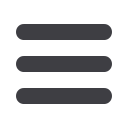

INFORMS Nashville – 2016
430
WC10
103C-MCC
Designing Energy and Water Supply Chains
for Prosperity
Sponsored: Energy, Natural Res & the Environment, Energy
Sponsored Session
Chair: Yao Zhao, 1 Washington Street, Newark, NJ, 07102,
United States,
yaozhao@andromeda.rutgers.edu1 - Distressed Selling By Farmers: Policy Recommendations
Shivam Gupta, University of Texas Dallas,
sxg104920@utdallas.edu,Milind Dawande, Ganesh Janakiraman,
Ashutosh Sarkar
In many developing countries, farmers sell a significant portion of their produce
at prices much lower than the guaranteed support price offered by the
government. We build a dynamic programming model to analyze this practice
and illustrate how it can serve as a useful decision making tool for policy
recommendations.
2 - Designing Hydro Supply Chains For Water, Food, Energy And
Flood Nexus
Kwon Gi Mun, Assistant Professor, Fairleigh Dickinson University,
Teaneck, NJ, United States,
kgmun@fdu.edu, Raza Ali Rafique,
Yao Zhao
The interconnected issues of water, food, energy and flood are among the most
formidable challenges faced by developing countries. We apply SCM principles to
water resource development and provide the end-to-end and dynamic
perspectives needed in the expansion of hydropower network, and also identify
the unique features and economies of hydropower systems and construct an
integrated location optimization model to capture the conflicts of these issues, to
explore the synergy among different sectors, and to maximize the overall benefit.
With the real-life situation of Pakistan, we provide solutions that outperform
common practices in all aspects of energy, irrigation, and flood control.
3 - Agricultural Support Prices In Developing Economies: Operational
Analysis And Its Use In Policy-making
Harish Guda, University of Texas Dallas,
hxg131530@utdallas.edu,
Tharanga Kumudini Rajapakshe, Milind Dawande,
Ganesh Janakiraman
The Guaranteed Support Price (GSP) scheme has been adopted in several
developing economies. Through this scheme, the government promises to procure
a crop from farmers at a guaranteed (and attractive) price announced ahead of
the selling season, and then distributes the procured amount to the
underprivileged population. The goal of this scheme is twofold: (a) as a supply-
side incentive, to ensure high output from farmers, and (b) as a demand-side
provisioning tool, to subsidize the consumption of the poor. In this talk, I present
our work on the operational decisions of the farmers and the government under
the GSP scheme, its impact on social welfare, and the use of our analysis to
policy-makers.
4 - Coordinating And Sharing Demand-side Energy Resources –
A Conceptual Design
Wei Qi, Lawrence Berkeley National Laboratory,
WQi@lbl.gov,Bo Shen, Hongcai Zhang, Zuo-Jun Max Shen
We present a coordination scheme for shared use of demand-side energy
resources (e.g. distributed generation, electric vehicles, etc.). Multiple users form
a sharing community within which trading electricity improves economic
efficiency. We develop a cost splitting scheme to ensure the participation of the
aggregator and the users.
WC11
104A-MCC
Decision Making Under Multistage Uncertainty
Sponsored: Optimization, Linear and Conic Optimization
Sponsored Session
Chair: Kartikey Sharma, Northwestern University, 2145 Sheridan Rd,
Evanston, IL, 60208, United States,
kartikeysharma2014@u.northwestern.eduCo-Chair: Omid Nohadani, Northwestern University,
Northwestern University, Evanston, IL, 60208, United States,
nohadani@northwestern.edu1 - Adjustable Robust Optimization Via Fourier-motzkin Elimination
Jianzhe Zhen, Tilburg University, Tilburg, Netherlands,
J.Zhen@tilburguniversity.edu,Melvyn Sim, Dick den Hertog
We demonstrate how adjustable robust optimization (ARO) problems with fixed
recourse can be casted as static robust optimization problems via Fourier-Motzkin
elimination (FME). Through the lens of FME, we characterize the structures of
the optimal decision rules for a broader class of ARO problems. A scheme based
on a blending of classical FME and a simple Linear Programming technique, that
can efficiently remove redundant constraints, is used to reformulate ARO
problems. This generic reformulation technique, contrasts with the classical
approximation scheme via linear decision rules, enables us to solve adjustable
optimization problems to optimality.
2 - Distributionally Robust Inventory Control When Demand Is
A Martingale
Linwei Xin, U of Illinois at Urbana-Champaign,
lxin@illinois.edu,
David Goldberg
Independence of random demands across different periods is typically assumed in
multi-period inventory models. In this talk, we consider a distributionally robust
model in which the sequence of demands must take the form of a martingale
with given mean and support. We explicitly compute the optimal policy and
value, and shed light on the interplay between the optimal policy and worst-case
martingale. We also compare to the analogous setting in which demand is
independent across periods, and identify interesting differences between these
two models.
3 - Robust Optimization With Decision Dependent Uncertainty Sets
Kartikey Sharma, Northwestern University,
kartikeysharma2014@u.northwestern.edu, Omid Nohadani
Robust optimization is increasingly used to solve multistage optimization
problems. In most such problems, the uncertainty sets are fixed. However in
many cases, these sets can be influenced by decision variables. We present a two-
stage robust optimization approach in which future uncertainty sets can be
affected by the decisions made in the first stage. We illustrate the advantages of
this model on a shortest path problem with uncertain arc lengths.
4 - Adaptive Probabilistic Satisficing Models
Zhi Chen, National University of Singapore, National University of
Singapore, Singapore, Singapore,
chenzhi@u.nus.edu, Melvyn Sim
In this paper, we study adaptive probabilistic satisficing models that can be used
for multi-stage decision making. We introduce the finite adaptability into
probabilistic satisficing models to overcome the difficulties of incorporating
recourse decisions as arbitrary functions of unfolded uncertain parameters. For
two-stage problems, we show that the complete adaptability is exact to the finite
adaptability, under a mild monotone condition. We propose an iterative scheme
for increasing the level of probabilistic satisficing. We discuss extensions of our
results for multi-stage problems. Our computational studies present that the
probabilistic satisficing solutions can be competitive.
WC12
104B-MCC
Recent Advances in Decision Diagrams
for Optimization
Sponsored: Optimization, Integer and Discrete Optimization
Sponsored Session
Chair: Andre Augusto Cire, University of Toronto Scarborough,
Toronto, ON, Canada,
acire@utsc.utoronto.ca1 - A Generic Approach To Solving Sequencing Problems With
Time-dependent Setup Times
Joris Kinable, Carnegie Mellon University, Pittsburgh, PA,
United States,
jkinable@cs.cmu.edu, Andre Augusto Cire,
Willem-Jan Van Hoeve
Tailoring dedicated solution approaches to solve scheduling and routing problems
is often complex and time consuming. This work presents a flexible framework
based on Constraint Programming, Mixed Integer Programming and Decision
Diagrams to solve hard scheduling problems including the Time-Dependent (TD)
TSP, TD-SOP and TD-TSP with Time Windows. The proposed method is
sufficiently generic to render it applicable to a variety of related sequencing
problems. Moreover, experiments indicate significant performance improvements
over pure MIP or CP approaches.
2 - Decision Diagram Bounds For Integer Programming Models
Christian Tjandraatmadja, Carnegie Mellon University, Pittsburgh,
PA, United States,
ctjandra@andrew.cmu.edu,Willem-Jan Van Hoeve
Decision diagrams are capable of generating strong bounds in practice for discrete
optimization problems when a certain structure is present. However, generalizing
decision diagram techniques to integer programming can be challenging due to
the lack of clear structure to exploit. We propose a framework to generate
decision diagram bounds that aims to overcome this issue. We start with a set of
base constraints that are well suited for decision diagrams and progressively
incorporate further constraints via strengthening and Lagrangian relaxation. We
discuss computational experiments on a set of binary optimization problems.
WC10