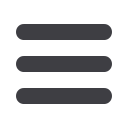

INFORMS Nashville – 2016
427
4 - Tractable Approximations Of Distributionally Robust Chance
Constraints In Radiation Therapy
Azin Khabazian, Research Assistance, University of Houston,
5465 Braesvalley Dr. Apt 566, Houston, TX, 77096, United States,
akhabazian@uh.edu, Maryam Zaghian, Gino J. Lim
Quadratic approximations of the distributionally robust chance constraints are
developed for treatment planning to guarantee the probabilistic constraint when
only partial information of the random dose contribution is known. Robust
chance constraints can be conservatively approximated by second-order cone
programming. In this study, we explore the condition in which the constraints
depend quadratically on the random parameter, and develop more precise
approximations for robust chance constraints. We evaluate these approximations
in the context of a radiation therapy treatment planning problem and numerically
demonstrate its superiority over the affine assumption of the constraints.
5 - Hospitalist’s Service Mix And Impacts On Length Of Stay
Masoud Kamalahmadi, Doctoral Student, Indiana University,
1309 E Tenth St, Bloomington, IN, 47405, United States,
maskamal@iu.edu, Kurt Bretthauer, Alex Mills, Jonathan Helm
Hospitalists are physicians that specialize in caring for hospital inpatients,
replacing a primary care physician who may only make rounds once per day and
thereby reducing delays. Given a limited number of hospitalists in a hospital, we
seek to determine their optimal service mix (workload and patient types).
Wednesday, 12:45PM - 2:15PM
WC01
101A-MCC
Data Mining in Aviation
Sponsored: Data Mining
Sponsored Session
Chair: Nima Safaei, Bombardier Aerospace., Unit 701, 23 Rean Dr.,
Toronto, ON, M2K 0A5, Canada,
nima.safaei@aero.bombardier.com1 - Multivariate Analysis Of Flight En Route Efficiency
Yulin Liu, University of California - Berkeley,
107 McLaughlin Hall, Berkeley, CA, 94709, United States,
liuyulin101@berkeley.edu,Michael O Ball, Mark M Hansen
We apply clustering and regression techniques to a large flight level trajectory
dataset that includes associated traffic management initiative (TMI) data. The
results quantify how TMIs, weather and other factors impact en route flight
efficiency. We further evaluate the variations of en route efficiency across city
pairs and over time.
2 - Data Clustering Using A Network Flow Problem To Study The
Aircraft Component Failure
Nima Safaei, Senior Specialist, Bombardier Aerospace., Unit 701,
23 Rean Dr., Toronto, ON, M2K 0A5, Canada,
nima.safaei@aero.bombardier.comAn integer programing model based on the network flow problem is proposed to
cluster the categorical variables and their attributes. The variables are related to
the age-related and -unrelated factors affecting the aircraft component failure.
The proposed model split the variables’ attributes into a number of clusters with
maximum transitive dependencies within each cluster
3 - Improving Airline Fuel Burn Predictions Using Super Learner
Lei Kang, Graduate Student Researcher, University of California-
Berkeley, 107 McLaughlin Hall, Berkeley, CA, 94720, United
States,
lkang119@gmail.com, Yulin Liu, Mark M Hansen
Accurate flight fuel burn predictions are crucial in the aviation industry. By
levering a large flight level fuel consumption dataset provided by a major US
airline, we propose to use integrated LASSO selection and Adaboost algorithm to
combine various machine learning algorithms into a super learner which can help
significantly reduce the fuel burn prediction error compared to our study airlines
flight planning system. The potential benefit of improved fuel burn predictions
will be quantified in terms of fuel savings.
WC02
101B-MCC
Data Mining Applications in Health Care
Sponsored: Data Mining
Sponsored Session
Chair: Eric Swenson, US Army, 643 Belmont Circle, State College, PA,
16803, United States,
eswen75@gmail.com1 - Identification Of Flu Hubs Using A Scale Free Network Of
Flu Distance
Hootan Kamran, PhD Candidate, University of Toronto,
Department of Mechanical and Industrial Engin, Room RS 311,
Toronto, ON, M5S 3G8, Canada,
hootan@mie.utoronto.ca, Michael
W Carter, Dionne Aleman, Kiearn Moore
Influenza is among the leading causes of death in the world. Rapid changes in
influenza virus make permanent immunity through vaccination an unviable
solution, and signify the importance of surveillance systems. Current systems
aggregate data based on predefined geopolitical divisions, and neglect historically
significant inter-regional time connections. We have devised a network structure
to model historic inter-regional flu distances in Ontario. We show that the
resulting network is not a random network and in fact, exhibits behaviours of a
scale-free network. The scale-free property helps us identify highly-connected
regions as flu hubs, which can be prioritized in containment policies.
2 - Children Segmentation Based On Risk Of Chronic Diseases
Nooshin Hamidian, Resaerch Assistant, University of Tennessee at
Knoxville, 301 Woodlawn Pike, Apt A5, Knoxville, TN, 37920,
United States,
nhamidia@vols.utk.edu, Jafar Namdar,
Rapinder Sawhney
Type 2 diabetes and obesity has increased among children during the last 3
decades. The main purpose of this study is to provide a framework that identifies
children who are at risk of diabetes and obesity. We explore a group of
demographic and behavioral characteristics, which increase the chance of these
diseases. Once the risk factors have been determined we develop a preventive
model. This model determines who is at risk of these diseases. Preventing chronic
diseases not only is beneficial for patients and their families, but also from the
hospital point of view, it can be a solution for cutting cost and increasing
hospitals’ revenue.
3 - Health Market Segmentation And Classification Of Total Joint
Replacement Surgery Patients
Eric Swenson, PhD Student, US Army, 643 Belmont Circle,
State College, PA, 16803, United States,
ers187@psu.eduEric Swenson, PhD Student, Pennsylvania State University,
University Park, PA, 16802, United States,
ers187@psu.edu,
Nathaniel Bastian, Harriet Black Nembhard
Understanding healthcare consumers’ behaviors and attitudes is critical
information when it comes to delivering patient-centered care. We apply a two-
stage methodology using supervised and unsupervised machine learning methods
to a 21 month sample of total joint replacement patient data. Patients cluster into
6 distinct market segments from which the cluster assignment is used as the
response variable in supervised learning to classify patients. The classification
model accurately predicts the cluster assignment for out-of-sample patients, while
offering insight into patient behaviors and attributes to help clinicians, health
marketers, and consumers enhance patient-centered care.
WC03
101C-MCC
Big Data III
Contributed Session
Chair: Mahamaya Mohanty, Research Scholar, IITDelhi, Shaheed Jeet
Singh Marg, New Delhi, 110016, India,
mahamayamohanty@gmail.com1 - Establishing A Big Data Analysis Framework For Computing Nash
Equilibrium With Vehicle Data
Lee Yu-Ching, National Tsing Hua University, Hsinchu, Taiwan,
yclee@ie.nthu.edu.tw, Ciou Si-Jheng, Huang Yi-Hao
This paper provides scalable framework to handle the data unable to be dealt with
by the general software. The aim is to generate a quantifiable value to represent
the customers’ willingness to buy products. Finally, the proposed method is
further validated by the real data of vehicles.
WC03