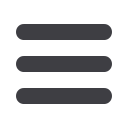

INFORMS Nashville – 2016
92
SC67
Mockingbird 3- Omni
Decision Analysis Approaches and Predictive
Modeling to Managing Uncertainty in Manufacturing
and Service Systems Design & Operations
Sponsored: Quality, Statistics and Reliability
Sponsored Session
Chair: Zhenyu Kong, Virginia Tech, 1145 Perry Street, Blacksburg, VA,
24060, United States,
zkong@vt.edu1 - Self-organizing Network For Variable Clustering And
Predictive Modeling
Hui Yang, Penn State University,
huy25@engr.psu.eduRapid advancement of sensing and information technology brings the big data,
which presents a gold mine of the 21st century to advance knowledge discovery.
However, big data also brings significant challenges for data-driven decision
making. In particular, it is common that a large number of variables (or
predictors, features) underlie the big data. Complex interdependence structures
among variables challenge the traditional framework of predictive modeling. This
paper presents a new methodology of self-organizing network for variable
clustering and predictive modeling.
2 - Forecasting Of Weather-driven Damage In A Distribution System
Of Electric Power
Zhiguo Li, IBM,
hardthinking@gmail.comElectric utilities spend a large amount of resources and budget on managing
unplanned outages, the majority of which are driven by weather. A major
ongoing effort is to improve their emergency preparedness process, in order to
reduce outage time, reduce repair costs, and improve customer satisfaction. This
paper proposes a method for forecasting the number of damages of different types
that will result from a weather event in a power distribution system. The
proposed method overcomes practical issues with sparsity of historical damage
and weather records, and its performance is evaluated on real utility data. This
work is the core of an approach called Outage Prediction and Response
Optimization.
3 - Prognostics Of Surgical Site Infections Using Dynamic
Health Data
Yan Jin, University of Washington - Seattle,
yanjin@uw.edu,
Shuai Huang
Surgical Site Infection (SSI) is a national priority in healthcare research. To
achieve better SSI risk prediction models, there have been emerging mobile
health (mHealth) apps that can closely monitor the patients and generate
continuous measurements of many wound-related variables and other evolving
clinical variables. Since existing predictive models of SSI have quite limited
capacity to utilize the evolving clinical data, we develop the corresponding
solution to improve these mHealth tools with decision-making capabilities for SSI
prediction. We derive efficient algorithms and demonstrate the advantage of our
new predictive model on a real-world dataset.
4 - Spatiotemporal Model With Dirichlet Process Mixing For
Nonnormal And Nonstationary Data
Jia Liu, Virginia Tech,
jliu@vt.eduIn real-life, sensor data often violate assumptions of normality and stationarity
required by many prevalent statistical methods. In order to acquire accurate
prediction and interpolation by sensor data, a nonparametric spatiotemporal
model is proposed, which takes non-normality and non-stationarity of data into
account. In this model, spatial correlation is captured by Dirichlet process mixture
model using particle filter. Moreover, temporal correlation is incorporated into
this model by using recurrent Dirichlet process. This model can be used in various
fields with data exhibiting non-normality and non-stationarity to achieve
accurate interpolation and prediction.
SC68
Mockingbird 4- Omni
Panel Discussion: Funding Opportunities
Sponsored: Quality, Statistics and Reliability
Sponsored Session
Moderator: Abhishek K Shrivastava, Florida State University,
Tallahassee, FL, United States,
ashrivastava@fsu.eduCo-Chair: Hui Wang, FSU, TBD, TBD, FL, 00000, United States,
hwang10@fsu.edu1 - Panel Discussion On Funding Opportunities
Abhishek Shrivastava, Florida State University, FAMU-FSU College
of Engineering, Tallahassee, FL, 32310, United States,
ashrivastava@fsu.eduIn this panel, program officers from NSF will discuss funding opportunities in
their programs. The panelists are Dr. Joanne Culbertson, Dr. David Mendonca, Dr.
Jon Leland and Dr. Alexandra Medina-Borja
2 - Panelist
Alexandra Medina-Borja, US National Science Foundation, 2507
Fowler St, Falls Church, VA, 22046, United States,
alexandra.medinaborja@upr.edu3 - Panelist
David Mendonca, NSF, Arlington, VA, 22230, United States,
mendonca@nsf.gov4 - Panelist
Joanne Culbertson, National Science Foundation, 4201 Wilson
Boulevard, Arlington, VA, 22230, United States,
jculbert@nsf.govSC70
Acoustic- Omni
Transportation, Freight III
Contributed Session
Chair: Carlos Alberto Gonzalez-Calderon, Rensselaer Polytechnic
Institute, 4 25th St, Apt 5, Troy, NY, 12180, United States,
gonzac8@rpi.edu1 - A Multi-commodity Intermodal Traffic Assignment Between
Rail And Truck
Lokesh Kumar Kalahasthi, Rensselaer Polytechnic Institute,
22 College Ave, Troy, NY, 12180, United States,
kalahl@rpi.eduTrilce Marie Encarnacion, Jose Holguin-Veras, John E Mitchell
The goal of the paper is to obtain an optimization model that gives a freight traffic
assignment on a combined network of road and rail; that could be used to assess
the freight modal split including vehicle types and intermodal transfers. Authors
of this paper have conducted In-Depth-Interviews (IDI) with shippers, carriers
and receivers regarding the factors influencing their mode choice. The challenge is
to incorporate the findings from these IDIs into a mathematical model. Major
findings include commodity type, backhaul, shipment limit, transfer time,
reliability in transit time restrictions. The model also incorporates the variation in
the rail pricing based on origin and destination.
2 - Reliable Routing Of Multicommodity Road-rail Intermodal Freight
Under Uncertainty
M. Majbah Uddin, University of South Carolina, 300 Main Street,
Civil and Environmental Engineering, Columbia, SC, 29208,
United States,
muddin@cec.sc.edu, Nathan Huynh
A reliable routing model for multicommodity shipments on a road-rail intermodal
freight transport network, where network elements are subject to uncertainty, is
proposed. A stochastic mixed integer program is formulated which minimizes not
only operational costs but also penalty cost associated with unsatisfied demand.
This study provides a novel distribution-free approach to ensure probabilistic
guarantees on the resulting routing plan. Case study on a small network reveals
the key characteristics of the proposed model.
3 - Shipment Consolidation And Dispatching With Cross-docks
Sinem Tokcaer, Izmir University of Economics, Fevzi Cakmak Mh,
Sakarya Cd No:156, Izmir, 35330, Turkey,
sinem.tokcaer@ieu.edu.tr,Ahmet Camci, Ozgur Ozpeynirci
Freight forwarders dealing with long haul transportation establish their own
consolidation systems in order to reduce costs by economies of scale and efficient
use of owned or rented vehicles. Such consolidation systems usually include
cross-docking terminals to provide additional services and reduce the travelling
time of the vehicles. We propose a shipment consolidation and dispatching
problem with cross-docks, and develop a mathematical programming model. The
model suggests the consolidation and transportation plans. We propose lower and
upper bounds, develop a Variable Neighborhood Search algorithm, and test the
performances of develop methods on randomly generated instances.
4 - Freight Trip Generation (FTG), Freight Generation FG) And Service
Trip Attraction (STA) In New York City (NYC) And Capital Region
Carlos Alberto Gonzalez-Calderon, Rensselaer Polytechnic
Institute, 4 25th St, Apt. 5, Troy, NY, 12180, United States,
gonzac8@rpi.edu, Jose Holguin-Veras, Shama Campbell,
Lokesh Kumar Kalahasthi
This paper presents a thorough analyses and econometric models explaining the
Freight Trip Generation (FTG), Freight Generation (FG) and the Service Trips
Attraction (STA) in the New York City and Capital Region. The team conducted a
detailed survey including the number of deliveries (received), shipments (sent),
type of cargo, weight of shipment, industry sector, truck type, who transports the
cargo (vendor or receiver). This study serves as a tool for transportation planners
in understanding the freight patterns in urban areas.
SC67