions under which inverse optimization can be used
to analyze unobservable supply constraints underlying pricing of competitive
markets. We present a study of price differentiation in the North American fuel
market.
2 - Inverse Optimization For Intensity Modulated Proton Therapy
Neal Kaw, University of Toronto, Toronto, ON, Canada,
neal.kaw@mail.utoronto.ca,Timothy Chan, Albin Fredriksson
Robust multiobjective optimization models can be used to determine treatment
plans for intensity-modulated proton therapy (IMPT), which is a method of
cancer therapy. The uncertainty set in such a model is a set of scenarios. In this
work, we propose a novel inverse optimization model to determine objective
weights and a set of scenarios, such that a historical treatment plan is optimal
with respect to a given robust IMPT optimization model. We apply our model to
prostate cancer data.
3 - Data-driven Objective Selection In Multi-objective Optimization:
Inverse Optimization Approach
Taewoo Lee, Rice University, 6100 Main MS-134, Houston, TX,
77005, United States,
taewoo.lee@rice.edu,Tayo Ajayi,
Andrew J Schaefer
Performance of a multi-objective optimization problem largely depends on the
choice of objectives. Currently, objectives are typically chosen in a trial-and-error
manner based on subjective beliefs. We propose an inverse optimization approach
to learn from data and determine a minimal set of objectives for a multi-objective
optimization problem such that the problem generates solutions that are
comparable to the given data. We provide theoretical properties of the
methodology in comparison with regression, and establish a relationship with the
traditional best subset regression approach. We demonstrate the methodology in
cancer therapy applications.
TC17
105B-MCC
Stochastic Optimization for Large-Scale Learning
General Session
Chair: Qihang Lin, the University of Iowa, 21 East Market Street, PBB,
S380, Iowa City, IA, 52245, United States,
qihang-lin@uiowa.edu1 - Adadelay: Delay Adaptive Distributed Stochastic Optimization
Adans Wei Yu, Carnegie Mellon University, Pittsburgh, PA,
United States,
weiyu@cs.cmu.eduWe develop distributed stochastic optimization algorithms under a delayed
gradient model in which server nodes update parameters and worker nodes
compute stochastic (sub)gradients. Our setup is motivated by the behavior of real-
world distributed computation systems; in particular, we analyze a setting
wherein worker nodes can be differently slow at different times. In contrast to
existing approaches, we do not impose a worst-case bound on the delays
experienced but rather allow the updates to be sensitive to the actual delays
experienced. This sensitivity allows use of larger stepsizes, which can help speed
up initial convergence without having to wait too long for slower machines.
2 - Rsg: Beating Subgradient Method Without Smoothness And
Strong Convexity
Tianbao Yang, the University of Iowa,
tianbao-yang@uiowa.edu,Qihang Lin
In this paper, we propose novel deterministic and stochastic {\bf R}estarted {\bf
S}ub{\bf G}radient (RSG) methods that can find an $\epsilon$-optimal solution
for a broad class of non-smooth and non-strongly convex optimization problems
faster than the vanilla deterministic or stochastic subgradient method (SG). We
show that for non-smooth and non-strongly convex optimization, RSG can
reduce the dependence of SG’s iteration complexity on the distance to the optimal
set of the initial solution to that of points on the $\epsilon$-level set. For a family
of non-smooth and non-strongly convex optimization problems whose epigraph
is a polyhedron, we further show that RSG could converge linearly.
3 - Worst-case Complexity Of Cyclic Coordinate Descent
Ruoyu Sun, Stanford University,
ruoyus@stanford.edu,Yinyu Ye
We establish several complexity bounds of cyclic coordinate descent (C-CD) for
quadratic minimization, and prove that these bounds are almost tight. Although
some existing examples showed C-CD can be much slower than randomized
coordinate descent (R-CD), in many practical examples C-CD is faster than R-CD,
making it unclear whether the worst-case complexity of C-CD is better or worse
than R-CD. One of our bounds shows that C-CD can be O(n^2) times slower than
R-CD in the worst case. One difficulty with the analysis of the constructed
example is that the spectral radius of a non-symmetric iteration matrix does not
necessarily constitute a lower bound for the convergence rate.
4 - Homotopy Smoothing For Structured Non-smooth Problems
Qihang Lin, Assistant Professor, University of Iowa, Iowa City, IA,
52242, United States,
qihang-lin@uiowa.edu,Yi Xu, Yan Yan,
Tianbao Yang
We develop a novel homotopy smoothing (HOPS) algorithm for non-smooth
convex optimization where the non-smooth term has a max-structure. The best
known iteration complexity of first-order method for this problem is
O(1/epsilon). We show that the proposed
HOPS achieves a lower iteration complexity of O (1/epsilon^(1-theta)) with theta
in (0, 1] capturing the local sharpness of the objective function around the
optimal solutions. The HOPS algorithm uses Nesterov’s smoothing method in
stages and gradually decreases the smoothing parameter until it yields a
sufficiently good approximation of the original function.
TC17