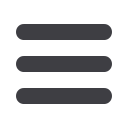

INFORMS Nashville – 2016
99
4 - Sparse Convex Regression
Nishanth Mundru, Massachusetts Institute of Technology,
Cambridge, MA, 02139, United States,
nmundru@mit.edu,
Dimitris Bertsimas
Given data (xi, yi) (d+1), i=1,..,n, the problem of convex regression finds a
convex function f: d that minimizes the error
∑
i (yi - f(xi) )2. We propose a
cutting plane algorithm that scales to (n,d) = (10^4,10) in minutes and (n,d) =
(10^5,10^2) in hours. Sparse convex regression finds the best k out of d features,
and solves for the optimal convex function on that subset. Using Mixed integer
optimization methods and first order convex optimization based heuristics, we
extend our algorithm to model sparsity, and solve the problem to provable
optimality for (n,d,k) = (10^4,10^2,10) in minutes.
SD16
105A-MCC
Buffered Probability of Exceedance and Applications
Sponsored: Optimization, Optimization Under Uncertainty
Sponsored Session
Chair: Matthew Norton, University of Florida, 355 Tigert Hall,
Gainesville, 32611, United States,
mdnorton@ufl.edu1 - Buffered Probability Of Exceedance And Applications To
Machine Learning
Matthew Norton, University of Florida,
mdnorton@ufl.eduWe present a new characterization of uncertainty, called Buffered Probability of
Exceedance (bPOE), specifically addressing its application to machine learning.
Using ideas from Robust Optimization, we show that Robust bPOE minimization
provides a highly flexible framework for binary classification which encompasses
support vector machines (SVM’s), including SVM variants which utilize Robust
Optimization and various convex/non-convex regularization schemes. While also
providing a more concrete interpretation for various SVM formulations, the
proposed framework reveals a fundamental connection between many
regularization schemes and Robust Optimization principals.
2 - Buffered Probability Of Exceedance: Methodology
And Applications
Stan Uryasev, University of Florida,
uryasev@ufl.eduThis paper investigates a new probabilistic characteristic called buffered
probability of exceedance (bPOE). With bPOE, it is possible to count outcomes
similar to a threshold value, rather than only outcomes exceeding the threshold.
To be more precise, bPOE counts tail outcomes averaging to some specific
threshold value. bPOE can be considered as an important supplement to POE. We
will discuss the Cash Matching problem for a Portfolio of Bonds. We minimize
bPOE that assets exceed liabilities.
3 - Smoothed Buffered Probability Of Exceedance: A New Class Of
Probability-like Uncertainty Measures
Alexander Mafusalov, University of Florida,
mafusalov@ufl.eduBuffered probability of exceedance (bPOE) is a risk-averse alternative to
probability of exceedance and cumulative distribution function. Minimization of
bPOE is reduced to convex programming or even LP for a wide class of problems.
However, being a non-smooth function, bPOE is not always well suited for
gradient optimization. In addition, bPOE reverses the curve of CVaR values, while
another family of risk measures might be preferable in a given application. This
paper introduces a new class of smooth probability-like uncertainty measures,
which are based on bPOE. Dual representations and other mathematical
properties, along with advantages in optimization, are studied.
4 - Approximation Of A Distribution With A Finite Mixture Of Some
Other Distributions Using Cvar Norm
Giorgi Pertaia, University of Florida, Gainesville, FL, United States,
georgepertaia@gmail.comCVaR norm is applied to approximate a distribution with a finite mixture of some
other distributions. A finite mixture is a weighted sum of simple distributions
(such as normal or triangular). Despite the simplicity of underlying distributions,
the finite mixture can model a wide variety of distributions with heavy tails.
Classical approach of fitting the mixture relies on Maximum Likelihood
estimation, which, in general, leads to a nonconvex optimization problem (with
many local minima where the optimization algorithms might get trapped). In
contrast, procedures using CVaR norm minimization lead to convex
programming, which can be reduced to linear programming problems.
SD17
105B-MCC
Polyhedral Methods in Integer Programming
under Uncertainty
Sponsored: Optimization, Optimization Under Uncertainty
Sponsored Session
Chair: Weijun Xie, Georgia Institute of Technology, 225 North Ave,
Atlanta, GA, 30332, United States,
wxie33@gatech.edu1 - Decomposition Methods For Solving Distributionally Robust
Two-stage Stochastic Integer Programs
Sanjay Mehrotra, Northwestern University,
mehrotra@northwestern.edu, Manish Bansal, Kuo-Ling Huang
We present a cutting surface L-shaped method for solving 2-stage distributionally
robust mixed integer programs. We show the finite convergence of the algorithm
under suitable conditions. Wasserstein and moment based uncertainty sets are
considered. Numerical results will be presented that demonstrate the performance
of our approach and illustrate its ability to perform distributional sensitivity
analysis.
2 - An Integer Programming Approach For Two-sided
Chance-constrained Programs
Xiao Liu, The Ohio State University,
liu.2738@osu.eduSimge Kucukyavuz, Fatma Kilinc-Karzan
We study two-sided chance-constrained programs with a finite probability space.
We reformulate this class of problems as a mixed-integer program. We study the
key substructure of the reformulation and propose valid inequalities that define
the convex hull of this substructure. Finally, we propose polynomial optimization
and separation algorithms for the optimization problem over this substructure.
3 - A Polyhedral Approach To Online Bipartite Matching
Alfredo Torrico, Georgia Institute of Technology, Atlanta, GA,
United States,
atorrico3@gatech.edu, Alejandro Toriello,
Shabbir Ahmed
We study the i.i.d. online bipartite matching problem, a dynamic version of the
classical model where one side of the bipartition is fixed and known in advance,
while nodes from the other side appear one at a time as i.i.d. realizations of a
uniform distribution, and must immediately be matched or discarded. We
consider various relaxations of the polyhedral set of achievable matching
probabilities, introduce valid inequalities, and discuss when they are facet-
defining. Several of these relaxations correspond to ranking policies and their
time-dependent generalizations.
4 - On Risk Averse Submodular Minimization
Weijun Xie, Georgia Institute of Technology, Atlanta, GA,
United States,
wxie33@gatech.edu, Shabbir Ahmed
This paper studies a risk averse submodular minimization problem (RASMP)
under a knapsack constraint. Many problems can be reformulated as RASMP
(e.g., chance constrained problems, mean-risk models, optimization with
conditional-value-at-risk, etc). Approximation algorithms are proposed for
RASMP by rounding an optimal solution to a suitable convex relaxation. We also
propose new valid inequalities by partitioning binary variables into groups, and
their separation.
SD18
106A-MCC
Marketing VI
Contributed Session
Chair: Gary Chao, Kutztown University, 888 Kingston Ln, Breinigsville,
PA, 18031, United States,
chao@kutztown.edu1 - Product Life Cycle Among Different Generations
Gary Chao, Kutztown University, PO Box 730, Department of
Business Administration, Kutztown, PA, 19530, United States,
chao@kutztown.edu,Maxwell Hsu
The launch of a new product needs a larger commitment in time, money, and
managerial resources. The new product introduction requires careful planning to
ensure the expected market success of a new product. We would like to study
how frequently and when an automaker introduced the new models, how an
automaker designed their product line, what factors influence the diffusion. We
attempt to fit longitudinal data across brands to the Bass diffusion model and
examine the generation change within the same model from different
automakers in order to identify the factors influencing car sales.
SD18