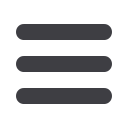

INFORMS Nashville – 2016
95
Keynote
Davidson Ballroom C-MCC
Analytic + IT + Deployment = Real World Impact
Keynote Session
Robin Lougee, IBM TJ Watson Research Center, Yorktown Heights, NY
10598,
rlougee@us.ibm.com1 - Analytic + IT + Deployment = Real World Impact
Ramayya Krishnan, Carnegie Mellon University, Pittsburgh, PA,
United States,
rk2x@cmu.eduThe Heinz College is home to two highly ranked graduate schools: 1) Information
Systems and Management and 2) Public Policy and Management, a deliberate
structure which exists only at Carnegie Mellon University (CMU). Founded by
noted Management Scientist W. W. Cooper to educate “men and women capable
of intelligent action”, the unique structure of the college gives its educational
programs a holistic focus on societal problem solving. This focus translates into
teaching cutting-edge information technologies and analytic methods and
providing students with multiple opportunities to apply them to solve real world
problems that matter. This focus also means an emphasis on structuring
unstructured problems and an education in the skills required to be effective at
that structuring and at decision making, and engendering change through
deployment. In this keynote, I will provide an overview of our award winning
analytics program and describe how we combine industry-funded research
centers and their partner ecosystems to provide students with multiple
opportunities to learn an array of analytic skills and problem-solving expertise in
order to be effective in the real world.
SC94
5th Avenue Lobby-MCC
Wiley/Provalis Research
Technology Tutorial: Wiley/Provalis
1 - Provalis Research will Showcase its Integrated Collection of Text
Analytics Software
Normand Peladeau, Provalis Research, Montreal, QC, Canada.
peladeau@provalisresearch.com2 - Wiley: Interested in Publishing for the Wiley Series in Operations
Research and Management Science?
James Cochran, University of Alabama, Tuscaloosa, AL,
jcochran@cba.ua.eduThe Wiley Series in Operations Research and Management Science is a broad
collection of books that meet the varied needs of researchers, practitioners, policy
makers, and students who use or need to improve their use of analytics. The
workshop will include presentations on the following: • The Mission of the Series
for the Betterment of the Community • The Proposal Process: Maximizing your
Success • What’s Next: The Writing, Review, and Publishing Process • Q&A In
addition to the presentation, you will be able to meet with Founding Series Editor
James J. Cochran and Wiley Editor Susanne Steitz-Filler to discuss any further
questions or potential book ideas you may have.
Sunday, 4:30PM - 6:00PM
SD01
101A-MCC
Interpretable Machine Learning in Social Science
Sponsored: Data Mining
Sponsored Session
Chair: Tong Wang, MIT, Cambridge, MA, United States,
tongwang@mit.edu1 - Interpretable Decision Sets: A Joint Framework For Description
And Prediction
Himabindu Lakkaraju, Stanford University,
himalv@cs.stanford.eduOne of the most important obstacles to deploying predictive models is the fact
that humans do not understand and trust them. In this talk, I will present
interpretable decision sets, a framework for building predictive models that are
highly accurate, yet also highly interpretable. We formalize decision set learning
via an objective function that simultaneously optimizes for accuracy, conciseness,
and meaningfulness of the rules. We prove that our objective is a non-monotone
submodular function, and efficiently optimize it with a 2/5 approximation
guarantee. Our experiments demonstrate that interpretable decision sets help
humans understand their data better than other interpretable models.
2 - Exploring Complex Systems Using Semi-parametric
Graphical Models
Mladen Kolar, University of Chicago,
mkolar@chicagobooth.eduExtracting knowledge and providing insights into the complex mechanisms
underlying noisy high-dimensional data sets is of utmost importance in many
scientific domains. Networks are an example of simple, yet powerful tools for
capturing relationships among entities over time. For example, in social media,
networks represent connections between different individuals and the type of
interaction that two individuals have. Unfortunately the relationships between
entities are not always observable and need to be inferred from nodal
measurements. I will present a line of work that deals with the estimation and
inference in high-dimensional semi-parametric elliptical copula models.
3 - Causal Rule Set For Subgroup Identification
Tong Wang, University of Iowa,
tongwang@mit.eduWe propose an interpretable classifier for causal analysis, Causal Rule Set (CRS),
that discriminates between two types of subgroups, one that benefits from the
treatment (Effective Class), and one does not (Ineffective class). CRS uses a set of
interpretable rules to present and characterize an Effective class. We present a
Bayesian framework with priors that favor simple models, and a Bayesian logistic
regression that models the relation between outcomes and a set of observed
(attributes) and inferred objects (subgroup membership). The simulation studies
and experiments on real data sets show that distributing treatment according to a
CRS model enhances the average treatment effect.
SD02
101B-MCC
Data Analytics and Modeling for Medical Prognosis
and Decision Making
Sponsored: Data Mining
Sponsored Session
Chair: Shouyi Wang, University of Texas-Arlington, Arlington, TX,
United States,
shouyiw@uta.edu1 - Disgnosis Of Posttraumatic Stress Disorder Using Functional
Near Infrared Spectroscopy (fNIRS) signals And Data
Mining Techniques
Rahil Hosseini, University of Texas at Arlington,
rahilsadat.hosseini@mavs.uta.eduIn this paper we extract various feature groups from FNIRS records that are from
the experiment about digits memorizing and recalling; it includes three phases in
each trial; encode, maintain and recall; we show the discovered patterns between
two classes for some selected features. Specifically the results show that the last
phase which is when the subject tries to recall the digits, is the most significant
part and with extracted features from Statistics, Autocorrelation and SVDNorm; it
is enough for highly accurate
classification.Wediscuss a new proposed feature
derived from SVD (Singular Value Decomposition) of raw data in channels. It
demonstrated promising results in classification. Third contribution is comparison
of feature selection methods to reduce the dimension of the feature matrix. We
compare the performance through number of selection and sensitivity and
specificity and their average. The proposed method includes Mutual Information
(MI) guided sparse models that outperform the existing features selection
techniques. The existing models are ‘’minimum Redundancy and Maximum
Relevancy’’ (mRMR), and ‘’Sparse Group Lasso’’ (SGL). We propose ‘’Mutual
Information and Least Absolute Selection and Shrinkage Operator’’ (MILASSO)
‘’Mutual Information Sparse Group Lasso’’ (MISGL). All these techniques are
applied to classify PTSD veterans and healthy controls using ‘’Support Vector
Machines’’ (SVM). Last contribution is finding the Region of Interest (ROI), we
conclude that two specific areas on brain are the most significant ones which are
directly related to memorizing
2 - Pattern Classication And Analysis Of Memory Processing In
Depression Using Eeg Signals
Kin Ming Puk, University of Texas at Arlington,
bookbook0089@gmail.comAn automatic, EEG-based approach of diagnosing depression with regard to its
effect on memory is presented. EEG signals are extracted from 15 depressed
subjects and 12 normal subjects during experimental tasks of reorder and
rehearsal. After pre-processing noisy EEG signals, seven groups of mathematical
features are extracted and classification with SVM is conducted under a five-fold
cross-validation, with accuracy of up to 70% - 100%. The contribution of this
paper lies in illustrating the usefulness of the classification framework in
facilitating the analysis and visualization of the difference of EEG signals between
depressed and control subjects in memory processing.
SD02
Abstract Available Online.