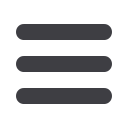

INFORMS Nashville – 2016
96
3 - Discriminating Parkinson’s Disease (pd) Using Functional
Connectivity And Brain Network Analysis
Shouyi Wang, University of Texas at Arlington,
shouyiw@uta.eduIn this study, we explored the use of functional connectivity patterns in fMRI data
to classify subjects on the basis of Parkinson’s disease. We explore various brain
networks and features. We partition our fMRI data in 5 filtered frequency ranges.
We use a proximal support vector machine paired with a minimum-redundancy
and maximum-relevance feature selection method on each frequency range. We
use a majority voting ensemble classification method on the results of the
proximal support vector machine classification results. Our results indicate that
the ensemble method is effective compared to a single broad frequency range,
and that Bonferroni correction may enhance classification results.
SD03
101C-MCC
Doing Good with Good OR II
Invited: Doing Good with Good OR
Invited Session
Chair: Chase Rainwater, University of Arkansas, 4207 Bell Engineering
Center, Fayetteville, AR, 72701, United States,
cer@uark.edu1- Optimizing Breast Cancer Diagnostic Decisions to
Reduce Overdiagnosis
Sait Tunc, Department of Industrial and Systems Engineering,
University of Wisconsin-Madison, Madison, WI 53706,
stunc@wisc.edu, Oguzhan Alagoz, Elizabeth S. Burnside
Although the early diagnosis of breast cancer through screening mammography
saves thousands of lives every year, overdiagnosis of breast cancer may cause
harm without benefit. We propose a comprehensive large-scale optimization
model to address overdiagnosis by making better-informed diagnostic decisions
and provide the exact optimal policies that potentially save up to 9% of the
biopsied population from overdiagnosis..
2 - A Decision Support System for the Management of Aid-In-Kind
Donations for Turkish Red Crescent
Semih Boz, Department of Industrial Engineering, Bilkent
University, Ankara, Turkey, Semih Kaldirim, Bilge Kaycioglu,
Buse Eylul Oruc, Eren Ozbay, Mirel Yavuz, Sinan Derindere,
Ali Erkan, Pinar Ozkurt
Turkish Red Crescent is the main body for collection and distribution of donations
in Turkey, and this project aims to improve their donation collection and
distribution processes by proposing a decision support system with numerous
subsystems; e.g. implementing new process flows and their accompanying
decisions. The proposed system is approved by TRC and it is being integrated to
their systems.
SD04
101D-MCC
Optimizing Urban Infrastructure Resilience Under
Climate Change
Sponsored: Energy, Natural Res & the Environment I Environment &
Sustainability
Sponsored Session
Chair: Mohammad Ramshani, University of Tennessee,
524 John D. Tickle Building, Knoxville, TN, 37996, United States,
mramshan@vols.utk.edu1 - Optimizing Green Roof Integrated Photovoltaics Placement Under
Climate Change
Mohammad Ramshani, University of Tennessee,
mramshan@vols.utk.edu, Xueping Li, Anahita Khojandi,
Olufemi A Omitaomu
We develop a two-stage stochastic model to optimally place green roofs and/or
Photovoltaic panels under climate change uncertainty, with the aim of improving
urban system resilience. Different climate forecasts from different climate models
are taken into account as different scenarios. The interaction between green roofs
and Photovoltaic panels in terms of efficiency is considered. An efficient L-shaped
algorithm is developed. Computational studies along with sensitivity analysis are
conducted.
2 - Optimal Planning Of Green Infrastructure Placement Under
Precipitation Uncertainty
Masoud Barah, University of Tennessee,
mbarah@vols.utk.edu,
Anahita Khojandi, Xueping Li, Jon Hathaway
Green Infrastructures (GIs) are low cost, low regret strategies that can
dramatically contribute to stormwater management. We develop a multi-objective
stochastic programming model to determine the optimal placement of GIs across a
set of candidate locations in a watershed to minimize the excess runoff under
short-term and medium-term precipitation uncertainties. We calibrate the model
using precipitation projections and stormwater system’s hydrologic responses to
them. We obtain the optimal GI placement for a watershed and perform
sensitivity and robustness analyses to provide insights.
3 - Optimal Placement Of Green Infrastructure Under Uncertainty
Anahita Khojandi, University of Tennessee, 603 W Main St.,
Apt 801, Knoxville, TN, 37902, United States,
anahitakhojandi@gmail.com, Mohit Shukla, Xueping Li,
Mohammad Ramshani
Despite the environmental and societal benefits of Green Infrastructure (GI), they
are mostly planned and established in response to an existing problem rather than
being actively incorporated into the early stages of urban planning. In this paper,
we present a stochastic model that would allow urban planners to incorporate
uncertainties in population and climate predictions, land use and budgetary
constraints and the ‘connectivity’ between GIs into the decision making process of
GI placement on a county or city scale land area. The proposed approach is tested
on data from a real county to evaluate its utility.
SD05
101E-MCC
ENRE Award Session
Sponsored: Energy, Natural Res & the Environment, Energy I
Electricity
Sponsored Session
Chair: Andy Sun, Georgia Institute of Technology, Atlanta, GA, 30332,
United States,
andy.sun@isye.gatech.eduSD06
102A-MCC
INFORMS 2016 Data Mining Best Student
Paper Awards II
Sponsored: Data Mining
Sponsored Session
Chair: Mustafa Gokce Baydogan, Bogazici University, Istanbul, Turkey,
baydoganmustafa@gmail.comSD07
102B-MCC
Joint Session DM/AI: Predictive Analytics in
Data Science
Sponsored: Data Mining
Sponsored Session
Chair: Xi Wang, University of Iowa, S210 John Pappajohn Business
Building, Iowa City, IA, 52242-1000,
xi-wang-1@uiowa.edu1 - Link Prediction In Multi-relational Networks For Online
Health Communities
Xi Wang, The University of Iowa,
xi-wang-1@uiowa.eduKang Zhao
Online Health Communities (OHCs) are a popular resource for those with health
problems to exchange information and support. Users often interact via multiple
communication channels, such as online discussions, blogs, and private messages.
Connections among users via different channels form a multi-relational social
network. Using data from a smoking cessation network, this study aims to predict
links between users in one sub-network based on information from other sub-
networks. Our findings regarding tie formation will inform the development and
ongoing management of online health communities.
SD03