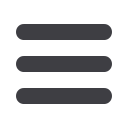

INFORMS Nashville – 2016
429
WC06
102A-MCC
Big Data 2
Sponsored: Data Mining
Sponsored Session
Chair: Milton Soto-Ferrari, Western Michigan University, 4601 Campus
Drive, Parkview Campus Building I, Kalamazoo, MI, 49008-5336,
United States,
miltonrene.sotoferrari@wmich.edu1 - Disease Detection Analytics: Comparing And Contrasting The
Performance Of Popular Predictive Models For Breast Cancer
And Diabetes Data Sets
Subhashish Samaddar, Professor, Business Analytics and
Operations, Georgia State University, Managerial Science,
P.O. Box 4014, Atlanta, GA, 30302-4014, United States, s-
samaddar@gsu.edu, Somnath Mukhopadhyay
Disease detection based on clinical data of the patient can save health care costs.
Consequently, data mining in disease detection is fast gaining popularity. Our
study applies popular predictive modeling algorithms such as random forest,
linear programming classifiers, and, neural network to two examples data sets of
clinical data: Breast cancer and Diabetes. The former data set has been bench
marked in the literature - so comparison with prior results was possible. The data
set on Diabetes can be used as new benchmark for future research. The article
reports compares and contrasts results from each method.
2 - Characterization Of Breast Cancer Patients Receiving
Unexpected Treatments
Milton Soto-Ferrari, Western Michigan University, 4601 Campus
Drive, Parkview Campus Building I, Kalamazoo, MI, 49008-5336,
United States,
miltonrene.sotoferrari@wmich.edu,Diana Prieto
In 2016, approximately 40,450 women in the US are expected to die from breast
cancer. Medical treatments are mainly driven by clinical factors including cancer
staging, tumor size, histology, and age. This research aims to propose a systematic
methodology to identify the clinical and non-clinical features that influence the
receipt of an unexpected treatment in breast cancer patients. We extend the factor
exploration and characterization of patients through a Bayesian Network
breakdown methodology that allows the analysis of conditional probabilities to
relate patient features with treatment receipt. We use registers of the SEER
program from Detroit area considering the period 2007-2012.
WC07
102B-MCC
Process Modeling
Sponsored: Data Mining
Sponsored Session
Chair: Najibe Sadatijafarkalaei, Wayne State University, 4815 Fourth
Street, Detroit, MI, 48202, United States,
fv0017@wayne.edu1 - An Efficient Nonparametric Fault Variable Identification Method
Mehmet Turkoz, Rutgers University, 16 Rachel Terrace, Piscataway,
NJ, 08854, United States,
turkoz@scarletmail.rutgers.eduIn a process, identifying which variables cause an out-of-control signal is a
challenging issue for quality problems. If the distribution of the process is
unknown, existing parametric methods are not suitable for identification of
changed variables. In this paper, we propose a new nonparametric method to
identify the fault variables and demonstrate its performance through various
simulation studies.
2 - A Hybrid Genetic Algorithm With Tabu List For Generating a
Stochastic Process Tree Model Based on Event Logs
Jin Young Choi, Ajou University, Worldcup-ro 206, Yeongtong-gu,
Suwon, 16499, Korea, Republic of,
choijy@ajou.ac.kr,
Woo-Min Joo, Do Gyun Kim
We present an efficient hybrid algorithm integrating genetic algorithm and tabu
search for generating a stochastic process tree model using event logs. It is
examined for its performance by considering some example event logs in
literature, evaluating four fitness measure such as simplicity, precision, replay,
and generalization.
3 - Observational Data Driven Modeling And Optimization Of
Manufacturing Processes
Najibe Sadatijafarkalaei, PhD Student, Wayne State University,
4815 Fourth Street, Detroit, MI, 48202, United States,
fv0017@wayne.edu,Ratna Babu Chinnam
The main objective of this study is to rely on observational data to achieve robust
parameter design of manufacturing processes. Controlled experiments can be
challenging in production environments and this paves for an effective alternative
approach to attain robust process parameter conditions. The proposed framework
relies on an integrated feature selection, response surface modeling, and
optimization methodology. We also report illustrative results from a tire
compound production process.
WC08
103A-MCC
Technology Mgt
Contributed Session
Chair: Mahmut Sonmez, Senior Lecturer in Management Science &
Statistics, University of Texas at San Antonio, College of Business, San
Antonio, TX, 78249-0631, United States,
maho.sonmez@utsa.edu1 - Wearable Technology In Fitness – Fitbit
Hongwei Du, Professor, California State University-East Bay,
25800 Carlos Bee Boulevard, Hayward, CA, 94542, United States,
hongwei.du@csueastbay.eduOne trending of wearable technology is Fitness Devices. This paper focuses on
wearable technology for fitness tracking and the FitBit Company. It identifies and
presents wearable technology behind the FitBit products, the pros and cons of the
FitBit, and the role that FitBit plays in the Internet of Things. Last, the future of
the FitBit Company and product is discussed.
2 - Dominant Design, Sequential Product Categories, And
Product Innovation
Hyunwoo Park, Postdoctoral Fellow, Georgia Institute of
Technology, 85 5th St NW, Atlanta, GA, 30332, United States,
hwpark@gatech.edu, Rahul C Basole
We study the impact of dominant design in sequential product categories on
product innovation using a dyadic perspective in the context of mobile phone
industry. Our results indicate that dominant design accelerates incremental
product innovation and causes temporary adverse shift in product category focus.
3 - Technological Innovation, International Patenting And National
Economic Development: A Multinational Multi-year Study
Kelvin Wayne Willoughby, Professor, Innovation and Intellectual
Property, Skolkovo Institute of Science and Technology, Skolkovo
Innovation Center, 3 Nobel Street, Moscow, 143026, Russian
Federation,
kelvin@skoltech.ru, Alexander Vidiborskiy
This paper reports the results of a study of the inbound and outbound patenting
activity of 78 countries for which reliable data were obtained over the 14 years
from 2000 to 2013. Several new indicators of the international patenting
proclivities of inventors were utilized in multiple phases of statistical analysis and
data vizualization to investigate the relationship between domestic inventive
activity, international patenting profiles and changes in the relative per capita
wealth levels of countries. The results suggest that the economic benefits
countries may gain from investing in technological innovation may be enhanced
by emphasizing the international patenting of domestic inventions.
4 - The D-day, V-day, And Bleak Days Of A Disruptive Technology:
A New Model For Ex-ante Evaluation Of The Timing Of
Technology Disruption
Chialin Chen, Professor, National Taiwan University, Taipei, 10617,
Taiwan,
cchen026@ntu.edu.tw, Jun Zhang, Ruey-Shan Guo
We conduct theoretical and empirical analyses to evaluate the timing of
technology disruption. We conceptualize the ease and network factors as key
determinants of performance improvement for a disruptive technology. A
dynamic consumer model is developed to identify two critical times, termed D-
Day and V-Day, of technology disruption. We also show that there may exist some
“bleak days” during which a firm would discontinue a “promising” technology
that will eventually disrupt. Empirical tests are conducted with data of hard disk
drives, semiconductor technologies, and CPU performance for mobile devices to
verify key model assumptions and to show how to estimate the ease and network
factors.
WC08