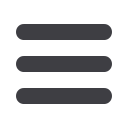

INFORMS Nashville – 2016
83
2 - Dea Computation For The Big Data – A Proactive Approach
Wen-Chih Chen, National Chiao Tung University, Hsinchu,
Taiwan,
wenchih@faculty.nctu.edu.tw, Yueh-Shan Chen
This talk presents a computation strategy to determine the DEA efficiencies of a
massive data set. The strategy proactively searches for the references of a data
point under evaluation by solving small-size linear programs (LPs). The size of
each individual LP solved is controlled within a guarantee upper bound. The
approach does not rely on the data density, and can improve the computational
performance significantly.
3 - Segmented Concave Least Squares: An Automatic Classification
Method With An Application To The Analysis Of The Room Rates
Of Hotels In Finland
Abolfazl Keshvari, Aalto University School of Business, Helsinki,
Finland,
abolfazl.keshvari@aalto.fiIn this paper, segmented concave least squares estimator is introduced. It
estimates a piecewise linear concave function, wherein the number of linear
segments (k) is pre-specified. Two extreme cases of this problem are ordinary least
squares (k=1) and concave least squares (k=n, the number of observations). The
estimator is used to analyze the room rates of hotels in Finland and to classify
them into three groups based on their pricing strategies.
SC41
207C-MCC
Finance Section Student Paper Competition
Sponsored: Financial Services
Sponsored Session
Chair: Rafael Mendoza, McCombs School of Business, 1, Austin, TX, 1,
United States,
rafael.mendoza-arriaga@mccombs.utexas.eduCo-Chair: Tim Siu-Tang Leung, Columbia University, New York, NY,
United States,
tl2497@columbia.edu1 - Robust Versus Sparse Portfolio Selection: Insights And
Alternatives
Yufei Yang, Singapore University of Technology and Design,
Singapore, Singapore,
eeyufei@gmail.comSelin Damla Ahipasaoglu, Jingnan Chen
In this talk, we will provide an in-depth discussion on the robustness and sparsity
trade-off in finding the mean-variance portfolio. We extend the classical mean-
variance framework by incorporating an ellipsoidal uncertainty set and fixed
transaction costs. We demonstrate that the optimal portfolio can be approximated
by a linear combination of three benchmark portfolios, and discuss how the
number of traded assets changes with respect to uncertainty level and transaction
cost.
2 - Portfolio Liquidity Estimation And Optimal Execution
Kai Yuan, Columbia Business School, Broadway, New York, NY,
10027, United States,
kyuan17@gsb.columbia.eduWe develop a tractable model to estimate portfolio liquidity costs through a multi-
dimensional generalization of the optimal execution model of Almgren and
Chriss. Our model allows for the trading of standardized liquid bundles of assets
(e.g., ETFs or indices). We show that in a “large universe” asymptotic limit, where
the correlations across a large number of assets arise from relatively few
underlying common factors, the liquidity cost of a portfolio is essentially driven
by its idiosyncratic risk. Moreover, the additional benefit of trading standardized
bundles is roughly equivalent to increasing the liquidity of individual assets.
3 - Spectral Portfolio Theory
Shomesh E Chaudhuri, Massachusetts Institute of Technology,
Cambridge, MA, 0, United States,
shomesh@mit.eduAndrew W Lo
Economic shocks can have diverse effects on financial market dynamics at
different time horizons, yet traditional portfolio management tools do not
distinguish between short- and long-term components in alpha, beta, and
covariance estimators. In this paper, we apply spectral analysis to quantify stock-
return dynamics across multiple time horizons. Using the Fourier transform, we
decompose asset-return variances, correlations, alphas, and betas into distinct
frequency components. These decompositions allow us to identify the relative
importance of specific time horizons in determining each of these quantities, as
well as to construct mean-variance-frequency optimal portfolios.
4 - Long Term Risk: A Martingale Approach
Likuan Qin, Northwestern University, Evanston, IL, 60208,
United States,
likuan.qin@gmail.comThis paper extends the long-term factorization of the stochastic discount factor
introduced and studied by Alvarez and Jermann (2005) in discrete time ergodic
environments and by Hansen and Scheinkman (2009) and Hansen (2012) in
Markovian environments to general semimartingale environments. The transitory
component discounts at the stochastic rate of return on the long bond and is
factorized into discounting at the long-term yield and a positive semimartingale
that extends the principal eigenfunction of Hansen and Scheinkman (2009) to the
semimartingale setting. The permanent component is a martingale that
accomplishes a change of probabilities to the long forward measure, the limit of T-
forward measures. The change of probabilities from the data generating to the
long forward measure absorbs the long-term risk-return trade-off and interprets
the latter as the long-term risk-neutral measure.
SC42
207D-MCC
Stochastic Systems in Finance
Sponsored: Financial Services
Sponsored Session
Chair: Alexandra Chronopoulou, Assistant Professor, University of
Illinois, Urbana-Champaign, 117 Transportation bldg. MC-238, 104 S.
Mathews Ave., Urbana, IL, 61801, United States,
achronop@illinois.edu1 - Statistical Inference For Long Memory Stochastic
Volatility Models
Alexandra Chronopoulou, University of Illinois,
Urbana-Champaign,
achronop@illinois.eduLong memory stochastic volatility (LMSV) models have been used to explain the
persistence of volatility in the market, while rough stochastic volatility (RSV)
models have been shown to reproduce statistical properties of low frequency
financial data. In these two classes of models, the volatility process is often
described by a fractional Ornstein-Uhlenbeck process with Hurst index H, where
H>1/2 for LMSV models and H<1/2 for RSV models. The goal of this talk is to
propose a general methodology for the estimation of the parameters of the above
models, the filtering of the volatility process, and the calibration of the Hurst
index, H, which will then be applied to the option pricing on the S&P 500 index.
2 - Optimal Randomized Unbiased Monte Carlo Simulation Of
Discounted Costs
Zhenyu Cui, Stevens Institute of Technology,
zcui6@stevens.eduIn this talk, we consider the problem of estimating the expected infinite-horizon
cost of running a stochastic system with stochastic fluctuations using Monte Carlo
simulation. We propose a randomized unbiased estimator based on truncating the
simulation horizon at an independent random time. The problem of determining
the optimal randomization distribution of the truncation random variable is
formulated as minimizing the “work-variance product” proposed by Glynn and
Whitt (1992). We solve this optimization problem explicitly and prove that it is
always optimal to use a “shifted” distribution. Numerical experiments illustrate
our findings. (This is joint work with Lingjiong Zhu).
3 - Monte Carlo Estimation Of Sensitivities From Analytic
Characteristic Functions
Runqi Hu, University of Illinois, Urbana-Champaign,
runqihu2@illinois.edu,Liming Feng
Sensitivity analysis is transformed into simulating a probability expectation
through the likelihood ratio method (LRM). In this paper, we apply Hilbert
transform inversion in evaluating a cdf on a uniform grid from its characteristic
function and provide explicit bound for estimation bias. In one dimension cases,
the bound allows one to determine the size and fineness of the grid and
numerical parameters for the inversion. For multidimensional cases, the
parameters can be determined by a procedure that will be proved to converge,
and work well practically. In the numerical experiments part, the method is
applied in estimating both European and Asian option deltas under CGMY model.
4 - Sensitivity Of The Eisenberg-Noe Network Model To The
Relative Liabilities
Mackenzie Wildman, University of California, Santa Barbara, CA,
United States,
mackenzie.wildman@gmail.comZachary Feinstein, Weijie Pang, Birgit Rudloff, Eric Schaanning,
Stephan Sturm
The Eisenberg-Noe algorithm gives a clearing payment vector for a system of
interconnected financial institutions in which some banks are unable to fulfill
their obligations to other banks in full. The network model takes as input a
relative liability matrix which gives the liabilities owed from each bank to its
counterparties. In practice, these liabilities are generally unknown and must be
estimated. We perform sensitivity analysis on this relative liability matrix and
obtain a worst-case scenario in terms of the payoff to a “society” node. We
illustrate our results on an EBA dataset of European banks.
SC42